Towards Malay Abbreviation Disambiguation: Corpus and Unsupervised Model.
NLPCC (2)(2023)
摘要
Abbreviation disambiguation constitutes a highly crucial natural language processing task in all languages, including Malay. Its objective involves the identification of the most suitable definition, from a candidate set of definitions, that corresponds to a given abbreviation based on contextual information. The current state of research on Malay abbreviation disambiguation is hindered by the absence of an extensive database of abbreviations, thus posing difficulties in supporting model training. Simultaneously, the challenge lies in developing a Malay abbreviation disambiguation model that can achieve a satisfactory level of restoration performance even in the absence of annotated samples, thereby facilitating enhanced comprehension of literature among individuals. Consequently, the lack of a large-scale abbreviation database and the construction of an effective disambiguation model without annotated samples present ongoing challenges in the field of Malay abbreviation disambiguation. To address the above issues, we construct a dataset of Malay abbreviations and propose an unsupervised method based on a pre-trained model to solve the problem of abbreviation disambiguation. This method sorts out the perplexity score of each definition according to the definition corresponding to the abbreviation in the same sentence. Subsequently, the definition associated with the lowest perplexity score is selected as the most suitable choice. On the constructed Malay dataset, our method exhibits a mere 3% decrease in accuracy compared to the current state-of-the-art (SOTA) supervised approach, thereby showcasing a remarkable advantage within the domain of unsupervised methods. Notably, in the SDU@AAAI-22-Shared Task 2: Acronym Disambiguation, our experimental results demonstrate effectiveness across all four test sets. Particularly, the performance is exceptionally notable in the context of legal English, achieving an accuracy rate of 77.28%. The source code and dataset of this paper is publicly available at https://github.com/bhysss/TMAD-CUM .
更多查看译文
关键词
malay abbreviation disambiguation,corpus
AI 理解论文
溯源树
样例
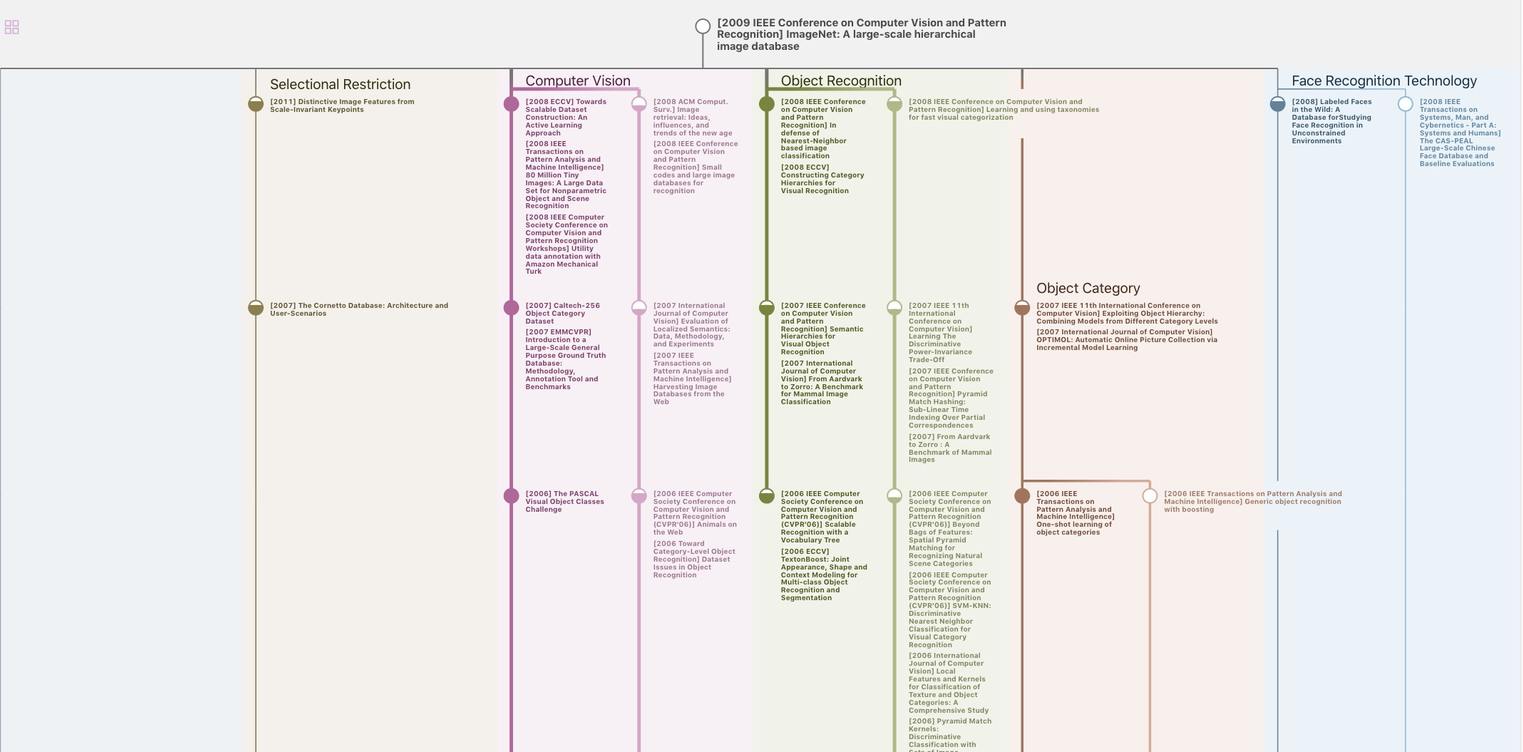
生成溯源树,研究论文发展脉络
Chat Paper
正在生成论文摘要