Hierarchical Image Fine-Grained classification via Hierarchical Feature Mining and Filtering.
ICDLT(2023)
摘要
Transformer network has been widely applied in the field of computer vision. Thanks to the application of self-attention mechanism, Transformer can extract and pay attention to features at each position in the image, capturing details more accurately. In this paper, in order to address the issue of obtaining different levels of classification results based on different semantic contexts, we propose a hierarchical image fine-grained classification network based on Swin Transformer. Specifically, multiple classification results of different classification granularity levels can be obtained for the same image. We also suggest a parallel multi-level feature mining module, establish a progressive training model, and use a patch generator to build training samples of different granularity and stages, so as to extract features of different classification granularity levels. We design a hierarchical feature fusion module to promote the classification results of the fine-grained branch on the coarse-grained branch. Furthermore, we present a weakly supervised feature selector to enhance the feature representation ability and improve the classification accuracy, which helps to select effective feature points from different stages of feature maps. Finally, we evaluate its performance on three benchmark datasets, and find that the proposed method achieves good performance in terms of weighted average accuracy.
更多查看译文
AI 理解论文
溯源树
样例
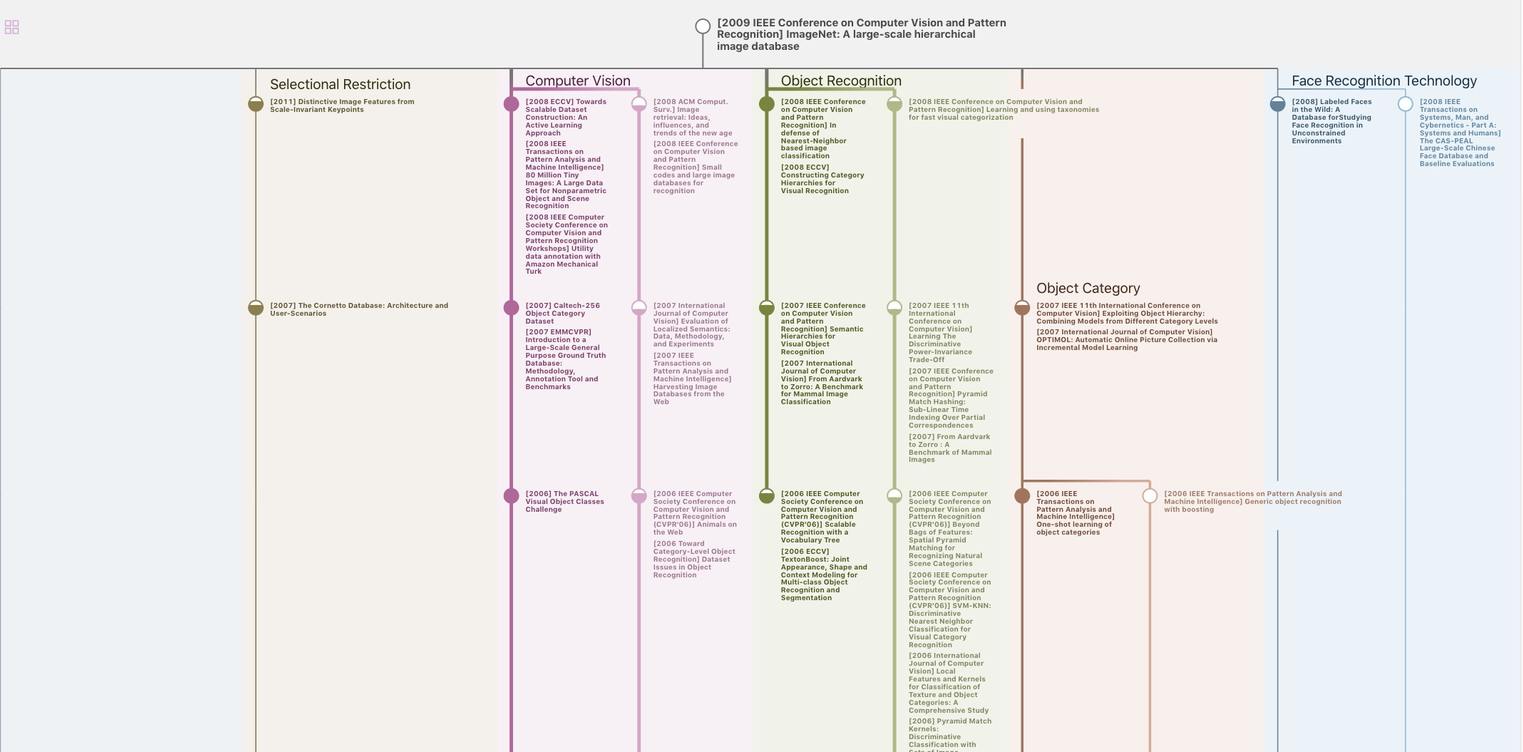
生成溯源树,研究论文发展脉络
Chat Paper
正在生成论文摘要