Development of meta-prompts for Large Language Models to screen titles and abstracts for diagnostic test accuracy reviews
medRxiv (Cold Spring Harbor Laboratory)(2023)
摘要
Systematic reviews (SRs) are a critical component of evidence-based medicine, but the process of screening titles and abstracts is time-consuming. This study aimed to develop and externally validate a method using large language models to classify abstracts for diagnostic test accuracy (DTA) systematic reviews, thereby reducing the human workload. We used a previously collected dataset for developing DTA abstract classifiers and applied prompt engineering. We developed an optimized meta-prompt for Generative Pre-trained Transformer (GPT)-3.5-turbo and GPT-4 to classify abstracts. In the external validation dataset 1, the prompt with GPT-3.5 turbo showed a sensitivity of 0.988, and a specificity of 0.298. GPT-4 showed a sensitivity of 0.982, and a specificity of 0.677. In the external validation dataset 2, GPT-3.5 turbo showed a sensitivity of 0.919, and a specificity of 0.434. GPT-4 showed a sensitivity of 0.806, and a specificity of 0.740. If we included eligible studies from among the references of the identified studies, GPT-3.5 turbo had no critical misses, while GPT-4 had some misses. Our study indicates that GPT-3.5 turbo can be effectively used to classify abstracts for DTA systematic reviews. Further studies using other dataset are warranted to confirm our results. Additionally, we encourage the use of our framework and publicly available dataset for further exploration of more effective classifiers using other LLMs and prompts ( ).
What is already known
What is new
Potential Impact for Readers
### Competing Interest Statement
Yuki Kataoka: none known Ryuhei So: grants from Osake-no-Kagaku Foundation, speakers honoraria from Otsuka Pharmaceutical Co., Ltd., Nippon Shinyaku Co., Ltd., and Takeda Pharmaceutical Co., Ltd., outside the submitted work. Masahiro Banno: none known Junji Kumasawa: none known Hidehiro Someko: none known Shunsuke Taito: none known Teruhiko Terasawa: none known Yasushi Tsujimoto: none known Yusuke Tsutsumi: none known Yoshitaka Wada: none known Toshi A. Furukawa: TAF reports personal fees from DT Axis, Kyoto University Original, MSD,SONY and UpToDate, and a grant from Shionogi, outside the submitted work; In addition, TAF has patents 2020-548587 and 2022-082495 pending, and intellectual properties for Kokoro-app licensed to Mitsubishi-Tanabe.
### Funding Statement
The application programming interface fee was supported by a JSPS Grant-in-Aid for Scientific Research (Grant Number 22K15664) provided to YK. The funder played no role in the study design, data collection and analysis, publication decisions, or manuscript preparation.
### Author Declarations
I confirm all relevant ethical guidelines have been followed, and any necessary IRB and/or ethics committee approvals have been obtained.
Yes
I confirm that all necessary patient/participant consent has been obtained and the appropriate institutional forms have been archived, and that any patient/participant/sample identifiers included were not known to anyone (e.g., hospital staff, patients or participants themselves) outside the research group so cannot be used to identify individuals.
Yes
I understand that all clinical trials and any other prospective interventional studies must be registered with an ICMJE-approved registry, such as ClinicalTrials.gov. I confirm that any such study reported in the manuscript has been registered and the trial registration ID is provided (note: if posting a prospective study registered retrospectively, please provide a statement in the trial ID field explaining why the study was not registered in advance).
Yes
I have followed all appropriate research reporting guidelines, such as any relevant EQUATOR Network research reporting checklist(s) and other pertinent material, if applicable.
Yes
All data produced are available online at ( )
更多查看译文
关键词
language models,large language models,titles,diagnostic test accuracy,meta-prompts
AI 理解论文
溯源树
样例
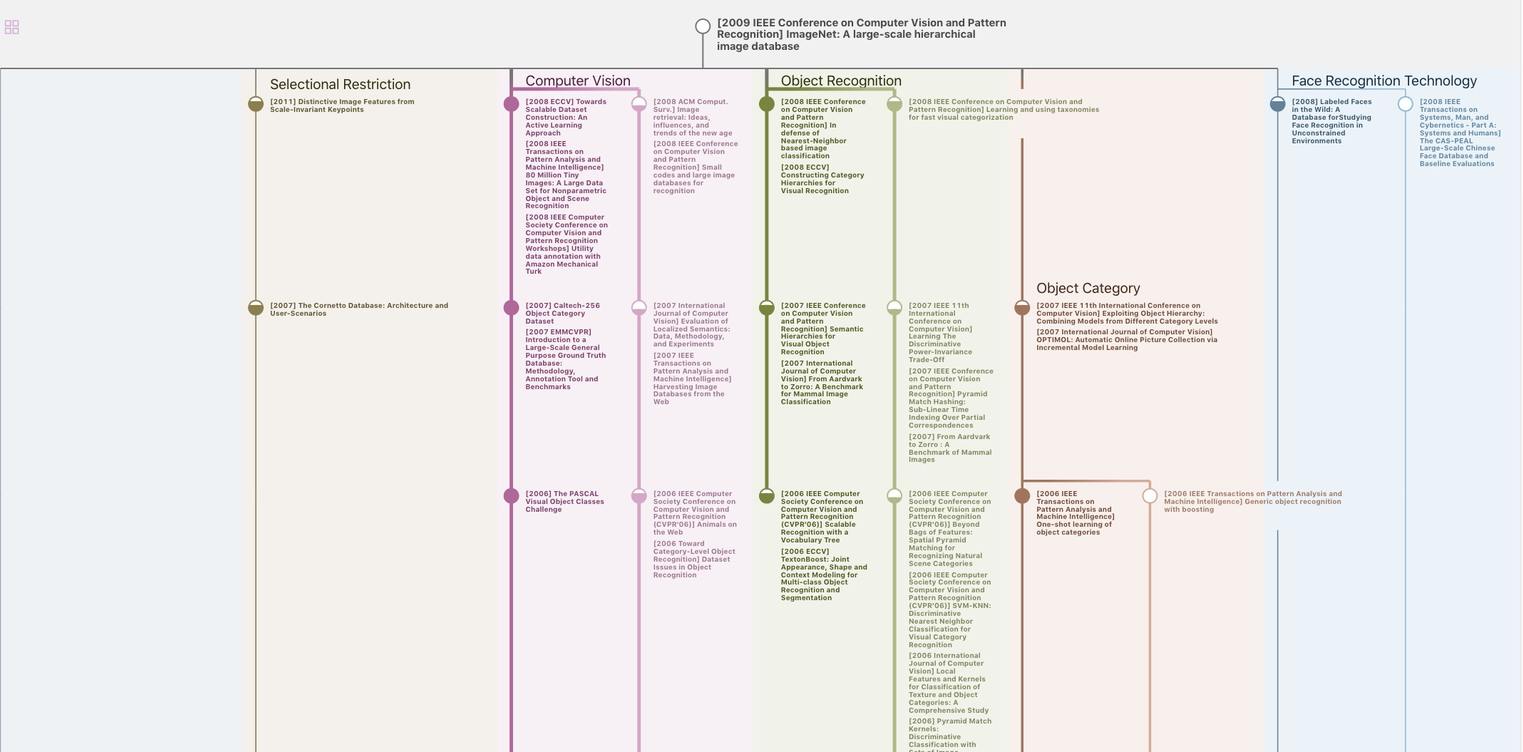
生成溯源树,研究论文发展脉络
Chat Paper
正在生成论文摘要