Identifying Signs and Symptoms of Urinary Tract Infection from Emergency Department Clinical Notes Using Large Language Models
medrxiv(2023)
摘要
Objectives Symptom characterization is critical to urinary tract infection (UTI) diagnosis, but identification of symptoms from the electronic health record (EHR) is challenging, limiting large-scale research, public health surveillance, and EHR-based clinical decision support. We therefore developed and compared two natural language processing (NLP) models to identify UTI symptoms from unstructured emergency department (ED) notes.
Methods The study population consisted of patients aged ≥ 18 who presented to the (ED) in a north-eastern United States health system between June 2013 and August 2021 and had a urinalysis performed. We annotated a random subset of 1,250 ED clinician notes from these visits for a list of 17 UTI symptoms. We then developed two task-specific large language models (LLMs) to perform the task of named entity recognition (NER): a convolutional neural network (CNN)-based model (SpaCy) and a transformer-based model designed to process longer documents (Longformer). Models were trained on 1,000 notes and tested on a holdout set of 250 notes. We compared model performance (precision, recall, F1 measure) at identifying the presence or absence of UTI symptoms at the note level.
Results 8,135 entities were identified in 1,250 notes; 83.6% of notes included at least one entity. Overall F1 measure for note-level symptom identification weighted by entity frequency was 0.84 for the SpaCy model and 0.88 for the Longformer model. F1 measure for identifying presence or absence of any UTI symptom in a clinical note was 0.96 (232/250 correctly classified) for the SpaCy model and 0.98 (240/250 correctly classified) for the Longformer model.
Conclusions The study demonstrated the utility of LLMs and transformer-based models in particular for extracting UTI symptoms from unstructured ED clinical notes; models were highly accurate for detecting the presence or absence of any UTI symptom on the note level, with variable performance for individual symptoms.
### Competing Interest Statement
The authors have declared no competing interest.
### Funding Statement
This publication was made possible by the Yale School of Medicine Fellowship for Medical Student Research.
### Author Declarations
I confirm all relevant ethical guidelines have been followed, and any necessary IRB and/or ethics committee approvals have been obtained.
Yes
The details of the IRB/oversight body that provided approval or exemption for the research described are given below:
The Yale University institutional review board approved this research and waived the need for informed consent (HIC# 1602017249).
I confirm that all necessary patient/participant consent has been obtained and the appropriate institutional forms have been archived, and that any patient/participant/sample identifiers included were not known to anyone (e.g., hospital staff, patients or participants themselves) outside the research group so cannot be used to identify individuals.
Yes
I understand that all clinical trials and any other prospective interventional studies must be registered with an ICMJE-approved registry, such as ClinicalTrials.gov. I confirm that any such study reported in the manuscript has been registered and the trial registration ID is provided (note: if posting a prospective study registered retrospectively, please provide a statement in the trial ID field explaining why the study was not registered in advance).
Yes
I have followed all appropriate research reporting guidelines, such as any relevant EQUATOR Network research reporting checklist(s) and other pertinent material, if applicable.
Yes
All data produced in the present study are available upon reasonable request to the authors.
更多查看译文
AI 理解论文
溯源树
样例
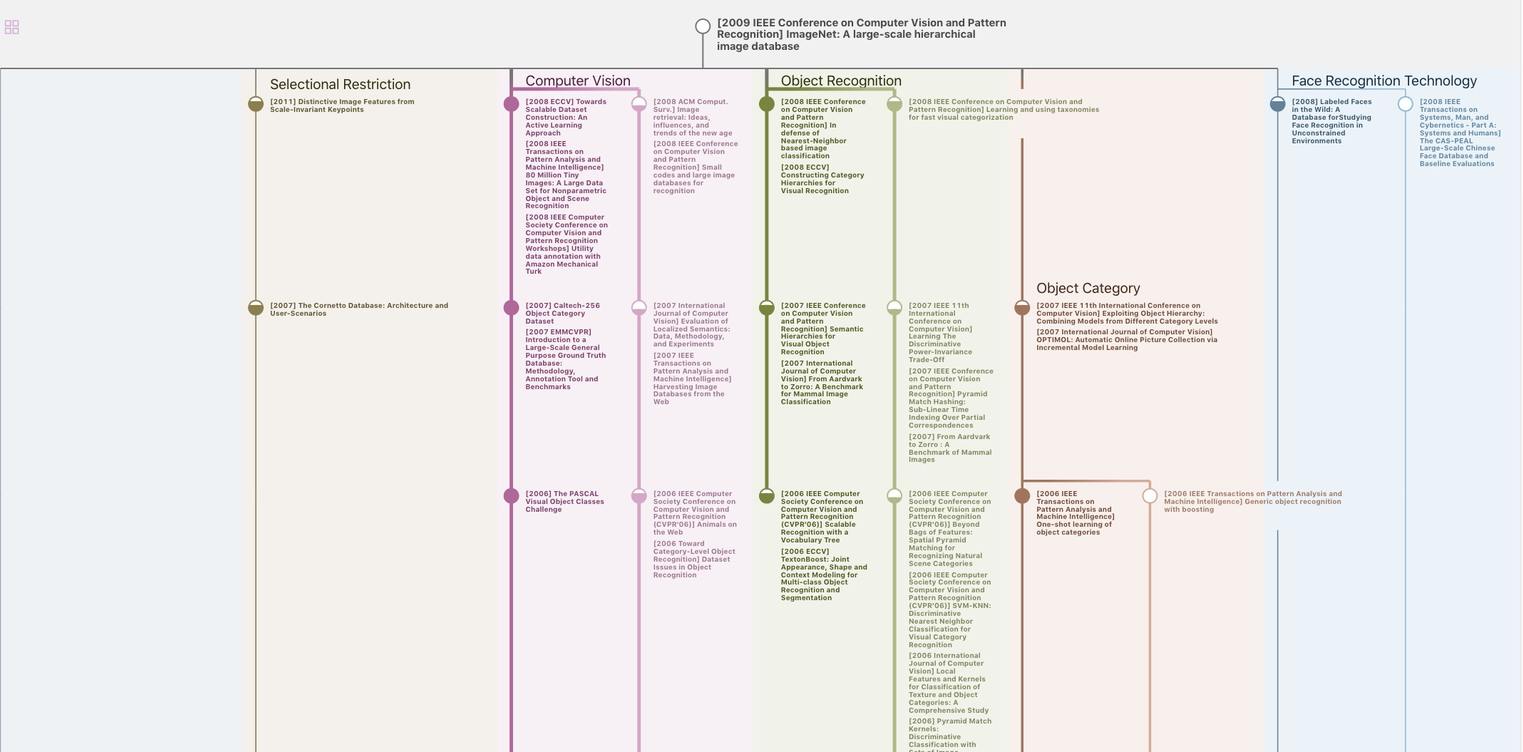
生成溯源树,研究论文发展脉络
Chat Paper
正在生成论文摘要