Impact of urban spatial factors on NO2 concentration based on different socio-economic restriction scenarios in US cities
Atmospheric Environment(2024)
摘要
The impact of socio-economic restriction measures in the United States showed a significant reduction in nitrogen dioxide (NO2) emissions due to the changes in commuting patterns, travel restrictions, and reduced social and economic activities. The data from 60 observation stations across different states representing of different urban settings were used to assess the impacts of dynamic changes in human mobility and static emission sources on NO2 concentrations. Two predictive models, Seasonal Autoregressive Integrated Moving Average with eXogenous (SARIMAX) and Long Short-Term Memory (LSTM), were used to forecast NO2 concentrations under a counterfactual scenario in the assumption of no lockdown was implemented. The predicted concentrations were then compared to the observed concentrations to evaluate the difference between the two scenarios, during lockdown and after lockdown by using the last five-years (2015-2019) average as baseline. Results showed that reduction in NO2 varied across stations due to a combination of factors such as human mobility, population density, income, local climate, and stationary sources of NO2 emissions in the neighbourhood. The stations located in high-income regions with higher population density experienced a greater reduction in mobility, resulting in a greater variation in NO2 levels during both periods. In contrast, stations in densely populated lowincome regions experienced lower variation in mobility and NO2 levels during the lockdown period but showed recovery during the post-lockdown period. Stations located outside the city with very low population density experienced no significant reduction in NO2 emissions. The study highlights the disparities in NO2 reduction and income inequality exposed by the pandemic, which may have scientific impacts on air quality and health disparities.
更多查看译文
关键词
Air pollution,Time-series forecasting,Public health,Socio-economic restrictions,Local neighbourhood
AI 理解论文
溯源树
样例
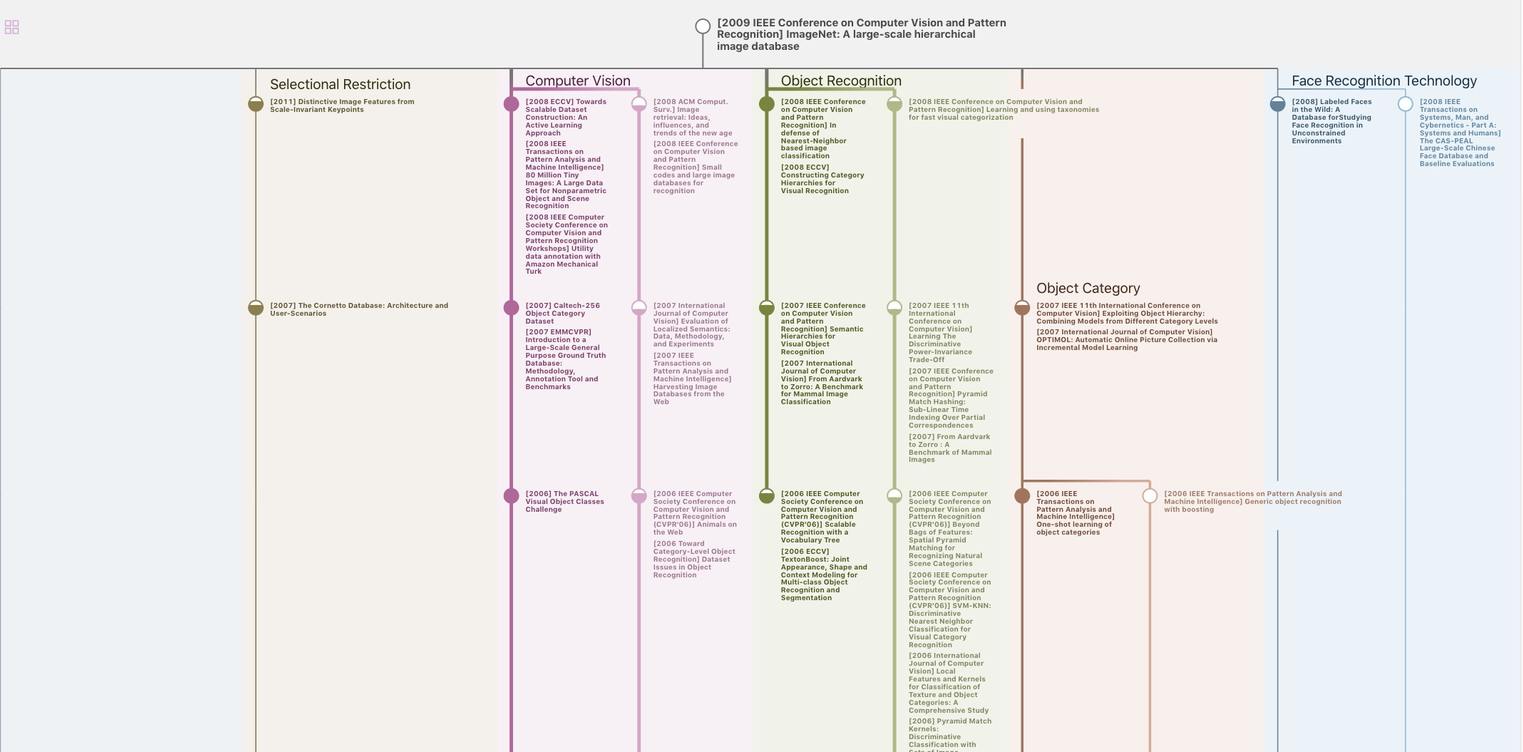
生成溯源树,研究论文发展脉络
Chat Paper
正在生成论文摘要