Towards Generalised Pre-Training of Graph Models
arxiv(2023)
摘要
The principal benefit of unsupervised representation learning is that a
pre-trained model can be fine-tuned where data or labels are scarce. Existing
approaches for graph representation learning are domain specific, maintaining
consistent node and edge features across the pre-training and target datasets.
This has precluded transfer to multiple domains.
In this work we present Topology Only Pre-Training, a graph pre-training
method based on node and edge feature exclusion. Separating graph learning into
two stages, topology and features, we use contrastive learning to pre-train
models over multiple domains. These models show positive transfer on evaluation
datasets from multiple domains, including domains not present in pre-training
data. On 75
better than a supervised baseline. These results include when node and edge
features are used in evaluation, where performance is significantly better on
85.7
show that out-of-domain topologies can produce more useful pre-training than
in-domain. We show better transfer from non-molecule pre-training, compared to
molecule pre-training, on 79
更多查看译文
AI 理解论文
溯源树
样例
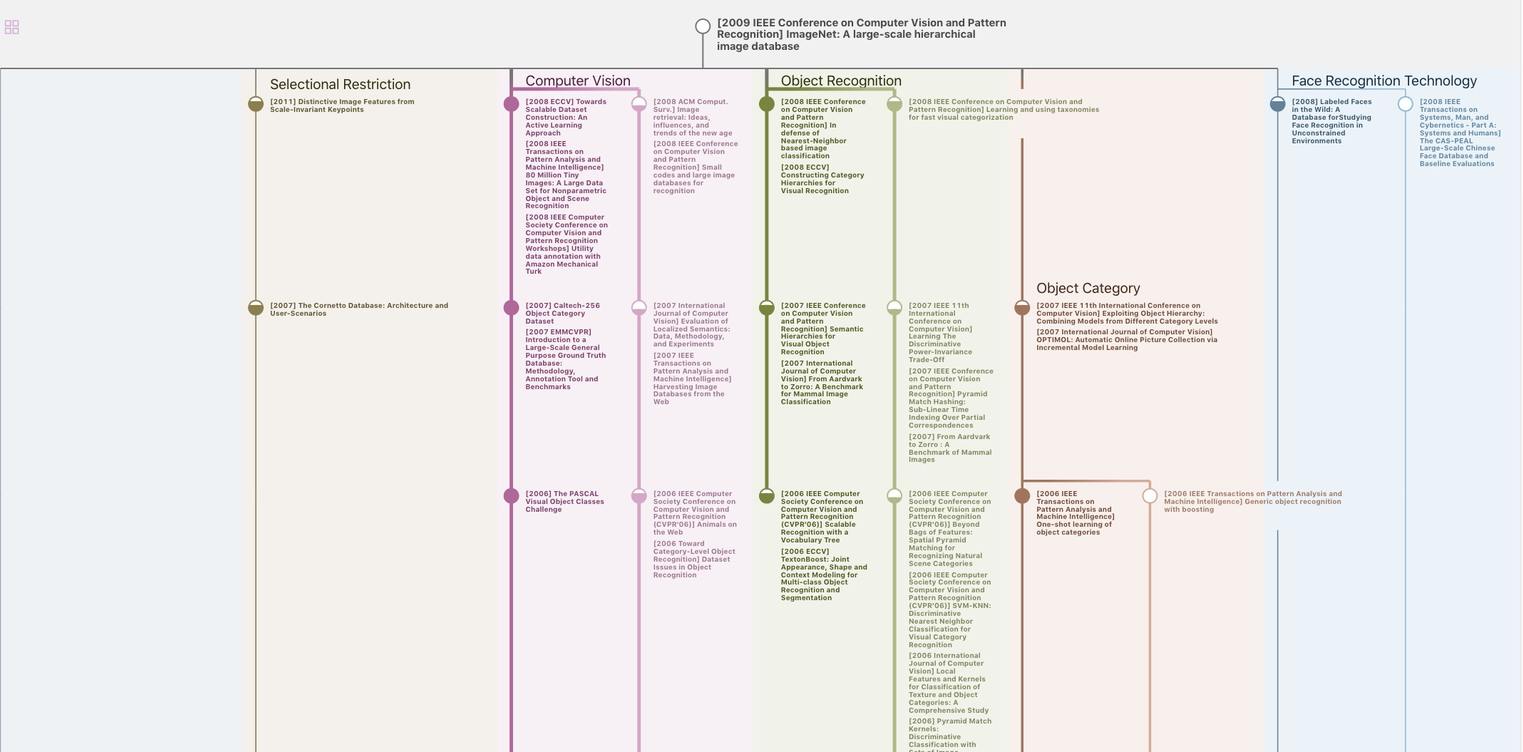
生成溯源树,研究论文发展脉络
Chat Paper
正在生成论文摘要