SCMNet: Toward Subsurface Chlorophyll Maxima Prediction Using Embeddings and Bi-GRU Network
IEEE Journal of Selected Topics in Applied Earth Observations and Remote Sensing(2023)
摘要
A subsurface chlorophyll maximum is an important ecological feature of planktonic ecosystems. Although the vertical profiles can be determined through the implementation of biogeochemical (BGC)-Argo buoy, this method is not compatible with the ocean observation requirements of high-resolution spatiotemporal measurements. Here, we demonstrate that deep learning can proficiently fill in these observational gaps when combined with sea surface data from ocean color radiometry. First, the sparse vertical profile data of BGC-Argo is fused with sea surface data to construct the benchmark dataset for deep learning. Second, encouraged by the idea of dense numerical representations, the comprehensive model combined with coupled embedding and bidirectional gated recurrent unit is proposed to inverse the vertical profile with BGC-Argo and satellite data. Then, the in-depth spatiotemporal analysis of the subsurface chlorophyll maxima phenomenon is performed by the parametric equation method and deep learning method as well. Finally, extensive experiments in the Northwest Pacific were conducted to demonstrate the effectiveness of the proposed methodology. The impressive results indicate that the proposed method can compensate for the lack of sparse in situ observations of chlorophyll concentration, the determination coefficient is increased by more than 20%. This study is of great significance to marine ecology and provides important insight into artificial intelligence in the study of subsurface oceanic phenomena.
更多查看译文
关键词
Sea surface,Oceans,Meters,Logic gates,Spatial resolution,Sea measurements,Satellites,Biogeochemical (BGC)-Argo,bidirectional gated recurrent unit (bi-GRU) network,deep embedding,remote sensing,subsurface chlorophyll maxima
AI 理解论文
溯源树
样例
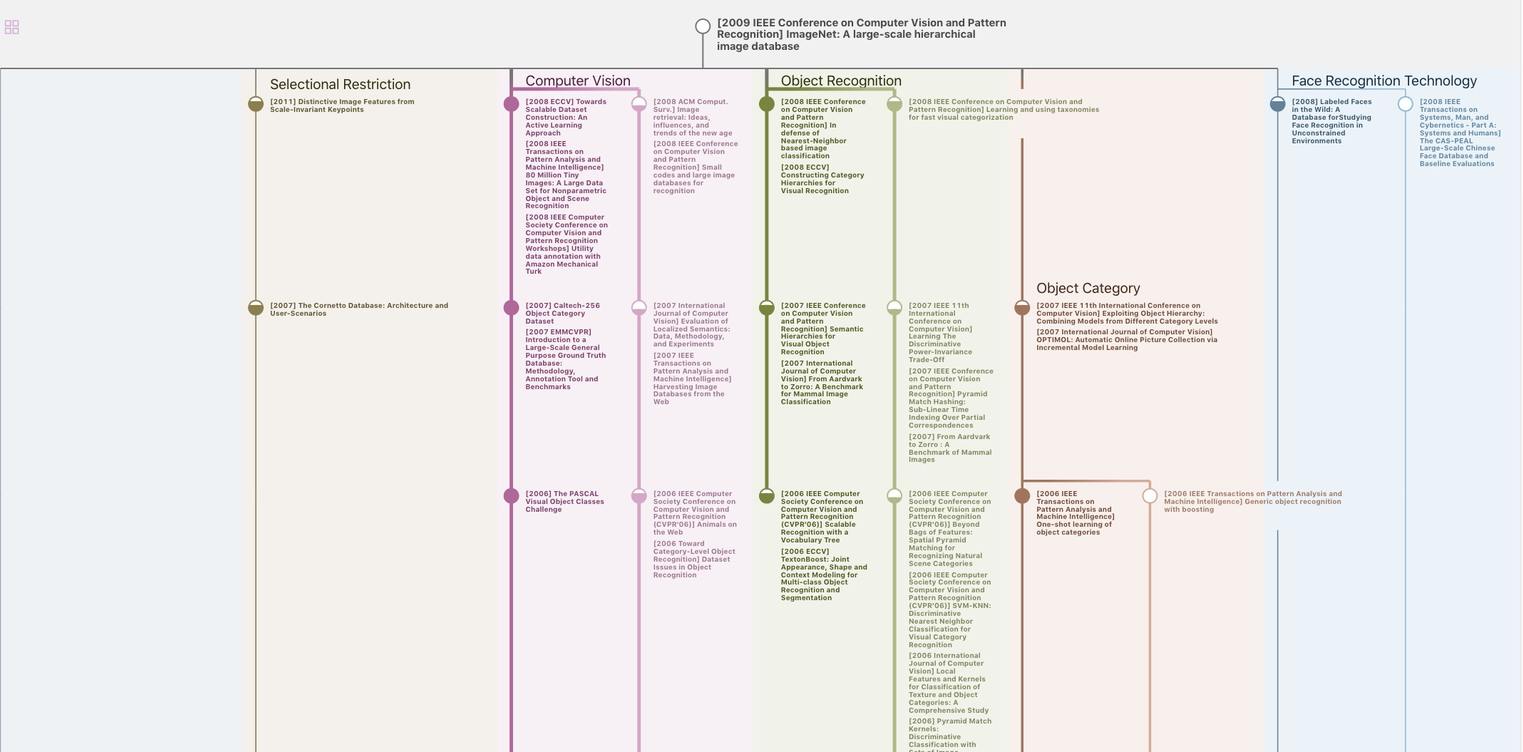
生成溯源树,研究论文发展脉络
Chat Paper
正在生成论文摘要