Unsupervised Transfer Learning Approach With Adaptive Reweighting and Resampling Strategy for Inter-Subject EOG-Based Gaze Angle Estimation
IEEE JOURNAL OF BIOMEDICAL AND HEALTH INFORMATICS(2024)
摘要
Gaze estimation based on electrooculograms (EOGs) has been widely explored. However, the inter-subject variability of EOGs still leaves a significant challenge for practical applications. It contributes to performance degradation when handling inter-subject issues. In this paper, an unsupervised transfer learning approach with an adaptive reweighting and resampling (ARR) strategy to fully consider individual variability is proposed for EOG-based gaze angle estimation. It allows quantifying domain shifts by leveraging the source-target similarities, reweighting and resampling the source data to retain relevant instances and disregard irrelevant instances during adaptation. Specifically, our proposed methodology first assesses the domain shifts via decomposing transformation matrices, which are estimated between the training subjects (denoted as multi-source domains) and the test subject (denoted as target domain). Then, the multi-domain shifts are assigned as weighted indicators to resample the multi-source domains for model training. Comparative experiments with several prevailing transfer learning methods including CORrelation ALignment (CORAL), Geodesic Flow Kernel (GFK), Joint Distribution Adaptation (JDA), Transfer component analysis (TCA), and Balanced distribution adaption (BDA) using two different normalization processes were conducted on a realistic scenario across 18 subjects. Experimental results demonstrate that the ARR strategy can significantly improve performance (mean absolute error (MAE) reduction: 7.0%, root mean square error (RMSE) reduction: 6.3%), outperforming the prevailing methods. Besides, the impacts of data diversity and data size on ARR strategy are further investigated. It exhibits that data size is more important than data diversity for EOG-based gaze angle estimation, and also presents the benefits of the ARR strategy for dealing with practical scenarios.
更多查看译文
关键词
Adaptive reweighting,EOG,individual variability,resampling strategy and transfer learning
AI 理解论文
溯源树
样例
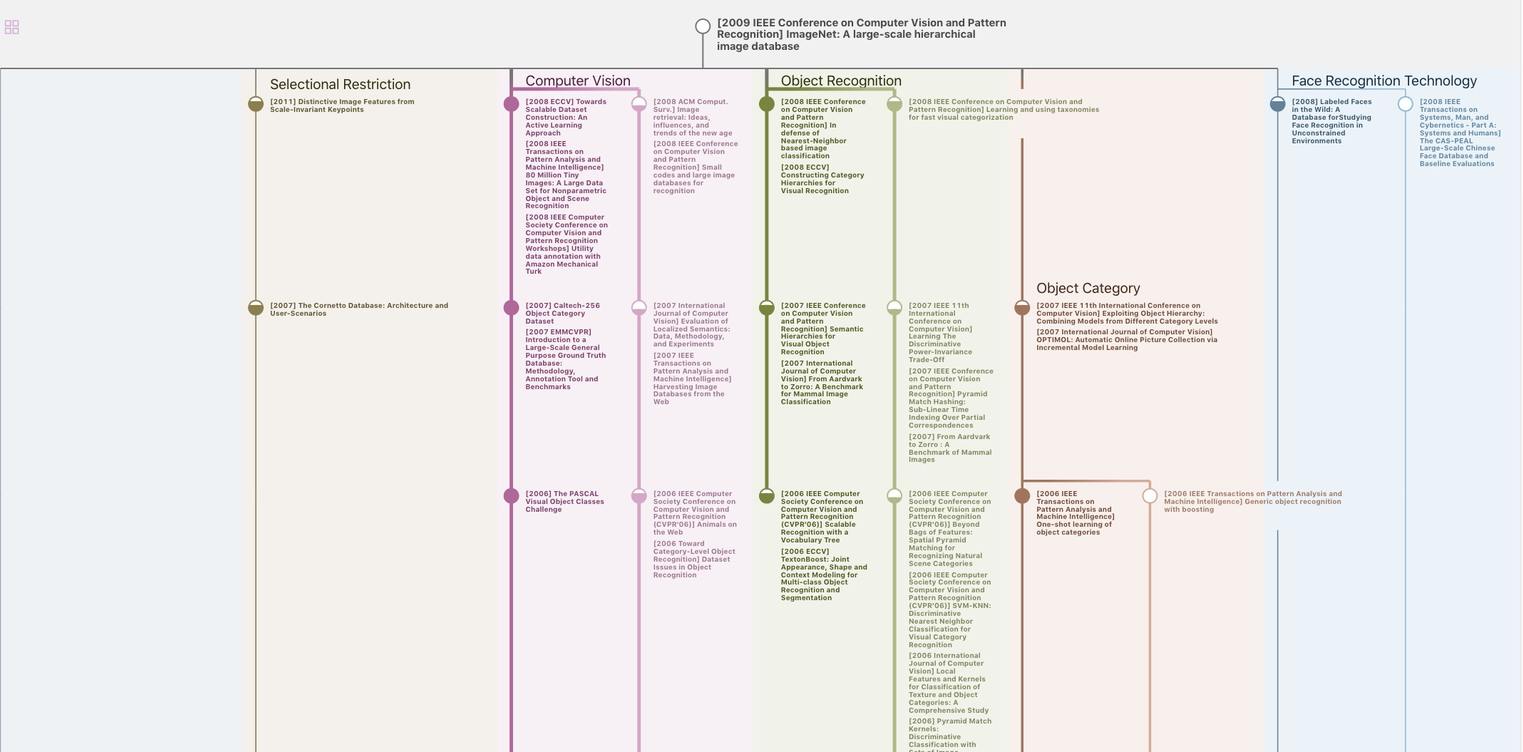
生成溯源树,研究论文发展脉络
Chat Paper
正在生成论文摘要