A novel Gaussian process regression-based stock index interval forecasting model integrating optimal variables screening with bidirectional long short-term memory
Soft Computing(2024)
摘要
Stock index forecasting has always been an interesting subject for investors and related scholars. Accurately stock index forecasting can provide some helpful suggestions for investors and keep financial markets stable. In this study, a new forecasting system, including point prediction and interval prediction, has been proposed to predict the stock index. For obtaining a better predictive effect, multiple influencing variables are also considered in the novel model. More specifically, in the point prediction models, this study applies gradient boosting decision tree (GBDT) to choose some variables related to the stock index by determining their contribution to accurate prediction. Next, an autoencoder (AE) is utilized to reduce the dimensionality of screened factors for the purpose of reducing the effect of noise and improving the efficiency of forecasting. These reconstructed features are all inputted into bidirectional long short-term memory (BiLSTM) to do point prediction. The interval prediction is based on point prediction results and Gaussian process regression (GPR), intended to quantitative uncertainty of the variables. This study chooses the Chinese stock index including the Shanghai Securities Composite Index (SSEC), Shenzhen Composite Index (SZI) and China Securities Index 300 (CSI300) to demonstrate the validity of the innovative hybrid model. Furthermore, this study also selects some other models for comparison. Evaluating the performance of the novel hybrid model, it could be considered as a valid way to do stock index forecasting.
更多查看译文
关键词
Predictive variables screening,Improved dimensionality reduction,Bidirectional long short-term memory,Interval forecasting,Stock index
AI 理解论文
溯源树
样例
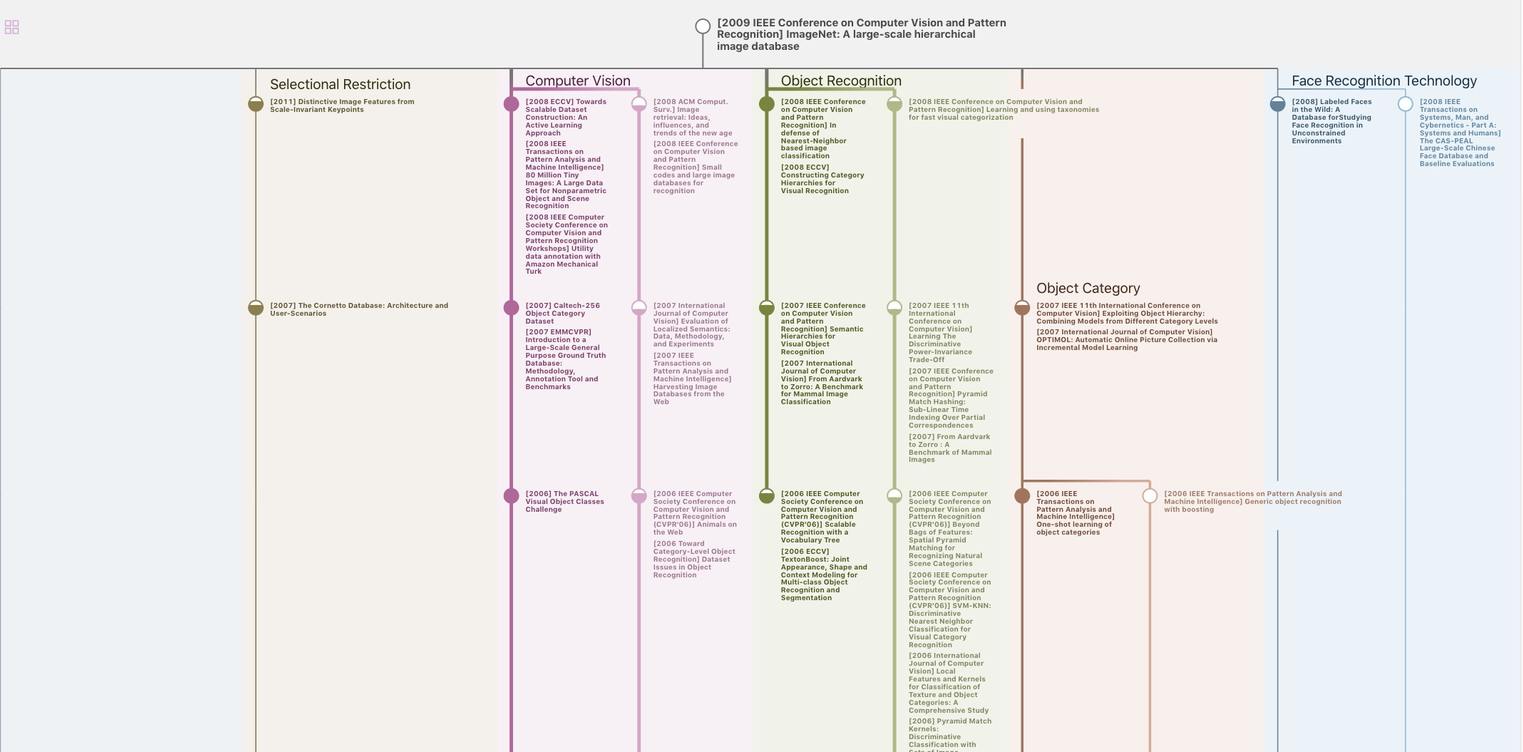
生成溯源树,研究论文发展脉络
Chat Paper
正在生成论文摘要