CCSMP: an Efficient Closed Contiguous Sequential Pattern Mining Algorithm with a Pattern Relation Graph
Applied intelligence(2023)
摘要
The closed contiguous sequential pattern combines the advantages of closedness constraints and contiguity constraints and in recent years has been widely used in the fields of sequence classification, traffic trajectory visualization and football player trajectory analysis. Most of the previously developed closed contiguous sequential pattern mining algorithms pose some challenges. For instance, CCSpan, BP-CCSM, and LCCspm cannot mine the large-scale sequence database with reasonable time and memory usage, while C3Ro, which can mine patterns with multiple constraints, does not consider the specificity induced by the contiguity constraint of the pattern. To address these problems and improve the efficiency of mining closed contiguous sequential patterns, in this paper, we present an algorithm called CCSMP based on the pattern relation graph. Pattern relation graph is a novel data structure that has some key properties related to closed contiguous sequential pattern mining. In the experimental section, we not only conducted extensive experiments on real datasets to evaluate the performance and scalability of CCSMP but also analyzed the running time of each step of CCSMP to verify the effectiveness of the pattern relation graph. The experimental results show that CCSMP outperforms the existing state-of-the-art algorithm in most cases and that the use of the pattern relation graph can significantly reduce the time for closure checking.
更多查看译文
关键词
Sequential pattern mining,Closed contiguous sequential pattern,Performance
AI 理解论文
溯源树
样例
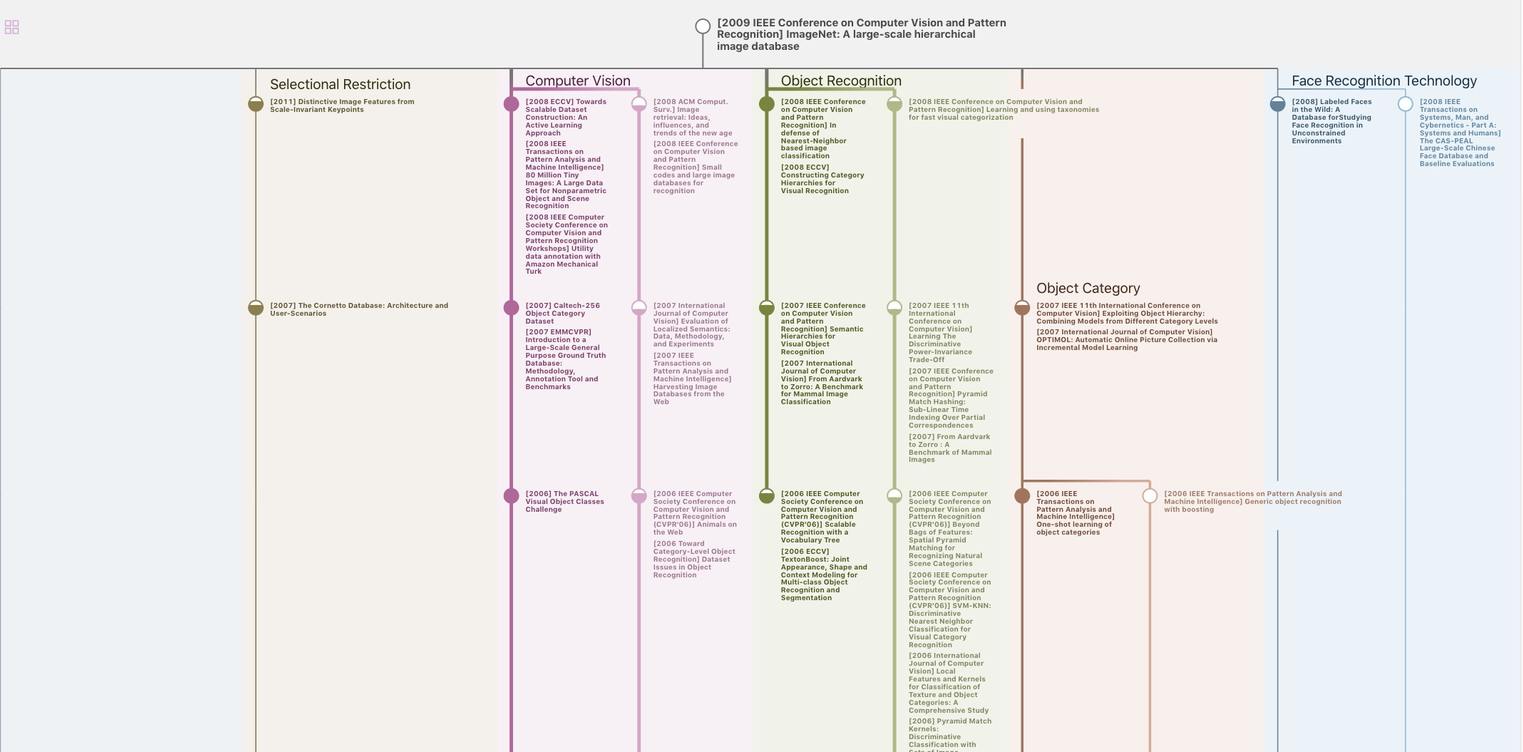
生成溯源树,研究论文发展脉络
Chat Paper
正在生成论文摘要