Applying a neural network machine learning model to predict seasonal allelopathic inhibitory effects of Myriophyllum spicatum on the growth of Microcystis aeruginosa
AQUATIC ECOLOGY(2023)
摘要
Cyanobacterial harmful algal blooms (cyanoHABs) are extremely detrimental to the environment and cause sizable economic losses. Microcystis aeruginosa is reported to be inhibited by Eurasian watermilfoil ( Myriophyllum spicatum ), and onset of the inhibitory effects of M. spicatum varied depending on the seasons. This study aimed to investigate the seasonal allelopathy effects in the metabolomes of M. spicatum using gas chromatography–mass spectrometry and predict the most effective season for its allelopathic inhibitory effects on the growth of M. aeruginosa . A machine learning approach using multi-layer perceptron was used to predict the season with maximum anti-cyanobacterial potential. The prediction model suggested that M. spicatum collected in August would have higher growth-inhibiting effects than other months with 93.6 (± 2.9) likelihood. These results were consistent with coexistence experiments where M. spicatum collected in August showed the earliest onset of inhibition. The study concluded that the inhibitory potential of M. spicatum on cyanobacterial growth was strong in the summer, especially in August. This suggests that neural network machine learning can be applied to a variety of topics using accumulated data, making clearer and more useful predictions possible even in multivariate and complex environmental data.
更多查看译文
关键词
Myriophyllum spicatum
AI 理解论文
溯源树
样例
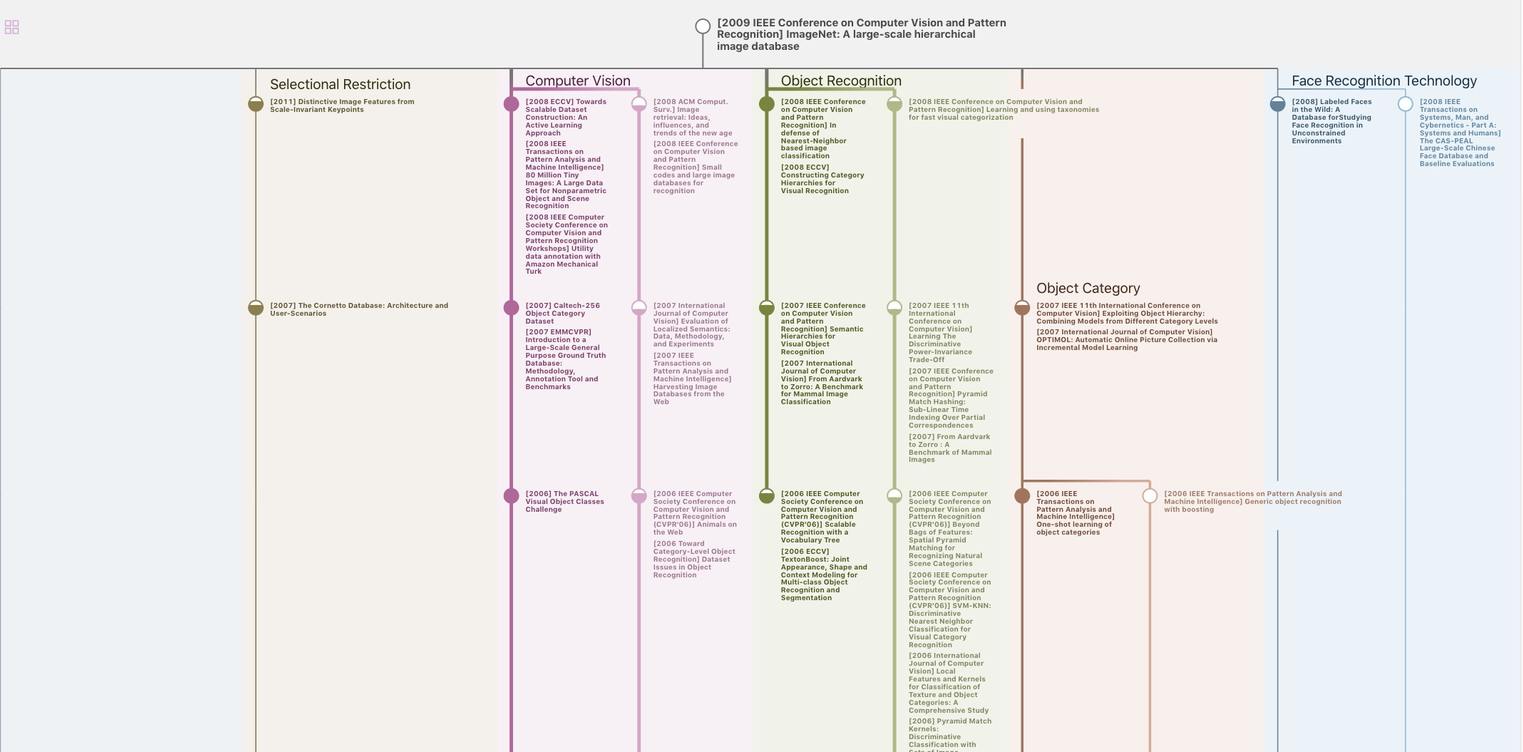
生成溯源树,研究论文发展脉络
Chat Paper
正在生成论文摘要