MINING: Multi-Granularity Network Alignment Based on Contrastive Learning
IEEE TRANSACTIONS ON KNOWLEDGE AND DATA ENGINEERING(2023)
摘要
Network alignment aims to discover nodes in different networks belonging to the same identity. In recent years, the network alignment problem has aroused significant attentions in both industry and academia. However, the continuous exploding of network data brings two challenges in solving the network alignment problem, i.e., large network scale and scarce labeled data. To bridge this gap, in this paper we propose a novel approach termed as Multi-granularIty Network alIgnment based on coNtrastive learninG (MINING). Specifically, in MINING, we first design multi-granularity alignment framework to solve the issue of large network scale. Then, we design intra-and inter-network contrastive learning to solve the issue of scarce labeled data. Moreover, we provide theoretical proofs to demonstrate the effectiveness of MINING. Finally, we conduct extensive experiments on the benchmark datasets of Facebook-Twitter, AMiner-LinkedIn and DBpediaZH-DBpediaEN, and results show that MINING can averagely achieve 15.93% higher Hits@ k and 14.82% higher MRR@ k compared with the state-of-the-art methods.
更多查看译文
关键词
Contrastive learning,deep learning,machine learning,network alignment
AI 理解论文
溯源树
样例
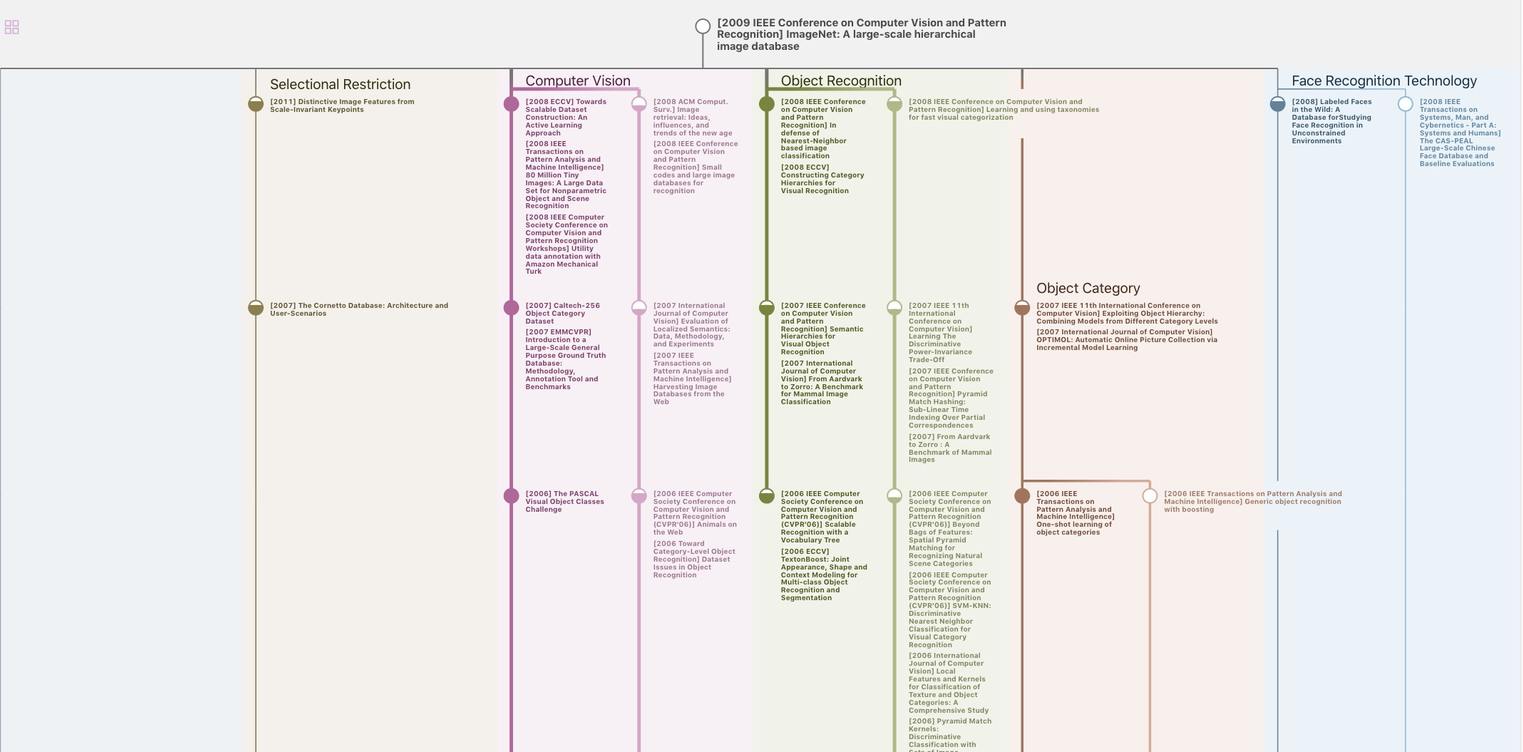
生成溯源树,研究论文发展脉络
Chat Paper
正在生成论文摘要