Temporal Heterogeneous Information Network Embedding via Semantic Evolution
IEEE TRANSACTIONS ON KNOWLEDGE AND DATA ENGINEERING(2023)
摘要
Real-world networks are often heterogeneous and constantly changing over time. Evolution reveals the trend of network development, which is vital for predicting its future state, and network embedding can effectively learn the information from it. Nevertheless, previous works only consider the impact of meta-path instances or node neighbors on the network dynamics but ignore the relationship between them, and hence the hidden semantic information is missed, which will result in performance deterioration. Therefore, we propose a novel temporal heterogeneous information network embedding method (SemE), which abstracts the instance of the meta-path as semantic units and then considers the interaction between them to discover deeper semantic information. Specifically, we first construct semantic networks by the Ethernet topology and the interaction between semantic units. The semantic units are sampled based on a pre-designed meta-path-guided random walk. To further capture the semantic evolution of the semantic network, we learn the embedding of nodes by the attention-Hawkes process. Finally, we generate the final embedding by aggregating the structure, semantic and temporal information with the attention mechanism. Experiments on three real-world temporal heterogeneous information networks show that SemE performs better than competitive counterparts.
更多查看译文
关键词
Network embedding,semantic evolution,temporal heterogeneous information network
AI 理解论文
溯源树
样例
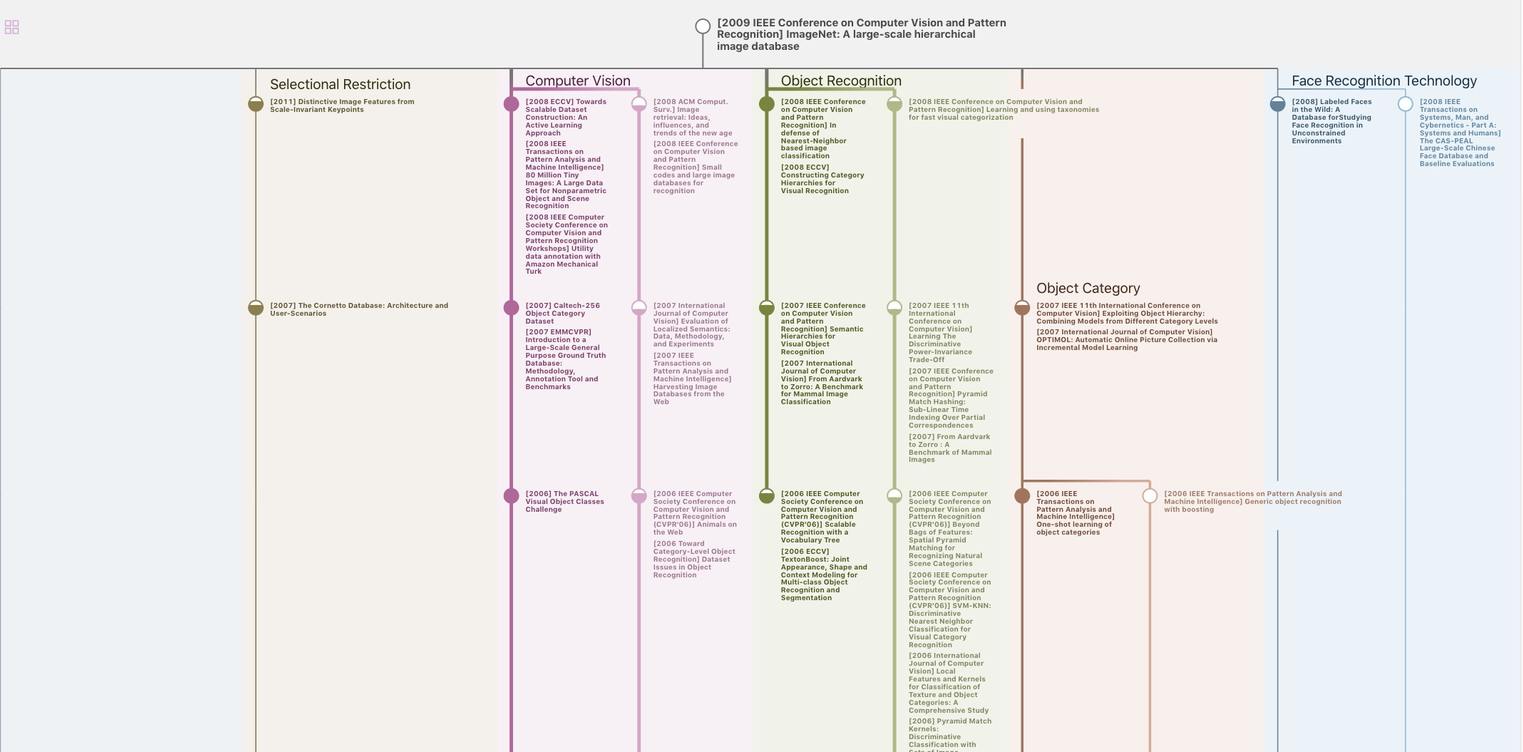
生成溯源树,研究论文发展脉络
Chat Paper
正在生成论文摘要