Dense-and-Similar Object detection in aerial images
PATTERN RECOGNITION LETTERS(2023)
摘要
The general object detection performance has been improving significantly due to the prosperity of deep learning. When applied to aerial images, these algorithms perform poorly. There are, as we summarized, two practical reasons: (1) photographed from far above, objects are commonly small in size, dense in clusters, but sparsely scattered in the whole image; (2) some classes are naturally similar in appearance, not to mention the insufficiency of distinguishable visual features captured by cameras due to factors such as bad weather, various angles of view and long distances, etc. To address the two issues, we propose a dense-and-similar object detector with four key components: a coarse detector, an adaptive clustering procedure, a similar-class classifier, and a fine detector. At first, we input the original image into both the coarse and fine detectors. Then we cluster and patch the coarsely detected results adaptively to form a foreground region image. We feed the patched image into the coarse detector again and use the similar-class classifier to re-identify the labels of detected bounding boxes. At last, we put re-identified detections and outputs of the fine detector together, and obtain the final detections after non-maximal suppression. The approach proposed in this study achieved excellent results on both the VisDrone 2019 and DIOR datasets through experimental validation.
更多查看译文
关键词
Dense and small object,Similar object,Confusion categories,Object detection,Aerial images
AI 理解论文
溯源树
样例
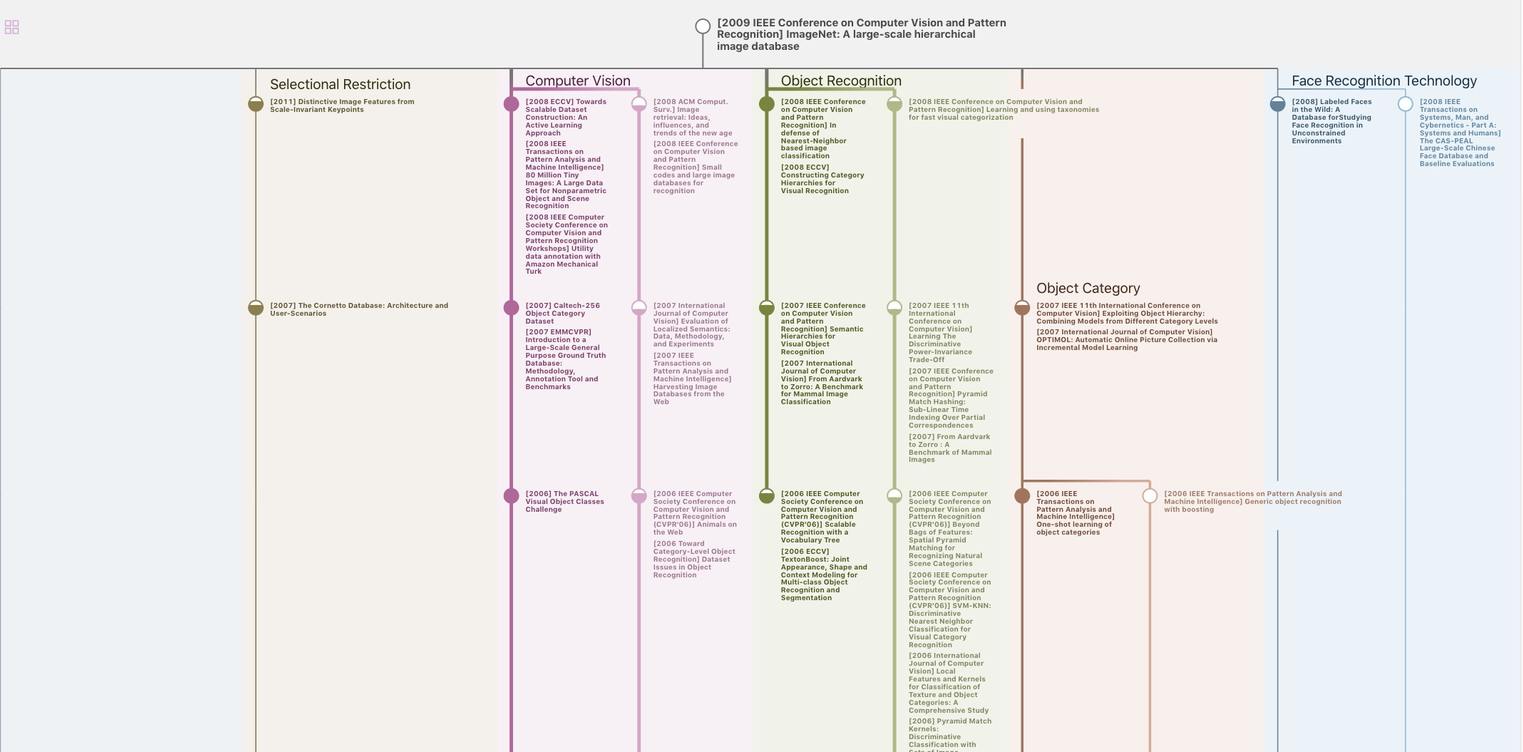
生成溯源树,研究论文发展脉络
Chat Paper
正在生成论文摘要