Time-space separation-based data driven method for monitoring distributed parameter process with sparse and noisy sensor data
CONTROL ENGINEERING PRACTICE(2024)
摘要
In industrial applications, real-time monitoring of the distributed parameter processes is a difficult task, due to the infinite-dimensional nature and the nonlinear spatiotemporal dynamics of distributed parameter systems (DPSs). In addition, the limited number of sensors and the existence of measurement noise may result in failure for detecting DPS dynamics. In this study, a data-driven model is proposed to reconstruct the spatiotemporal states in DPSs using sparse and noisy sensor data based on the time/space separation theory. The proposed reconstruction model maps the high-dimensional DPS states into low-dimensional spatial features using an autoencoder-based spatial model, and then predicts temporal evolution of the spatial features based on the long short-term memory network (LSTM). In addition, in order to improve robustness of the model, a random noise layer is developed and is integrated with the reconstruction model to extract robust spatiotemporal features. The performance of the proposed model is illustrated through applications in two case studies including a thermal process of lithium-ion battery and a fluid process in oceanography. The effects of sensor number and noise intensity on the proposed spatiotemporal model for monitoring DPSs are quantitatively investigated. In addition, the proposed spatiotemporal model is compared with proper orthogonal decomposition-based model. Research results demonstrate that the proposed spatiotemporal model is able to produce accurate and robust reconstruction results for monitoring distributed parameter processes with sparse and noisy sensor data.
更多查看译文
关键词
Process monitoring,Distributed parameter process,Deep learning,Sensors,Lithium -ion battery
AI 理解论文
溯源树
样例
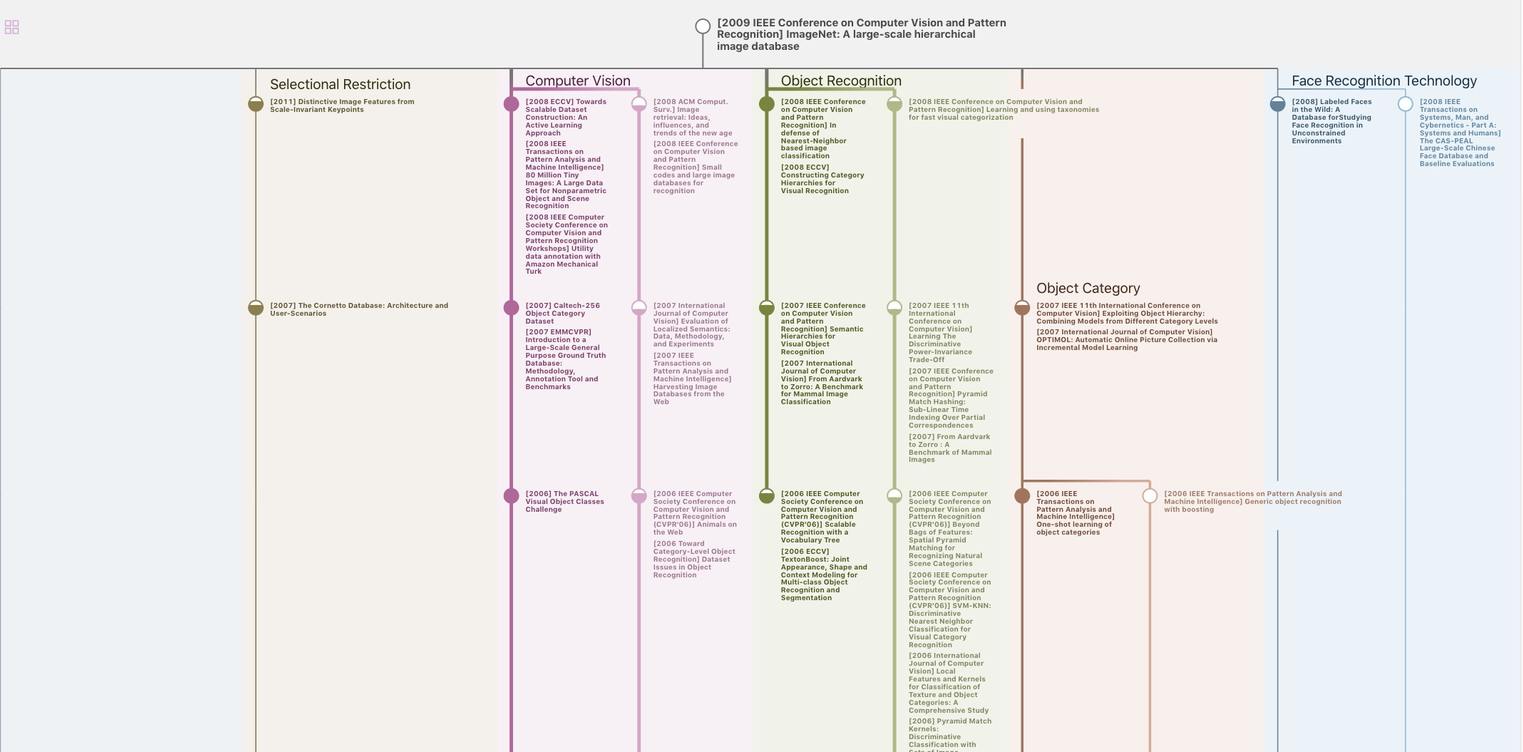
生成溯源树,研究论文发展脉络
Chat Paper
正在生成论文摘要