A 3D generative model of pathological multi-modal MR images and segmentations
International Conference on Medical Image Computing and Computer-Assisted Intervention(2023)
摘要
Generative modelling and synthetic data can be a surrogate for real medical
imaging datasets, whose scarcity and difficulty to share can be a nuisance when
delivering accurate deep learning models for healthcare applications. In recent
years, there has been an increased interest in using these models for data
augmentation and synthetic data sharing, using architectures such as generative
adversarial networks (GANs) or diffusion models (DMs). Nonetheless, the
application of synthetic data to tasks such as 3D magnetic resonance imaging
(MRI) segmentation remains limited due to the lack of labels associated with
the generated images. Moreover, many of the proposed generative MRI models lack
the ability to generate arbitrary modalities due to the absence of explicit
contrast conditioning. These limitations prevent the user from adjusting the
contrast and content of the images and obtaining more generalisable data for
training task-specific models. In this work, we propose brainSPADE3D, a 3D
generative model for brain MRI and associated segmentations, where the user can
condition on specific pathological phenotypes and contrasts. The proposed joint
imaging-segmentation generative model is shown to generate high-fidelity
synthetic images and associated segmentations, with the ability to combine
pathologies. We demonstrate how the model can alleviate issues with
segmentation model performance when unexpected pathologies are present in the
data.
更多查看译文
关键词
3d generative model,segmentations,images,mr,multi-modal
AI 理解论文
溯源树
样例
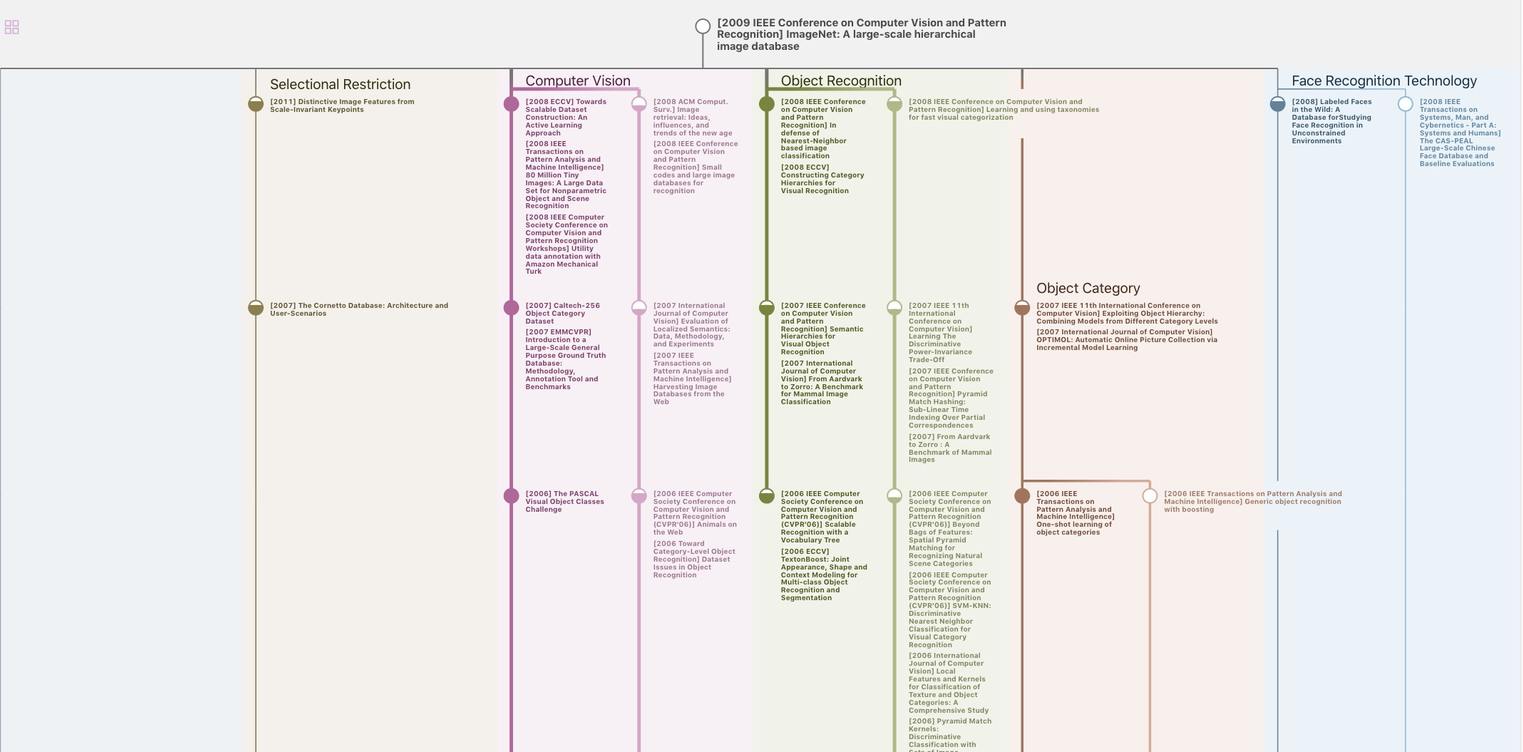
生成溯源树,研究论文发展脉络
Chat Paper
正在生成论文摘要