Inferring delays in partially observed gene regulation processes
BIOINFORMATICS(2023)
摘要
Motivation Cell function is regulated by gene regulatory networks (GRNs) defined by protein-mediated interaction between constituent genes. Despite advances in experimental techniques, we can still measure only a fraction of the processes that govern GRN dynamics. To infer the properties of GRNs using partial observation, unobserved sequential processes can be replaced with distributed time delays, yielding non-Markovian models. Inference methods based on the resulting model suffer from the curse of dimensionality.Results We develop a simulation-based Bayesian MCMC method employing an approximate likelihood for the efficient and accurate inference of GRN parameters when only some of their products are observed. We illustrate our approach using a two-step activation model: an activation signal leads to the accumulation of an unobserved regulatory protein, which triggers the expression of observed fluorescent proteins. With prior information about observed fluorescent protein synthesis, our method successfully infers the dynamics of the unobserved regulatory protein. We can estimate the delay and kinetic parameters characterizing target regulation including transcription, translation, and target searching of an unobserved protein from experimental measurements of the products of its target gene. Our method is scalable and can be used to analyze non-Markovian models with hidden components.Availability and implementation Our code is implemented in R and is freely available with a simple example data at https://github.com/Mathbiomed/SimMCMC.
更多查看译文
关键词
regulation processes,delays,gene
AI 理解论文
溯源树
样例
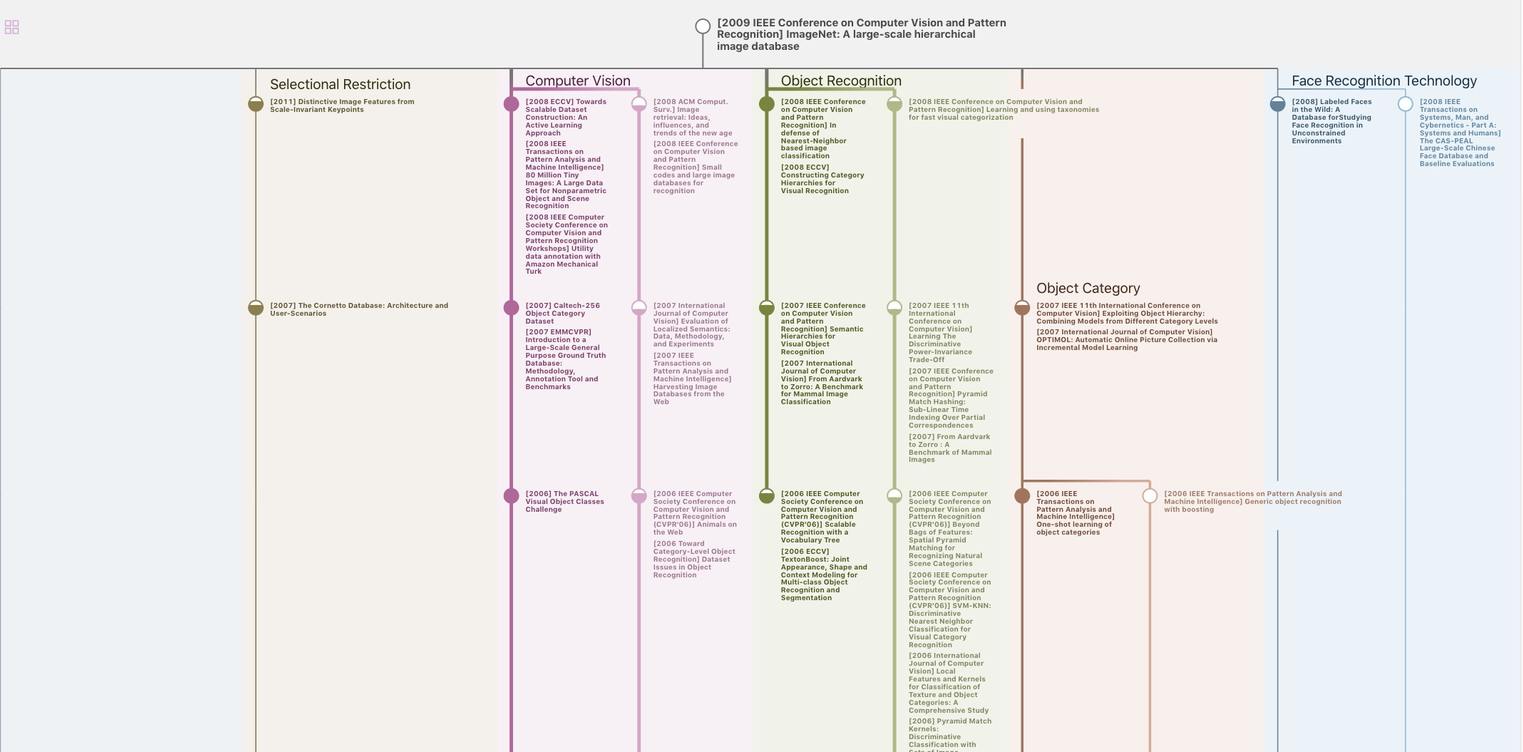
生成溯源树,研究论文发展脉络
Chat Paper
正在生成论文摘要