Strategic Distribution Shift of Interacting Agents via Coupled Gradient Flows.
NeurIPS(2023)
摘要
We propose a novel framework for analyzing the dynamics of distribution shift in real-world systems that captures the feedback loop between learning algorithms and the distributions on which they are deployed. Prior work largely models feedback-induced distribution shift as adversarial or via an overly simplistic distribution-shift structure. In contrast, we propose a coupled partial differential equation model that captures fine-grained changes in the distribution over time by accounting for complex dynamics that arise due to strategic responses to algorithmic decision-making, non-local endogenous population interactions, and other exogenous sources of distribution shift. We consider two common settings in machine learning: cooperative settings with information asymmetries, and competitive settings where a learner faces strategic users. For both of these settings, when the algorithm retrains via gradient descent, we prove asymptotic convergence of the retraining procedure to a steady-state, both in finite and in infinite dimensions, obtaining explicit rates in terms of the model parameters. To do so we derive new results on the convergence of coupled PDEs that extends what is known on multi-species systems. Empirically, we show that our approach captures well-documented forms of distribution shifts like polarization and disparate impacts that simpler models cannot capture.
更多查看译文
关键词
gradient flows,distribution,shift,non-local
AI 理解论文
溯源树
样例
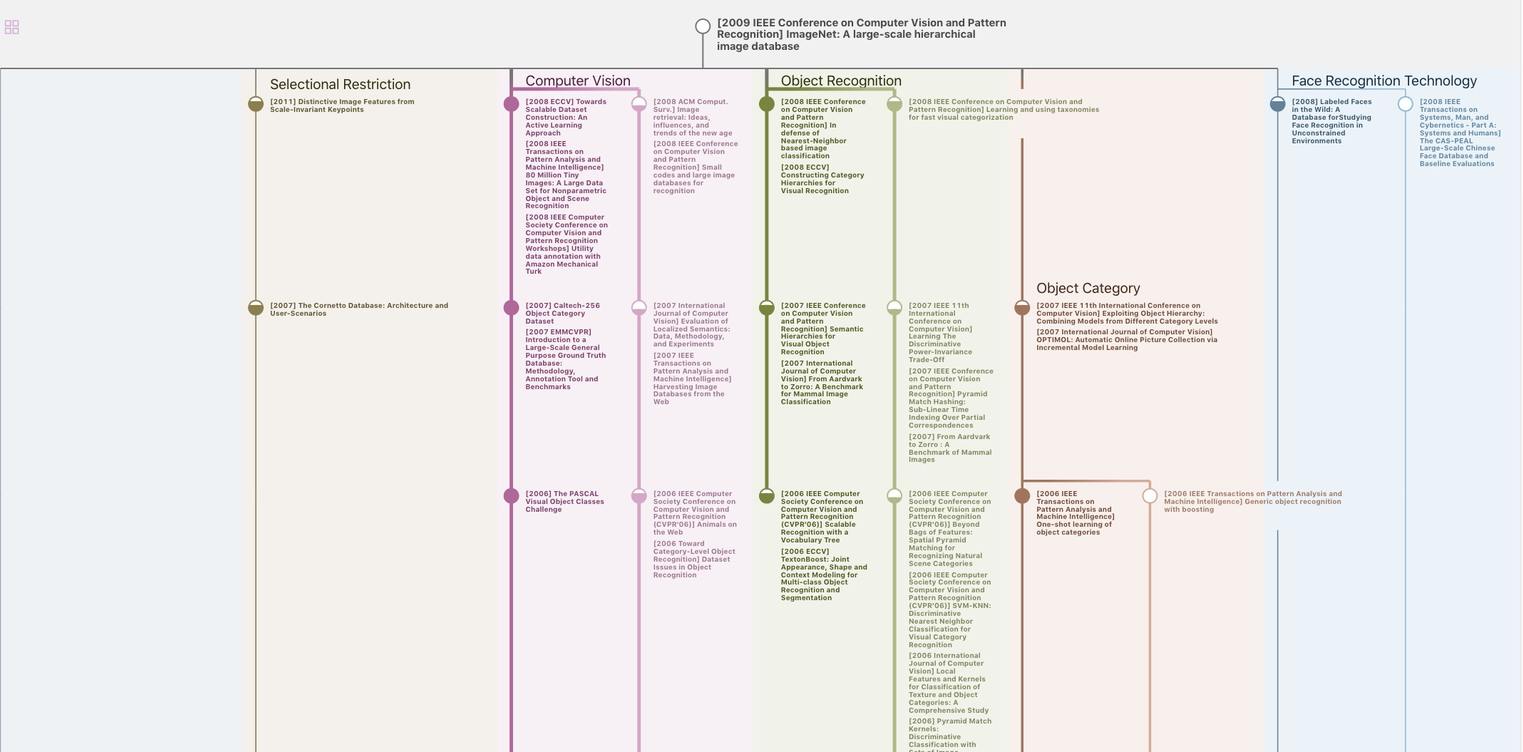
生成溯源树,研究论文发展脉络
Chat Paper
正在生成论文摘要