Trust Region-Based Safe Distributional Reinforcement Learning for Multiple Constraints.
NeurIPS 2023(2023)
摘要
In safety-critical robotic tasks, potential failures must be reduced, and multiple constraints must be met, such as avoiding collisions, limiting energy consumption, and maintaining balance. Thus, applying safe reinforcement learning (RL) in such robotic tasks requires to handle multiple constraints and use risk-averse constraints rather than risk-neutral constraints. To this end, we propose a trust region-based safe RL algorithm for multiple constraints called a safe distributional actor-critic (SDAC). Our main contributions are as follows: 1) introducing a gradient integration method to manage infeasibility issues in multi-constrained problems, ensuring theoretical convergence, and 2) developing a TD($\lambda$) target distribution to estimate risk-averse constraints with low biases. We evaluate SDAC through extensive experiments involving multi- and single-constrained robotic tasks. While maintaining high scores, SDAC shows 1.93 times fewer steps to satisfy all constraints in multi-constrained tasks and 1.78 times fewer constraint violations in single-constrained tasks compared to safe RL baselines. Code is available at: https://github.com/rllab-snu/Safe-Distributional-Actor-Critic.
更多查看译文
关键词
Reinforcement learning,Safety,Multiple Constraints,Distributional Critic
AI 理解论文
溯源树
样例
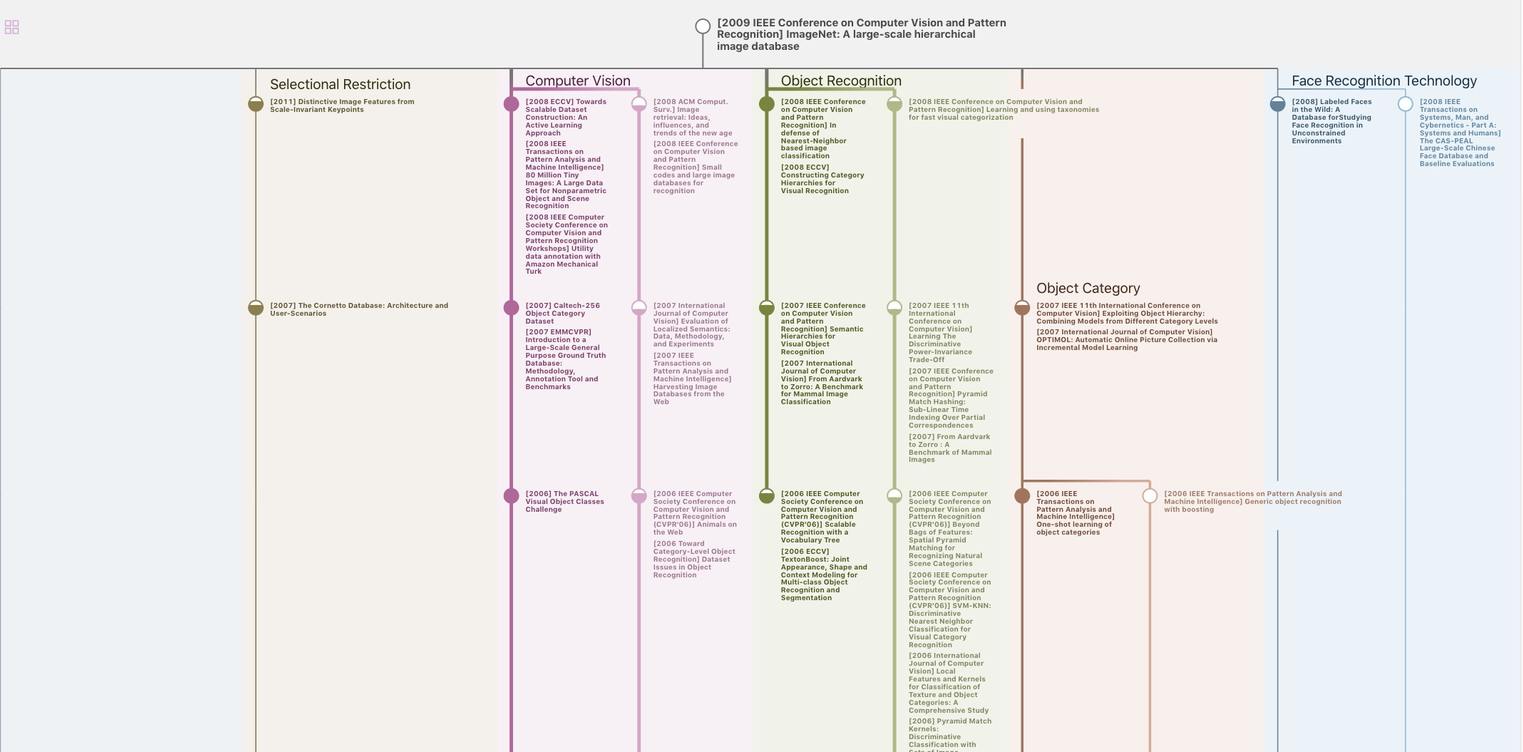
生成溯源树,研究论文发展脉络
Chat Paper
正在生成论文摘要