Effectively Learning Initiation Sets in Hierarchical Reinforcement Learning.
NeurIPS(2023)
摘要
An agent learning an option in hierarchical reinforcement learning must solve three problems: identify the option's subgoal (termination condition), learn a policy, and learn where that policy will succeed (initiation set). The termination condition is typically identified first, but the option policy and initiation set must be learned simultaneously, which is challenging because the initiation set depends on the option policy, which changes as the agent learns. Consequently, data obtained from option execution becomes invalid over time, leading to an inaccurate initiation set that subsequently harms downstream task performance. We highlight three issues---data non-stationarity, temporal credit assignment, and pessimism---specific to learning initiation sets, and propose to address them using tools from off-policy value estimation and classification. We show that our method learns higher-quality initiation sets faster than existing methods (in MiniGrid and Montezuma's Revenge), can automatically discover promising grasps for robot manipulation (in Robosuite), and improves the performance of a state-of-the-art option discovery method in a challenging maze navigation task in MuJoCo.
更多查看译文
AI 理解论文
溯源树
样例
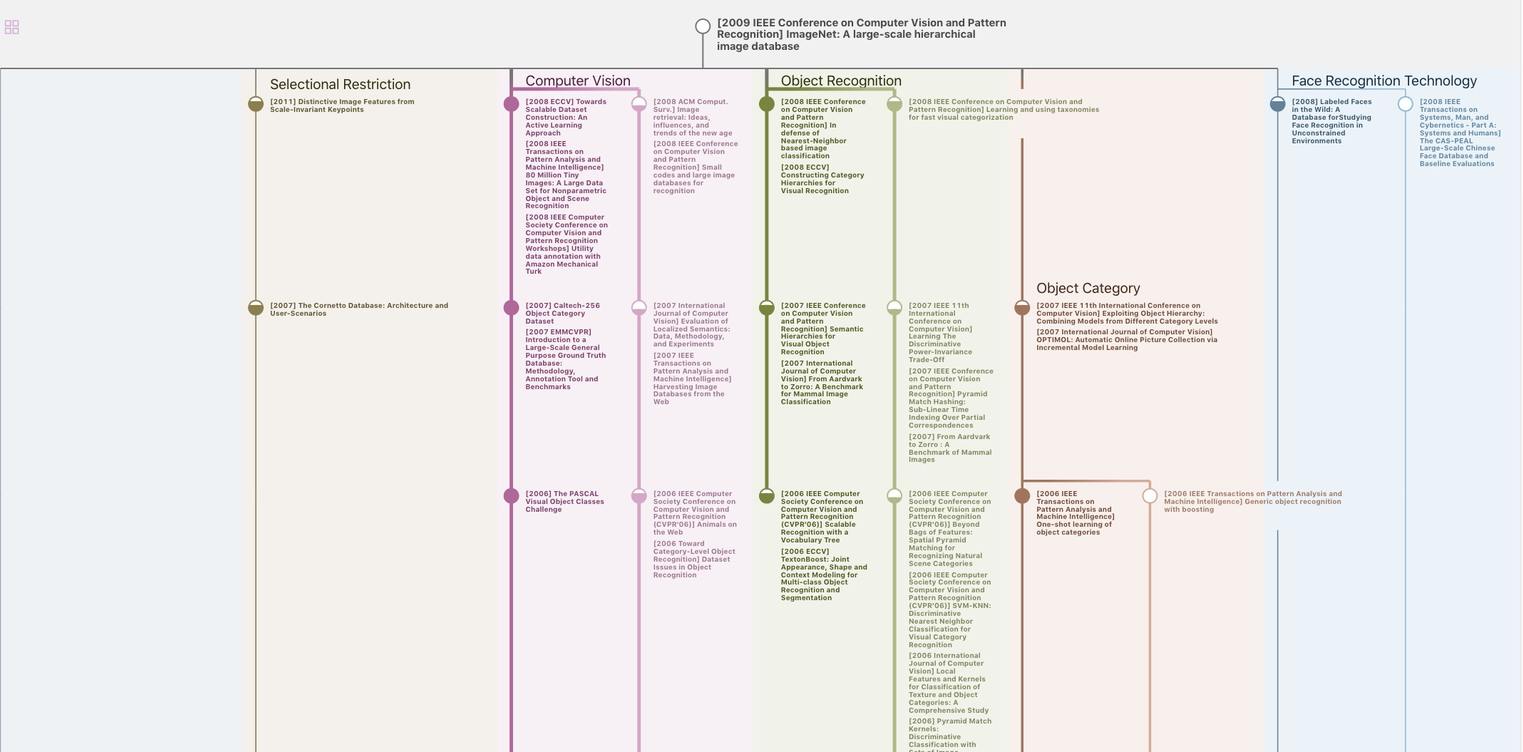
生成溯源树,研究论文发展脉络
Chat Paper
正在生成论文摘要