Rank-N-Contrast: Learning Continuous Representations for Regression.
NeurIPS(2023)
摘要
Deep regression models typically learn in an end-to-end fashion without explicitly emphasizing a regression-aware representation. Consequently, the learned representations exhibit fragmentation and fail to capture the continuous nature of sample orders, inducing suboptimal results across a wide range of regression tasks. To fill the gap, we propose Rank-N-Contrast (RNC), a framework that learns continuous representations for regression by contrasting samples against each other based on their rankings in the target space. We demonstrate, theoretically and empirically, that RNC guarantees the desired order of learned representations in accordance with the target orders, enjoying not only better performance but also significantly improved robustness, efficiency, and generalization. Extensive experiments using five real-world regression datasets that span computer vision, human-computer interaction, and healthcare verify that RNC achieves state-of-the-art performance, highlighting its intriguing properties including better data efficiency, robustness to spurious targets and data corruptions, and generalization to distribution shifts. Code is available at: https://github.com/kaiwenzha/Rank-N-Contrast.
更多查看译文
关键词
continuous representations,regression,learning,rank-n-contrast
AI 理解论文
溯源树
样例
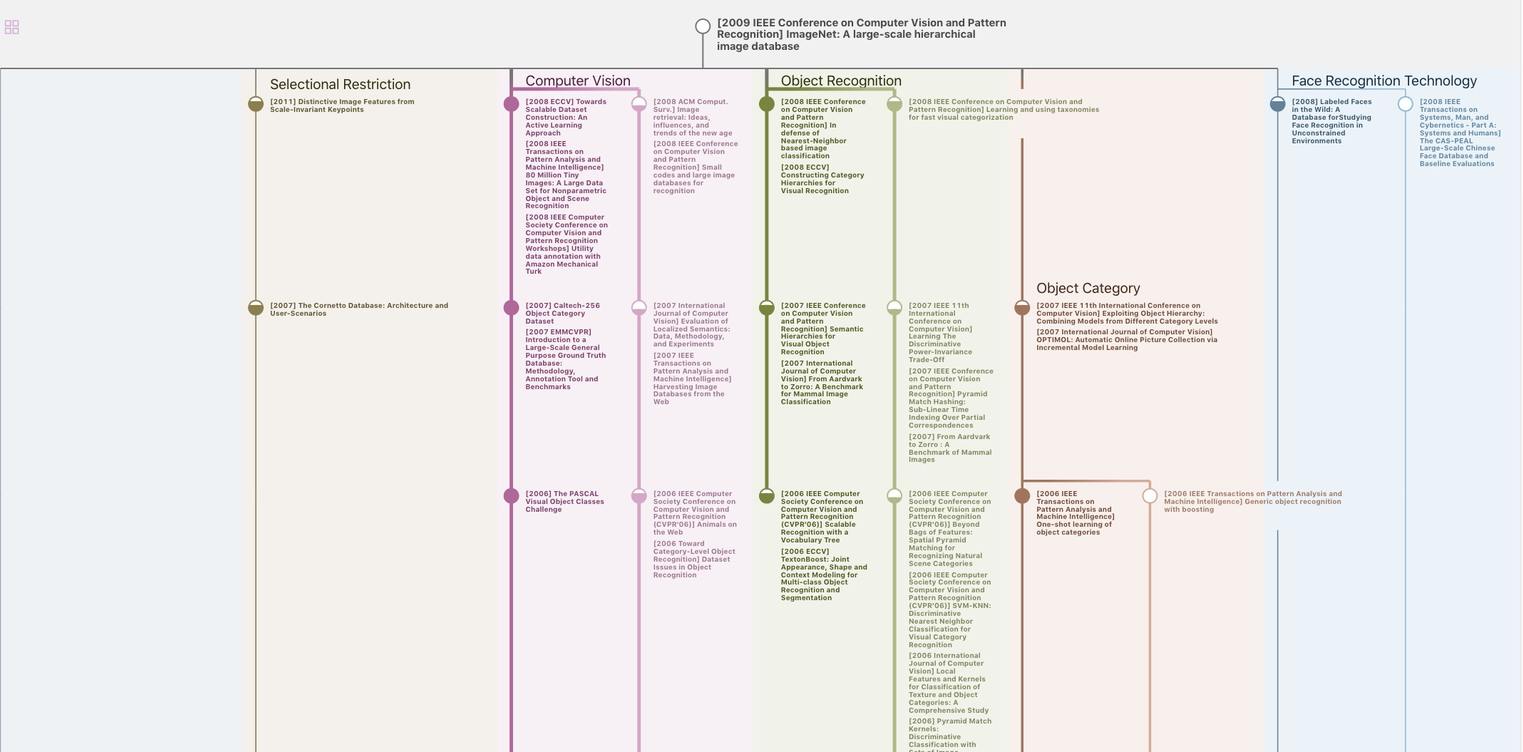
生成溯源树,研究论文发展脉络
Chat Paper
正在生成论文摘要