A rapid review exploring the effectiveness of artificial intelligence for cancer diagnosis
medRxiv (Cold Spring Harbor Laboratory)(2023)
摘要
There is growing demand for diagnostic services in the UK. This rapid review aimed to assess the effectiveness of artificial intelligence (AI) in diagnostic radiology with a focus on cancer diagnosis. A range of AI models including machine learning, deep learning and ensemble models, were assessed in this review. The review included an initial broad mapping exercise and a more in-depth synthesis of a specific sub-set of the evidence. The review included evidence available from 2018 until June 2023. A total of 92 comparative primary studies were included in the evidence map. The evidence map identified 52 studies in which the AI models were in the early stages of development and validation, and highlighted breast, lung and prostate cancers as the type of cancers most frequently reported on. 28 studies evaluating an established model and focusing on the diagnosis of breast, lung, and prostate cancer were included in the in-depth synthesis. All studies included in the in-depth synthesis were classified as diagnostic accuracy studies. Only one study evaluated an AI model that was commercially available in the UK. Most studies reported results in favour of the AI models, however, these improvements were not always statistically significant. The studies also varied considerably in terms of AI models studied, type of cancer, images used, and comparison made; and were limited in terms of their methodology. When used as a standalone diagnostic tool, there is evidence to suggest that AI can improve diagnostic accuracy or is comparable to experienced radiologists, however this may be dependent on the AI model being used. There is evidence to suggest that AI may be beneficial when used as a support tool for clinicians/radiologists with less experience. The impact of AI on the timeline involved in diagnosis appeared inconsistent. AI may speed up the diagnostic timeline when the level of cancer suspicion is low but may increase diagnostic timelines when the level of cancer suspicion is high. The evidence suggests that clinicians are accepting of AI-based assistance for cancer diagnosis. Policy and practice implications: The overall evidence for effectiveness appeared in favour of AI and several factors were identified that impact the effectiveness of the AI models. AI may improve diagnostic accuracy in clinicians/radiologists with less experience of interpreting radiological images. However, further well-designed high-quality research is needed from the UK and similar countries to better understand the effectiveness of AI in cancer diagnosis. Economic considerations: There is little evidence on the cost-effectiveness of using AI for cancer diagnosis. In theory, it might be possible for AI to assist with earlier diagnosis of cancer with both health and economic benefits.
### Competing Interest Statement
The authors have declared no competing interest.
### Funding Statement
The Public Health Wales Observatory was funded for this work by the Health and Care Research Wales Evidence Centre, itself funded by Health and Care Research Wales on behalf of Welsh Government.
### Author Declarations
I confirm all relevant ethical guidelines have been followed, and any necessary IRB and/or ethics committee approvals have been obtained.
Yes
I confirm that all necessary patient/participant consent has been obtained and the appropriate institutional forms have been archived, and that any patient/participant/sample identifiers included were not known to anyone (e.g., hospital staff, patients or participants themselves) outside the research group so cannot be used to identify individuals.
Yes
I understand that all clinical trials and any other prospective interventional studies must be registered with an ICMJE-approved registry, such as ClinicalTrials.gov. I confirm that any such study reported in the manuscript has been registered and the trial registration ID is provided (note: if posting a prospective study registered retrospectively, please provide a statement in the trial ID field explaining why the study was not registered in advance).
Yes
I have followed all appropriate research reporting guidelines, such as any relevant EQUATOR Network research reporting checklist(s) and other pertinent material, if applicable.
Yes
All data produced in the present study are available upon reasonable request to the authors
更多查看译文
关键词
cancer diagnosis,artificial intelligence
AI 理解论文
溯源树
样例
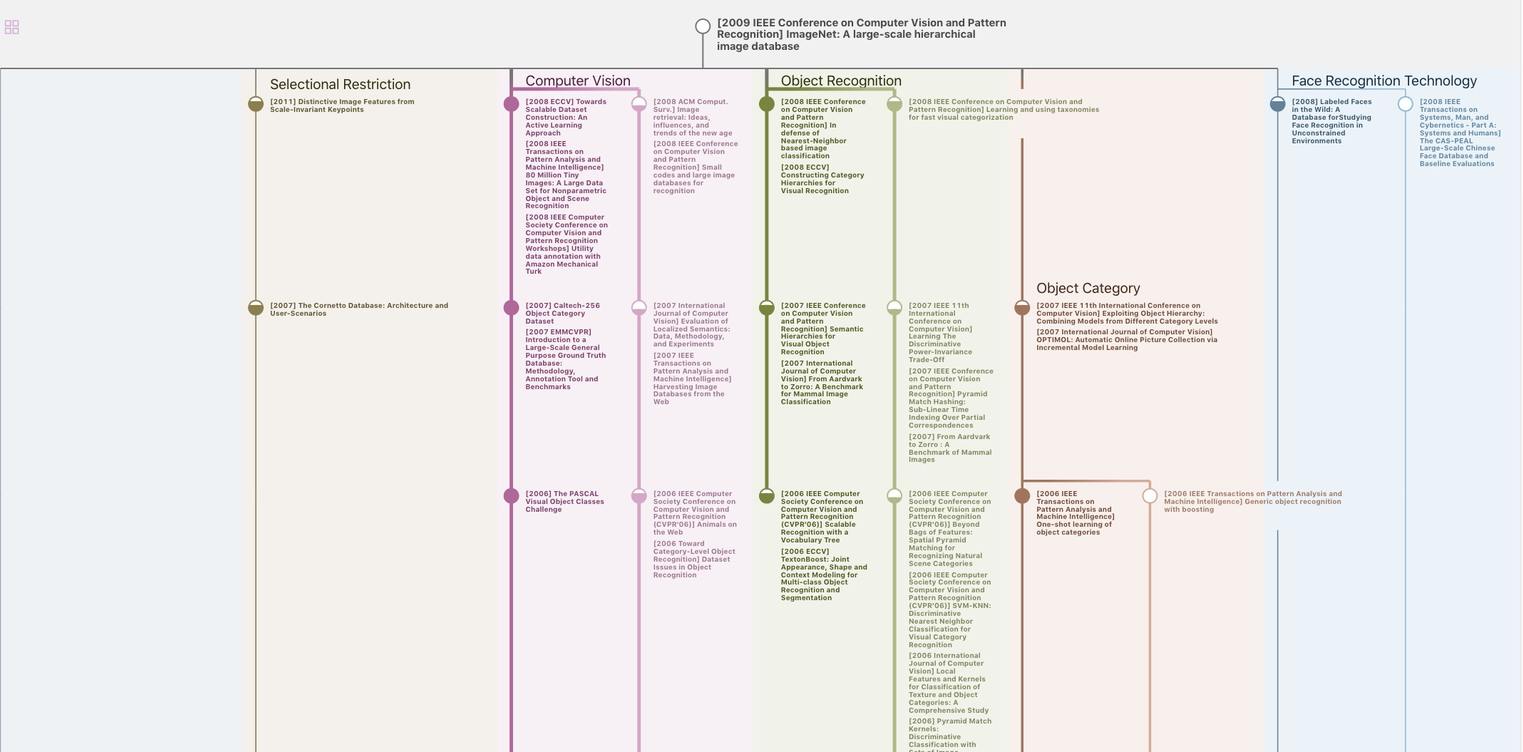
生成溯源树,研究论文发展脉络
Chat Paper
正在生成论文摘要