A Framework to Assess (Dis)agreement Among Diverse Rater Groups.
CoRR(2023)
摘要
Recent advancements in conversational AI have created an urgent need for safety guardrails that prevent users from being exposed to offensive and dangerous content. Much of this work relies on human ratings and feedback, but does not account for the fact that perceptions of offense and safety are inherently subjective and that there may be systematic disagreements between raters that align with their socio-demographic identities. Instead, current machine learning approaches largely ignore rater subjectivity and use gold standards that obscure disagreements (e.g., through majority voting). In order to better understand the socio-cultural leanings of such tasks, we propose a comprehensive disagreement analysis framework to measure systematic diversity in perspectives among different rater subgroups. We then demonstrate its utility by applying this framework to a dataset of human-chatbot conversations rated by a demographically diverse pool of raters. Our analysis reveals specific rater groups that have more diverse perspectives than the rest, and informs demographic axes that are crucial to consider for safety annotations.
更多查看译文
关键词
diverse rater groups,assess
AI 理解论文
溯源树
样例
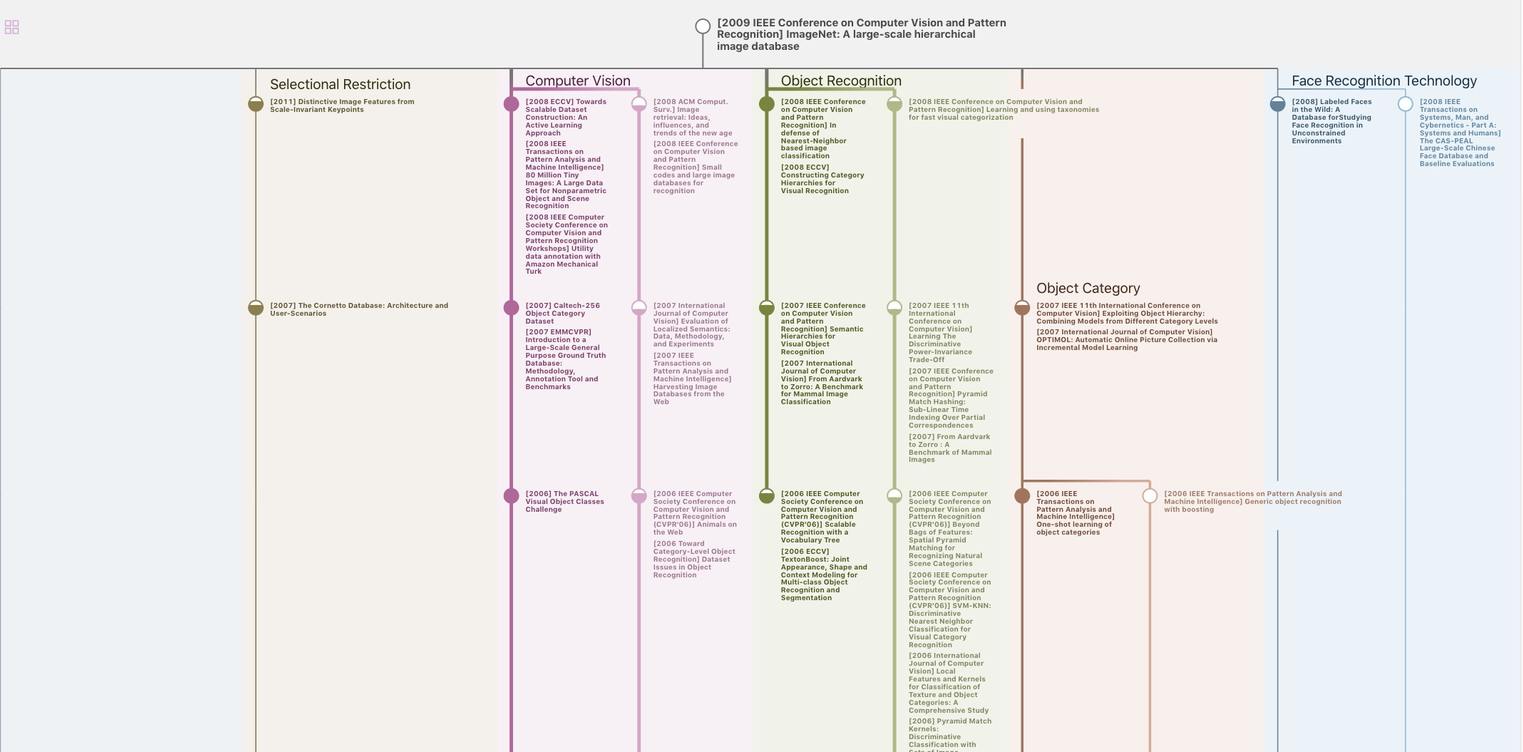
生成溯源树,研究论文发展脉络
Chat Paper
正在生成论文摘要