Mental Health Diagnosis in the Digital Age: Harnessing Sentiment Analysis on Social Media Platforms upon Ultra-Sparse Feature Content.
CoRR(2023)
摘要
Amid growing global mental health concerns, particularly among vulnerable groups, natural language processing offers a tremendous potential for early detection and intervention of people's mental disorders via analyzing their postings and discussions on social media platforms. However, ultra-sparse training data, often due to vast vocabularies and low-frequency words, hinders the analysis accuracy. Multi-labeling and Co-occurrences of symptoms may also blur the boundaries in distinguishing similar/co-related disorders. To address these issues, we propose a novel semantic feature preprocessing technique with a three-folded structure: 1) mitigating the feature sparsity with a weak classifier, 2) adaptive feature dimension with modulus loops, and 3) deep-mining and extending features among the contexts. With enhanced semantic features, we train a machine learning model to predict and classify mental disorders. We utilize the Reddit Mental Health Dataset 2022 to examine conditions such as Anxiety, Borderline Personality Disorder (BPD), and Bipolar-Disorder (BD) and present solutions to the data sparsity challenge, highlighted by 99.81% non-zero elements. After applying our preprocessing technique, the feature sparsity decreases to 85.4%. Overall, our methods, when compared to seven benchmark models, demonstrate significant performance improvements: 8.0% in accuracy, 0.069 in precision, 0.093 in recall, 0.102 in F1 score, and 0.059 in AUC. This research provides foundational insights for mental health prediction and monitoring, providing innovative solutions to navigate challenges associated with ultra-sparse data feature and intricate multi-label classification in the domain of mental health analysis.
更多查看译文
关键词
harnessing sentiment
AI 理解论文
溯源树
样例
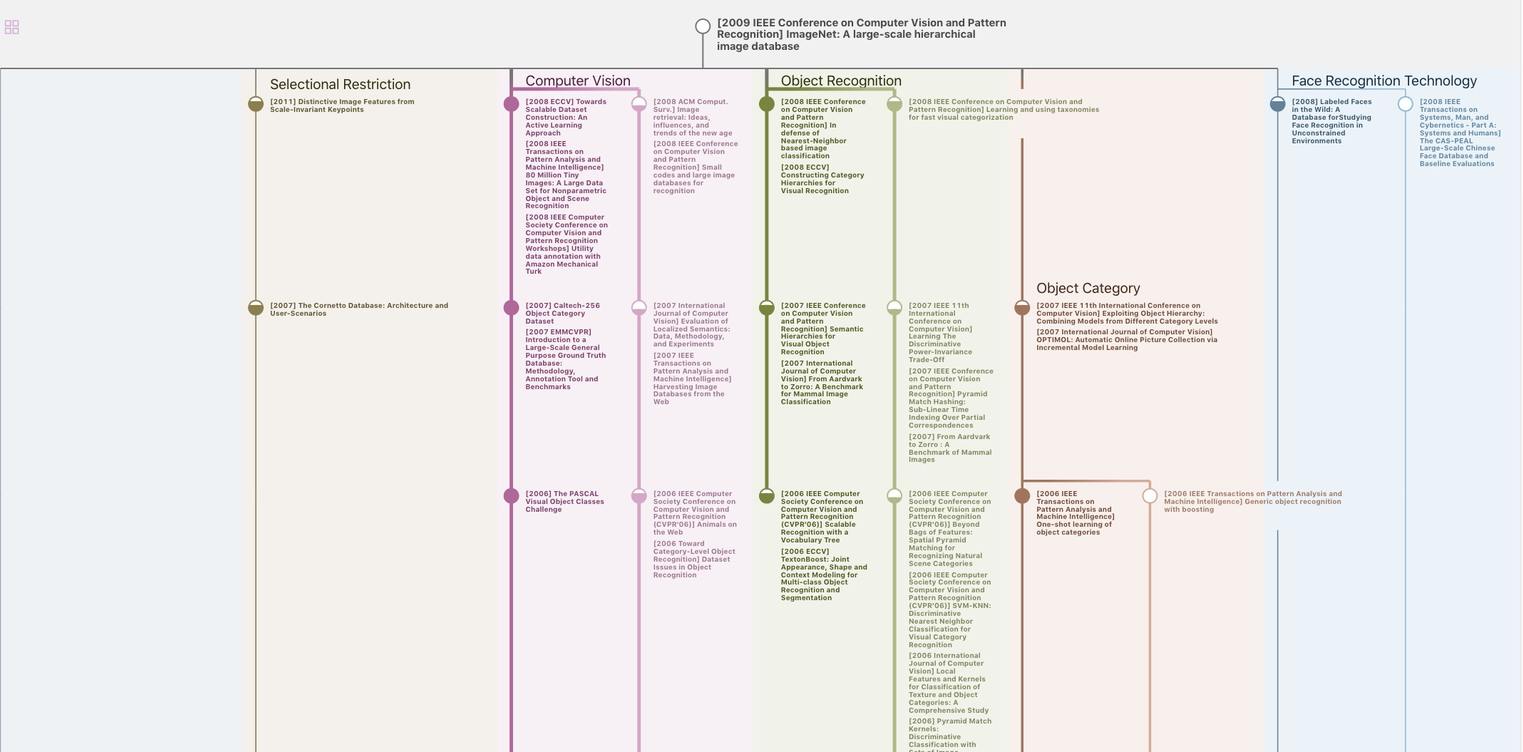
生成溯源树,研究论文发展脉络
Chat Paper
正在生成论文摘要