Accelerated Shapley Value Approximation for Data Evaluation.
CoRR(2023)
摘要
Data valuation has found various applications in machine learning, such as data filtering, efficient learning and incentives for data sharing. The most popular current approach to data valuation is the Shapley value. While popular for its various applications, Shapley value is computationally expensive even to approximate, as it requires repeated iterations of training models on different subsets of data. In this paper we show that the Shapley value of data points can be approximated more efficiently by leveraging the structural properties of machine learning problems. We derive convergence guarantees on the accuracy of the approximate Shapley value for different learning settings including Stochastic Gradient Descent with convex and non-convex loss functions. Our analysis suggests that in fact models trained on small subsets are more important in the context of data valuation. Based on this idea, we describe $\delta$-Shapley -- a strategy of only using small subsets for the approximation. Experiments show that this approach preserves approximate value and rank of data, while achieving speedup of up to 9.9x. In pre-trained networks the approach is found to bring more efficiency in terms of accurate evaluation using small subsets.
更多查看译文
关键词
approximation,data,evaluation,value
AI 理解论文
溯源树
样例
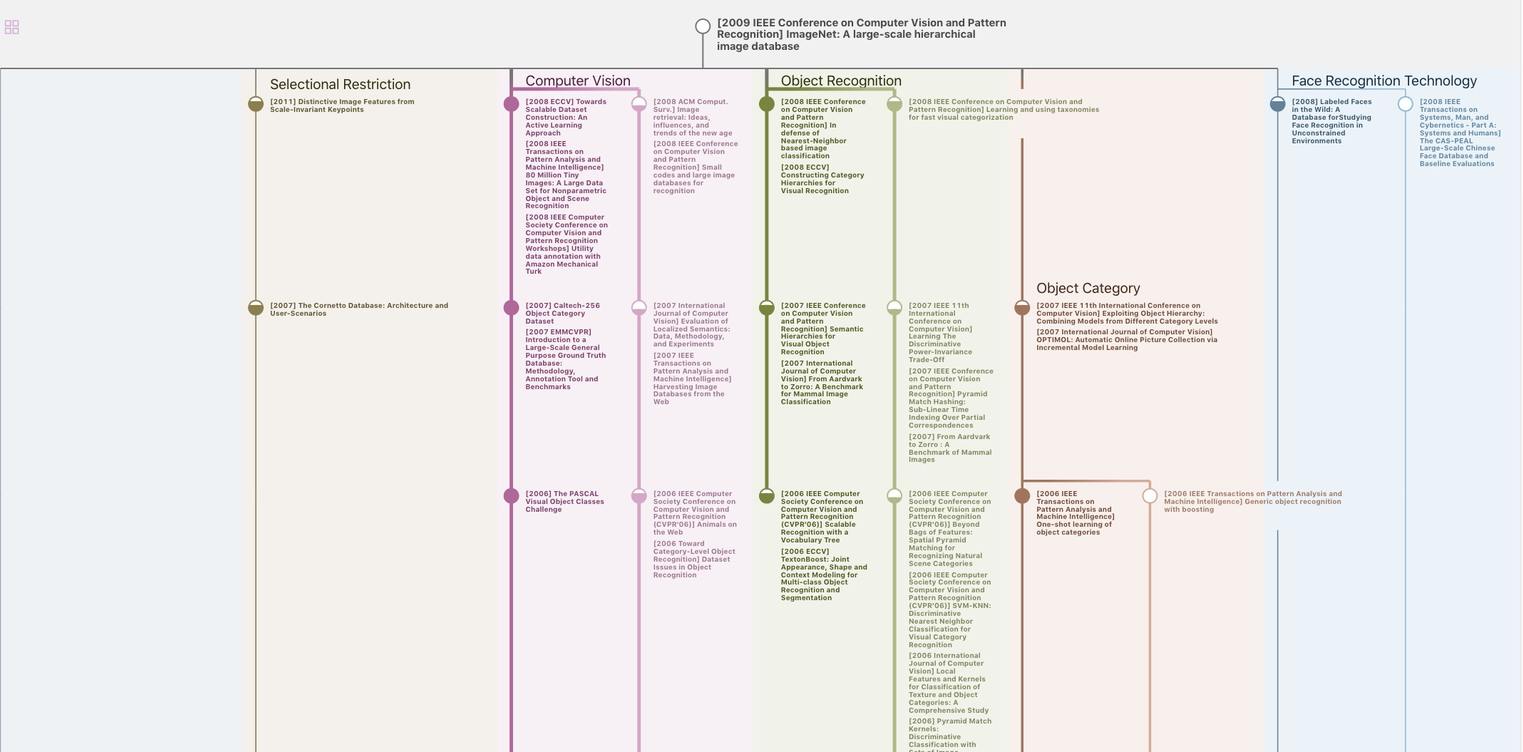
生成溯源树,研究论文发展脉络
Chat Paper
正在生成论文摘要