Beyond the training set: an intuitive method for detecting distribution shift in model-based optimization.
CoRR(2023)
摘要
Model-based optimization (MBO) is increasingly applied to design problems in science and engineering. A common scenario involves using a fixed training set to train models, with the goal of designing new samples that outperform those present in the training data. A major challenge in this setting is distribution shift, where the distributions of training and design samples are different. While some shift is expected, as the goal is to create better designs, this change can negatively affect model accuracy and subsequently, design quality. Despite the widespread nature of this problem, addressing it demands deep domain knowledge and artful application. To tackle this issue, we propose a straightforward method for design practitioners that detects distribution shifts. This method trains a binary classifier using knowledge of the unlabeled design distribution to separate the training data from the design data. The classifier's logit scores are then used as a proxy measure of distribution shift. We validate our method in a real-world application by running offline MBO and evaluate the effect of distribution shift on design quality. We find that the intensity of the shift in the design distribution varies based on the number of steps taken by the optimization algorithm, and our simple approach can identify these shifts. This enables users to constrain their search to regions where the model's predictions are reliable, thereby increasing the quality of designs.
更多查看译文
关键词
optimization,intuitive method,shift,training,model-based
AI 理解论文
溯源树
样例
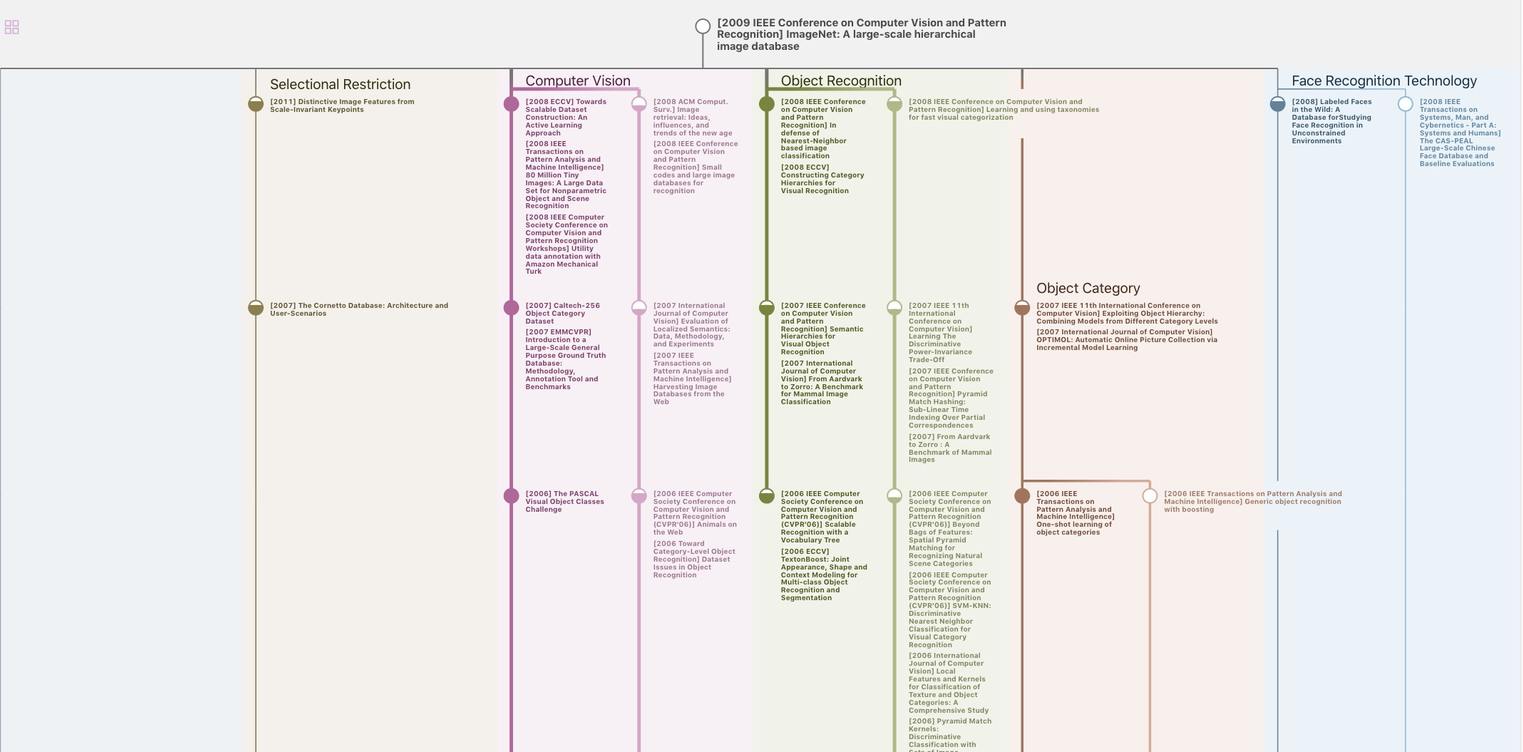
生成溯源树,研究论文发展脉络
Chat Paper
正在生成论文摘要