A holistic human activity recognition optimisation using AI techniques
IET RADAR SONAR AND NAVIGATION(2024)
摘要
Building on previous radar-based human activity recognition (HAR), we expand the micro-Doppler signature to 6 domains and exploit each domain with a set of handcrafted features derived from the literature and our patents. An adaptive thresholding method to isolate the region of interest is employed, which is then applied in other domains. To reduce the computational burden and accelerate the convergence to an optimal solution for classification accuracy, a holistic approach to HAR optimisation is proposed using a surrogate model-assisted differential evolutionary algorithm (SADEA-I) to jointly optimise signal processing, adaptive thresholding and classification parameters for HAR. Two distinct classification models are evaluated with holistic optimisation: SADEA-I with support vector machine classifiers (SVM) and SADEA-I with AlexNet. They achieve an accuracy of 89.41% and 93.54%, respectively. This is an improvement of & SIM;11.3% for SVM and & SIM;2.7% for AlexNet when compared to the performance without SADEA-I. The effectiveness of our holistic approach is validated using the University of Glasgow human radar signatures dataset. This proof of concept is significant for dimensionality reduction and computational efficiency when facing a multiplication of radar representation domains/feature spaces and transmitting/receiving channels that could be individually tuned in modern radar systems. This study enhances radar-based human activity recognition by expanding the micro-Doppler signature (MDS) to six domains, each exploited with a patented set of handcrafted features. We also introduce a novel holistic optimisation approach using the surrogate model-assisted differential evolutionary algorithm (SADEA-I), which jointly optimises signal processing, adaptive thresholding, and classification parameters, significantly reducing computational burden and improving classification accuracy. Our optimisation approach proves crucial for efficient dimensionality reduction and computational performance in the context of modern radar systems with diverse representation domains and tunable transmitting/receiving channels.image
更多查看译文
关键词
evolutionary computation,pattern classification,radar,radar signal processing
AI 理解论文
溯源树
样例
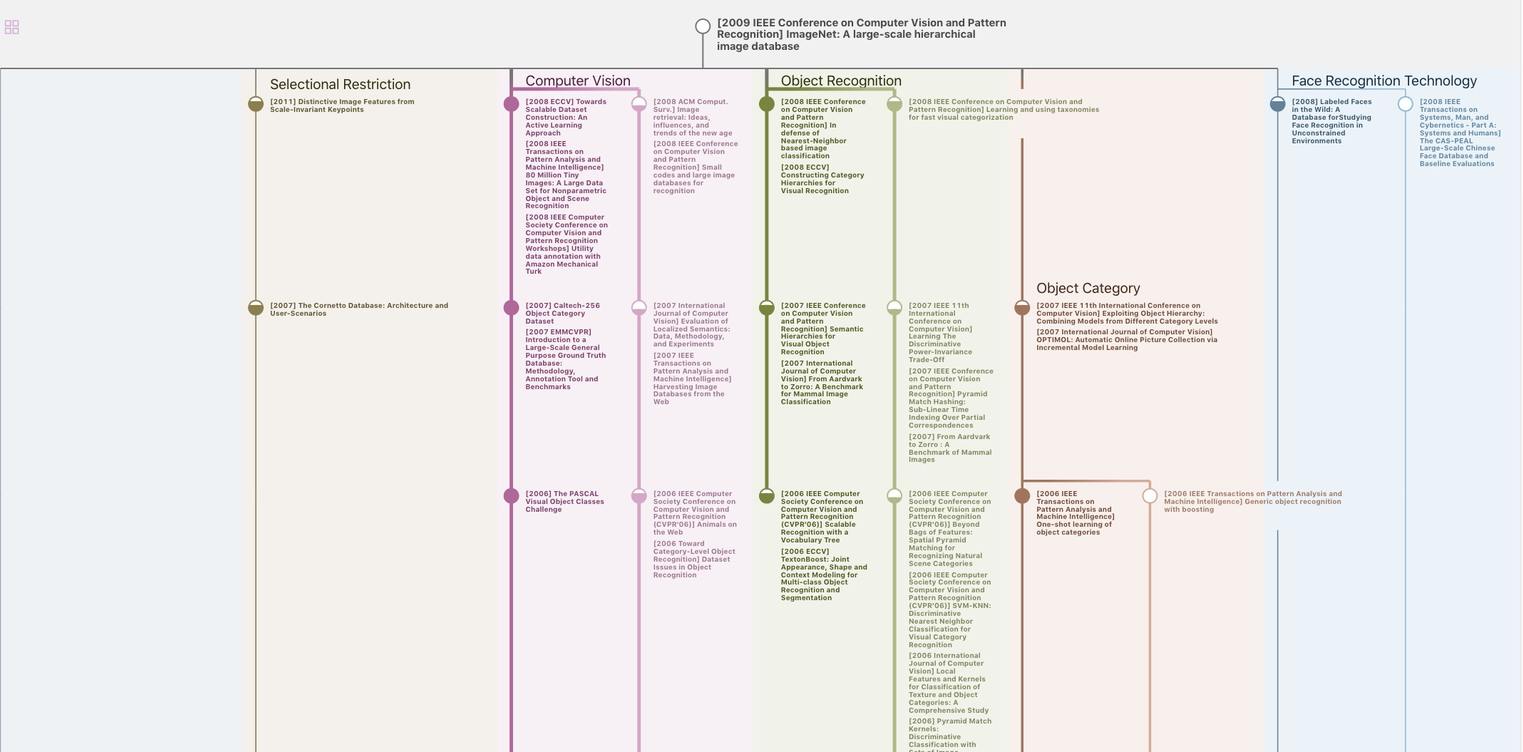
生成溯源树,研究论文发展脉络
Chat Paper
正在生成论文摘要