Automated and Interpretable Deep Learning For Carotid Plaque Analysis Using Ultrasound
JOURNAL OF VASCULAR SURGERY(2023)
摘要
The aim of this study was to develop an automated and interpretable deep learning method to classify symptomatic vs asymptomatic carotid plaques based on carotid ultrasound imaging. A total of 128 B-mode longitudinal carotid ultrasounds were included from patients with moderate to severe carotid disease; 68 from asymptomatic patients and 60 from symptomatic patients. Ultrasounds were divided into training (70%) and testing (30%) subsets. Using training ultrasounds, a convolutional neural network (CNN) was trained to automatically learn and extract deep features for classifying symptom status. High-risk regions in ultrasounds were identified using a new deep learning interpretability method. Radiomic features, including grayscale and texture descriptors, were extracted from these high-risk regions in ultrasounds. Neural networks were trained to predict symptom status using deep features, radiomic features, and combined deep and radiomic features. The importance and effect of individual radiomic features were analyzed using Shapley Additive Explanations (SHAP). The performance of each neural network was evaluated on the test ultrasounds using receiver operating characteristic area under the curve (AUC), sensitivity, and specificity. SHAP analysis of radiomic features showed that higher grayscale variation, lower texture heterogeneity, and higher grayscale variation between “zones” of homogenous pixels within plaque regions increased risk of symptomatic status. Using the test ultrasounds, performance of the neural network using combined deep and radiomic features (Fig 1) was better (AUC, 0.70; sensitivity, 0.75; specificity, 0.75) than using either deep features (AUC, 0.64; sensitivity, 0.70; specificity, 0.65) or radiomic features (AUC, 0.54; sensitivity, 0.50; specificity, 0.60) alone. High-risk plaque regions in sample test ultrasounds could be visualized (Fig 2). An automated deep learning method classified carotid ultrasounds by symptom status of patients with carotid disease. Model interpretability enabled visualization of high-risk regions and description of high-risk ultrasound features, including grayscale variation and texture homogeneity. Using this data-driven method, we can potentially find new imaging markers and predict vulnerable plaques to prevent stroke in patients.Fig 2Sample b-mode ultrasound (left) and associated heatmap illustrating automatically identified high-risk regions (right).View Large Image Figure ViewerDownload Hi-res image Download (PPT)
更多查看译文
关键词
carotid plaque analysis,interpretable deep learning,deep learning,ultrasound
AI 理解论文
溯源树
样例
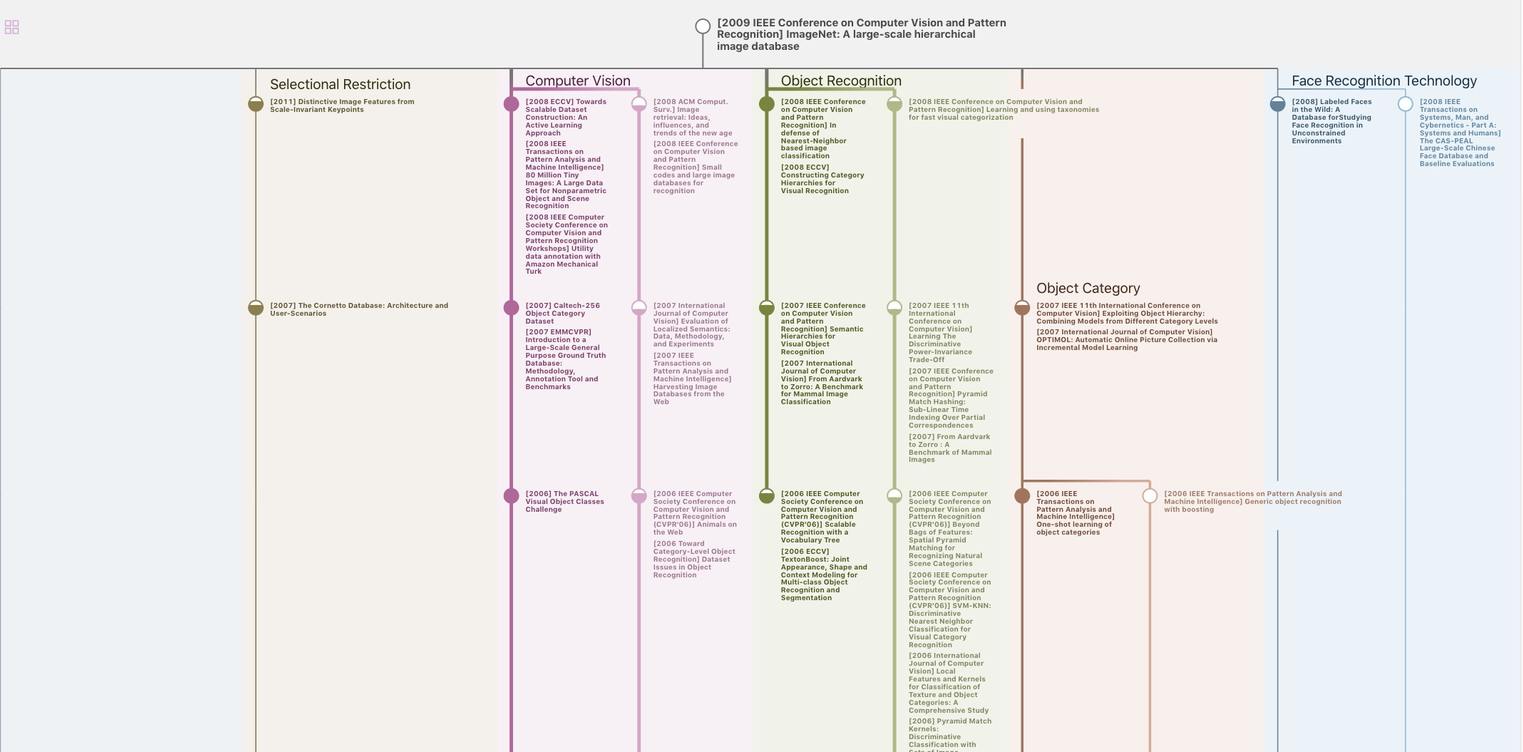
生成溯源树,研究论文发展脉络
Chat Paper
正在生成论文摘要