Ensemble learning to predict solar-to-hydrogen energy conversion based on photocatalytic water splitting over doped TiO2
GREEN CHEMISTRY(2023)
摘要
The hydrogen production rate of TiO2 photocatalytic water splitting is significantly influenced by various doped elements and experimental conditions (i.e., dopant/Ti mole ratio, calcination temperature, and calcination time). A more systematic approach is essential to effectively extract valuable knowledge from large amounts of complex and mismatched data. Herein, we demonstrate a regression fusion model for predicting the hydrogen production rate via a machine learning methodology. In this work, a database of TiO2 photocatalytic water splitting is constructed using simple descriptive features and the stacking method is used to integrate the fusion of LightGBM, XGBoost and random forest to improve the prediction ability of the machine learning model. Cross-validation is used to train the dataset to avoid the randomness of one-time assignments and improve the generalization ability of the model. The stacking model obtains the highest R-squared score and the lowest error. The impact of each factor on the hydrogen production rate is determined using the LightGBM and random forest models. Visualization of the decision tree is used to heuristically obtain high-yield hydrogen properties with an accuracy of 81.7%.
更多查看译文
关键词
photocatalytic water splitting,ensemble learning,solar-to-hydrogen
AI 理解论文
溯源树
样例
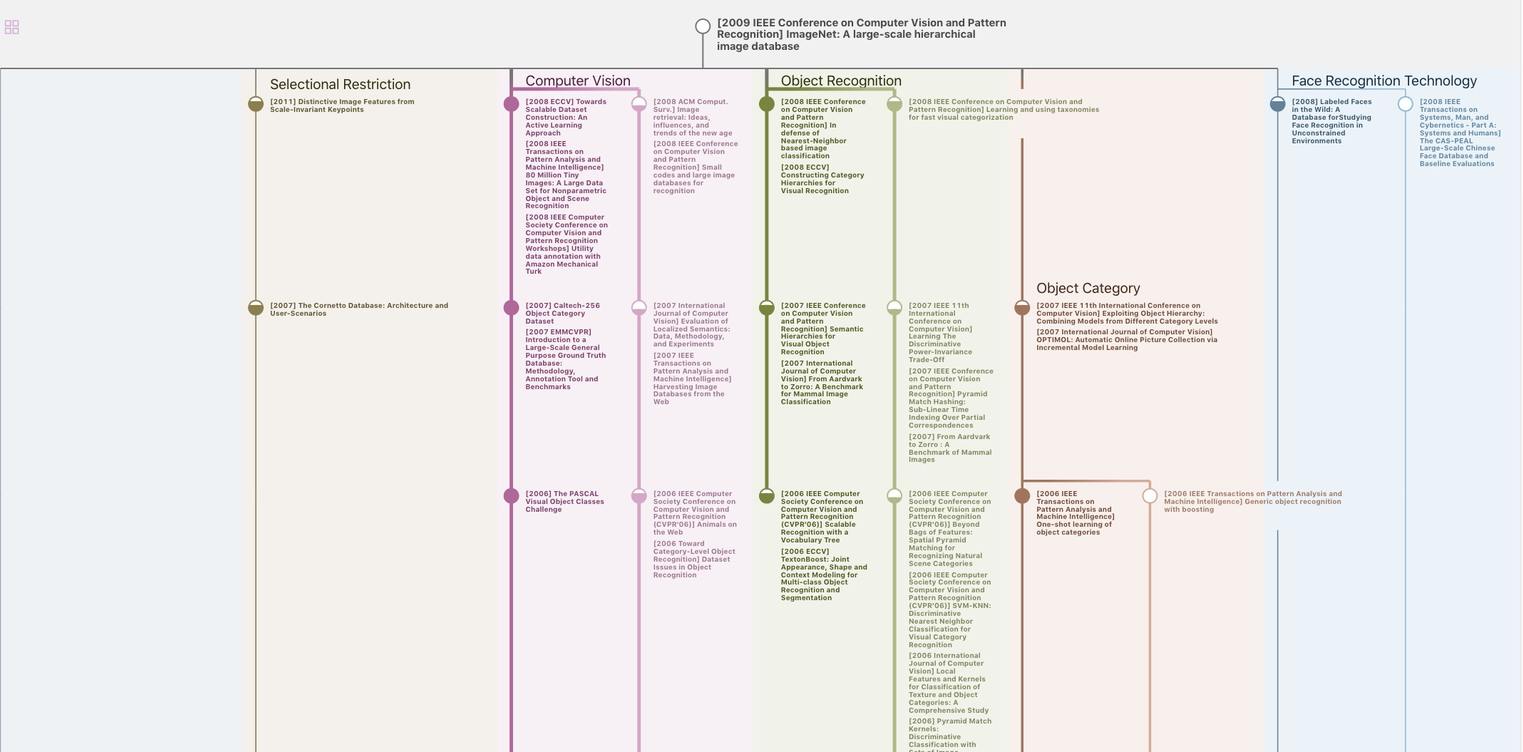
生成溯源树,研究论文发展脉络
Chat Paper
正在生成论文摘要