Deep-Learning-Based Group Point-Wise Spatial Mapping of Structure to Function in Glaucoma
INVESTIGATIVE OPHTHALMOLOGY & VISUAL SCIENCE(2023)
摘要
Purpose
To establish generalizable point-wise spatial relationship between structure and function through occlusion analysis of a deep-learning (DL) model for predicting the visual field (VF) sensitivities from three-dimensional (3D) optical coherence tomography (OCT) scan.
Design
Retrospective cross-sectional study.
Participants
2151 eyes from 1129 patients.
Methods
A DL model was trained to predict 52 VF sensitivities of 24-2 standard automated perimetry from 3D spectral-domain OCT images of the optic nerve head (ONH) with 12915 OCT-VF pairs. Using occlusion analysis, the contribution of each individual cube covering a 240 x 240 x 31.25 μm region of the ONH to the model's prediction was systematically evaluated for each OCT-VF pair in a separate test set that consisted of 996 OCT-VF pairs. After simple translation (shifting in x and y-axes to match the ONH center), group t-statistic maps were derived to visualize statistically significant ONH regions for each VF test point within a group. This analysis allowed for understanding the importance of each super voxel (240 x 240 x 31.25 μm covering the entire 4.32 x 4.32 x 1.125 mm ONH cube) in predicting VF test points for specific patient groups.
Main Outcome Measures
The region at the ONH corresponding to each VF test point and the effect of the former on the latter.
Results
The test set was divided to two groups, the healthy-to-early-glaucoma group (792 OCT-VF pairs, VF mean deviation (MD): -1.32 ± 1.90 dB) and the moderate-to-advanced-glaucoma group (204 OCT-VF pairs, VF MD: -17.93 ± 7.68 dB). Two-dimensional group t-statistic maps (x, y projection) were generated for both groups, assigning related ONH regions to visual field test points. The identified influential structural locations for VF sensitivity prediction at each test point aligned well with existing knowledge and understanding of structure-function spatial relationships.
Conclusions
This study successfully visualized the global trend of point-by-point spatial relationships between OCT-based structure and VF-based function without the need for prior knowledge or segmentation of OCTs. The revealed spatial correlations were consistent with previously published mappings. This presents possibilities of learning from trained machine learning models without applying any prior knowledge, potentially robust and free from bias.
更多查看译文
关键词
structure-to-function mapping,deep learning,structure-function relationship,OCT,VF,glaucoma
AI 理解论文
溯源树
样例
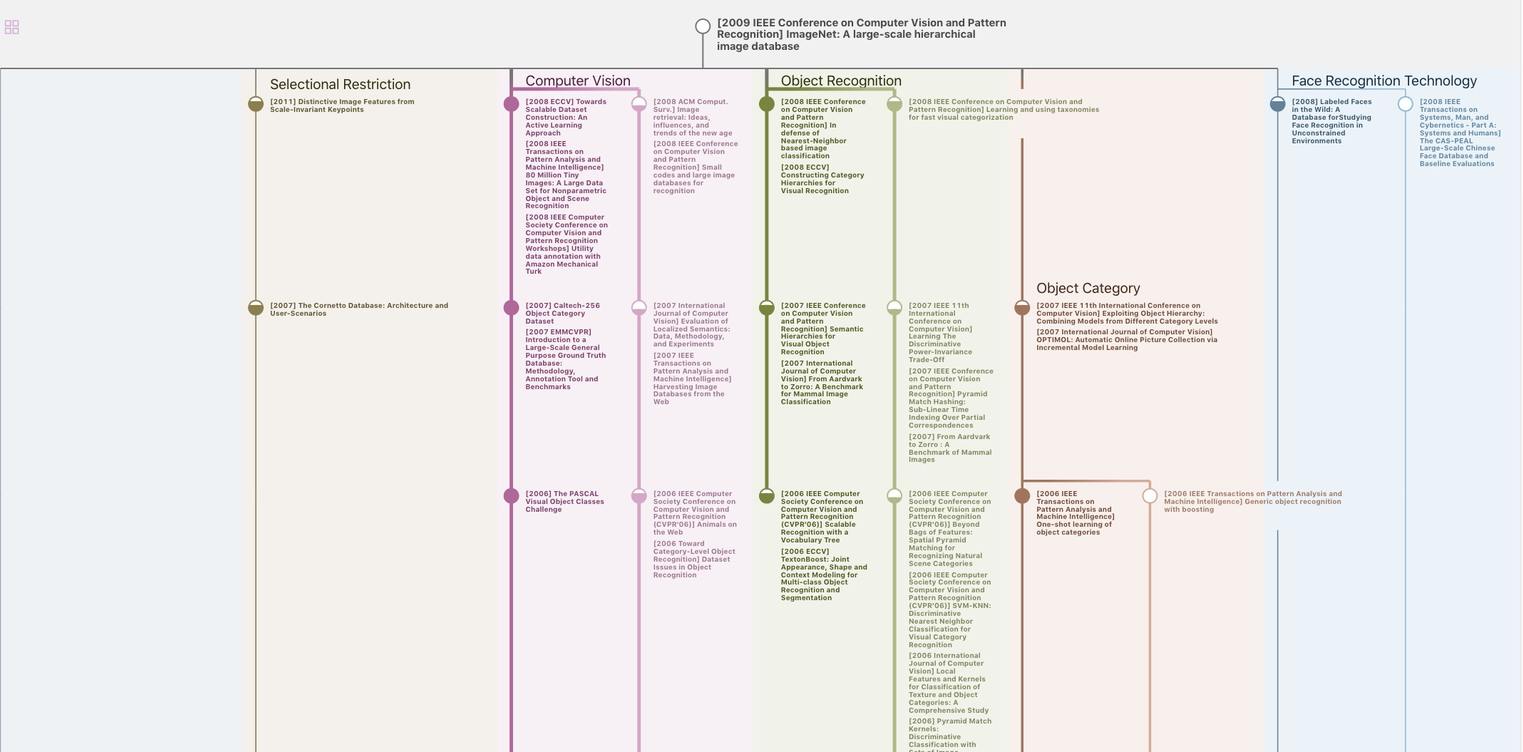
生成溯源树,研究论文发展脉络
Chat Paper
正在生成论文摘要