Construction of a High Spatiotemporal Resolution Dataset of Satellite-Derived Pco2 and Air–Sea CO2 Flux in the South China Sea (2003–2019)
IEEE transactions on geoscience and remote sensing(2023)
摘要
The South China Sea (SCS) is one of the largest marginal seas in the world. It includes a river-dominated, highly productive ocean margin on the northern shelf and an oligotrophic ocean-dominated basin along with other subregions with various features. It was a challenge to estimate the air-sea CO2 flux in this area. We developed a retrieval algorithm for sea surface partial pressure of CO2 (pCO(2)) by a combination of our previously established mechanistic semianalytical method (MeSAA) and machine learning (ML) method, named MeSAA-ML-SCS, built upon a large dataset of sea surface pCO(2) collected from in situ measurements during 44 cruises /legs to the SCS in the last two decades. We set several semianalytical parameters, including pCO(2_therm) represented the combined effect of thermodynamics and the atmospheric CO2 forcing on seawater pCO(2); upwelling index (UISST) and mixing layer depth (MLD) to characterize the mixing processes; and chlorophyll-a concentration (Chl-a) with remote sensing reflectance at 443 and 555 nm [Rrs(443) and Rrs(555)], which were proxies of biological effects and other characteristics for distinguishing shelf, basin, and subregions. We set the difference between seawater pCO(2) and atmospheric pCO(2)(Delta pCO(2)(Sea-Air)) as the output, and the seawater pCO(2) was finally obtained by summing atmospheric pCO(2) and Delta pCO(2)(Sea-Air). We compared several ML models, and the XGBoost model was confirmed as the best. Independent cruisebased datasets that are not involved in the model training were used to validate the satellite products, with low root-mean-square error (RMSE = 11.69 mu atm) and mean absolute percentage deviation (APD = 1.59%). The increasing trend of time-series satellite-derived pCO(2) (2.44 +/- 0.24 mu atm/year) was validated by the in situ data at the Southeastern Asia Time-series Study (SEATS) station, showing good consistency. Results indicate that
更多查看译文
关键词
Air-sea CO2 flux,machine learning (ML),satellite retrieval,seawater p CO2,semianalytical algorithm,South China Sea (SCS)
AI 理解论文
溯源树
样例
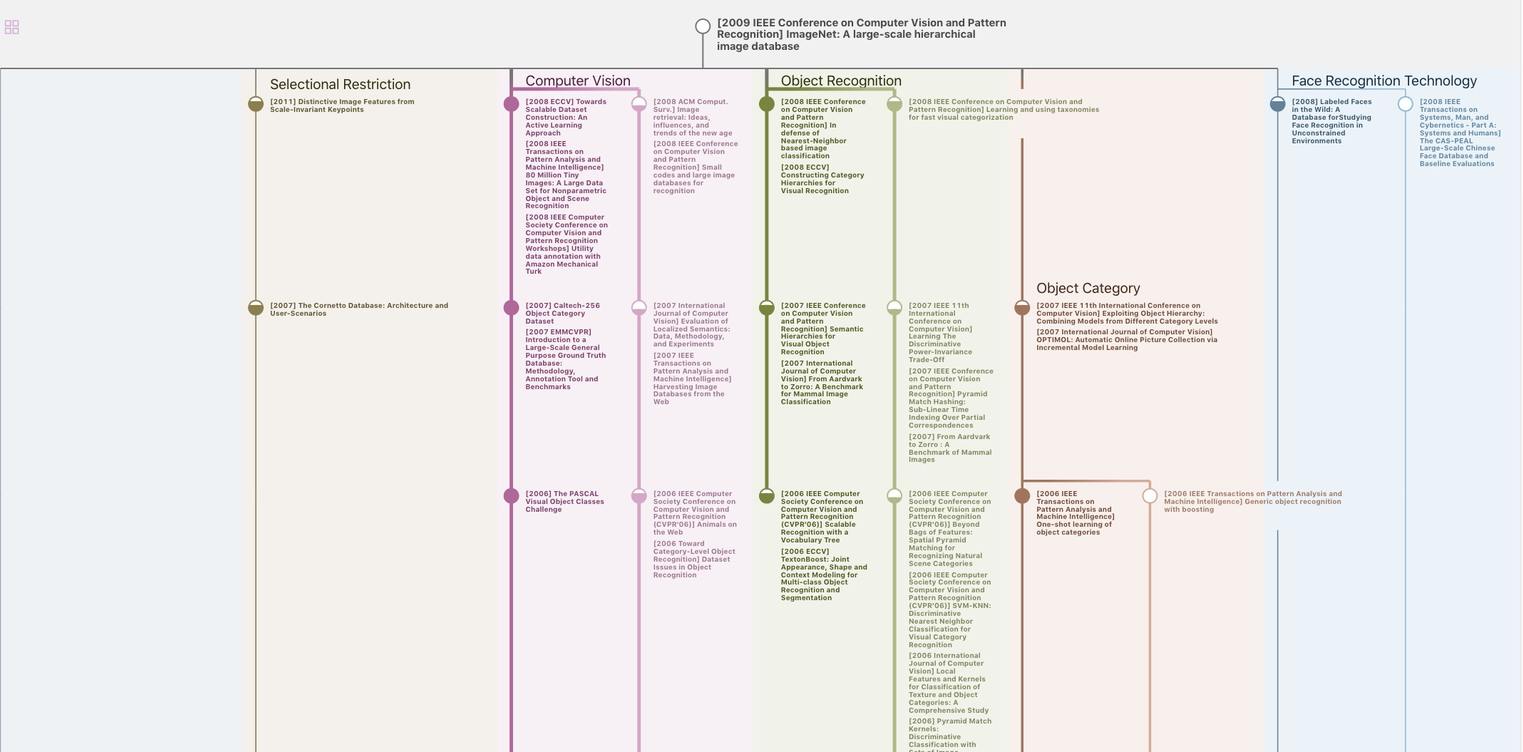
生成溯源树,研究论文发展脉络
Chat Paper
正在生成论文摘要