A two-layer integrated model for cyclist trajectory prediction considering multiple interactions with the environment
TRANSPORTATION RESEARCH PART C-EMERGING TECHNOLOGIES(2023)
摘要
Interacting with complex environments is a major challenge for autonomous vehicles (AVs), especially interacting with bicycles, because bicycle trajectories are difficult to predict. Bicycles typically interact with multiple traffic participants simultaneously, resulting in diverse and random trajectories, even sudden-change behavior. Sudden-change behavior of bicycles, which is hazardous for AVs but with sparse data, cannot be well learned by current deep-learning models due to the characteristics of "learning average behavior". Furthermore, the current domain knowledge of explicit trajectory laws integrated into the deep learning modes cannot be used to describe the implicit decision-making of cyclists which is a major factor affecting cyclists' sudden change behavior. Based on these observations, this paper develops a two-layer integrated framework for bicycle trajectory prediction. The trajectory generation layer is used to provide a kinematically feasible set of candidate trajectories, and then the trajectory selection layer is performed to select a reasonable trajectory by analyzing the cyclists' decision-making. In the trajectory generation layer, the kinematic laws are first used to determine a feasible set of endpoints, and then deep learning is utilized to learn and generate each complete trajectory under the known start and endpoints. In the trajectory selection layer, a utility-based model is proposed to analyze the decision-making for cyclists, which is defined as the cyclist's tradeoff between path efficiency and risk in the multiple interactions of the environment. Finally, the candidate with the maximum utility serves as the predicted trajectory. The reasonableness of the proposed model has been verified using the bicycle trajectory data from an intersection in Shanghai, China. Compared with the state-of-the-art models, the integrated model not only exhibits significantly lower errors but also fewer long-tail samples for the prediction error. In addition, it demonstrates a higher validity of predicting cycling decisions during sudden changes in behaviors.
更多查看译文
关键词
Bicycle trajectory prediction,Sudden-change behavior,Integrated framework,Deep learning,Utility analysis,Risk-efficiency tradeoff
AI 理解论文
溯源树
样例
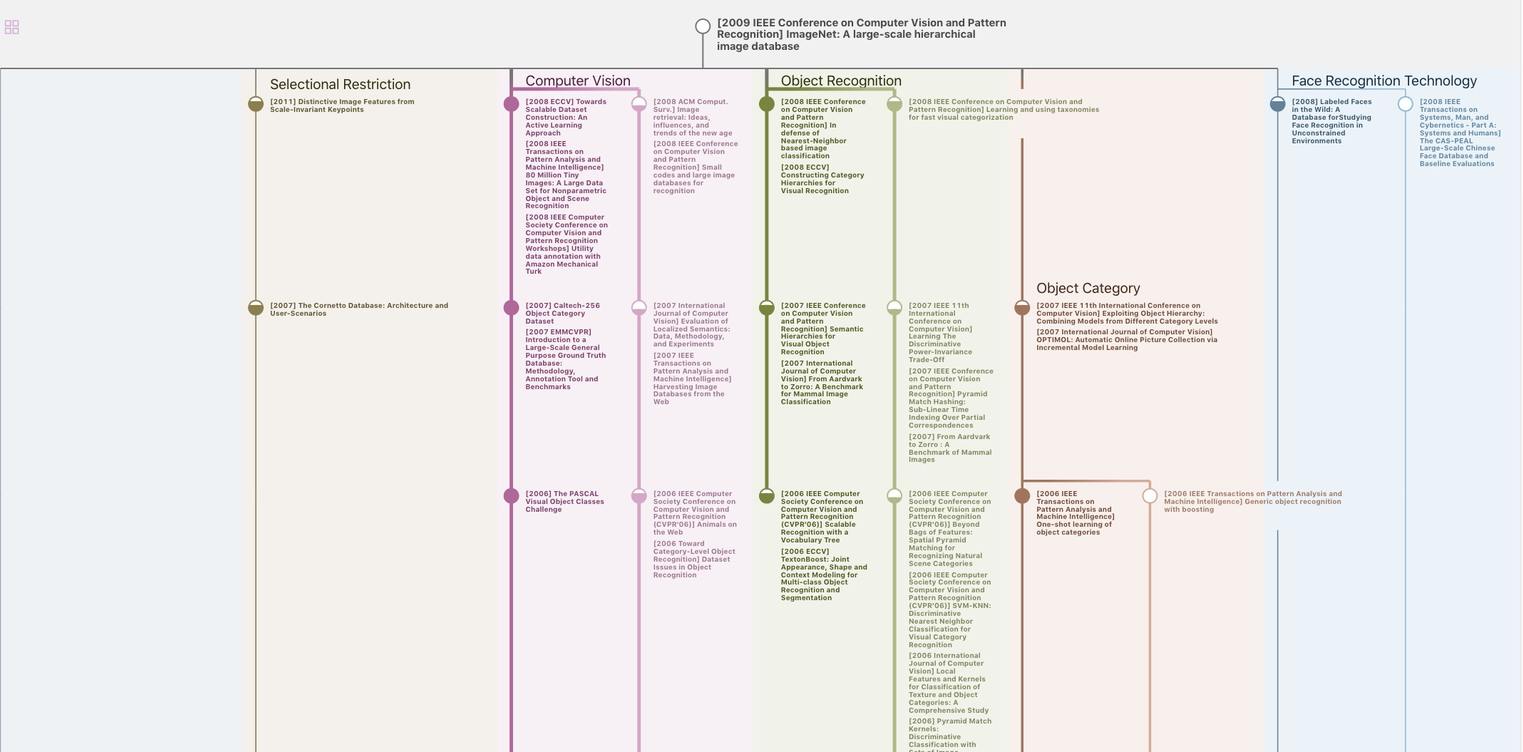
生成溯源树,研究论文发展脉络
Chat Paper
正在生成论文摘要