RDMA: low-light image enhancement based on retinex decomposition and multi-scale adjustment
INTERNATIONAL JOURNAL OF MACHINE LEARNING AND CYBERNETICS(2024)
摘要
Images are often affected by insufficient illumination and suffer from degradation problems such as low brightness, noise, and color distortion, which results in reduced image quality. Existing low-light image enhancement methods based on Retinex theory decompose images into reflectance and illumination components, which are adjusted separately; however, the intrinsic connection between reflectance and illumination during decomposition is not considered, and multi-scale information during subsequent adjustments is inadequately utilized. In this study, we propose a low-light image enhancement network based on Retinex decomposition and multi-scale adjustment (RDMA), which performs initial decomposition followed by subsequent adjustment. We utilized prior knowledge to design the feature interaction module (FIM) and the feature fusion module (FFM) for image decomposition. Furthermore, a coarse-to-fine multi-scale network with residual channel and spatial attention (RCSA) was designed to remove noise from reflectance, suppress color distortion, preserve image details, and adjust the brightness of illumination. An evaluation of various low-light image datasets and comparisons with state-of-the-art methods showed that the proposed network is superior in terms of enhancement results.
更多查看译文
关键词
Image restoration,Low-light image enhancement,Neural network,Deep learning
AI 理解论文
溯源树
样例
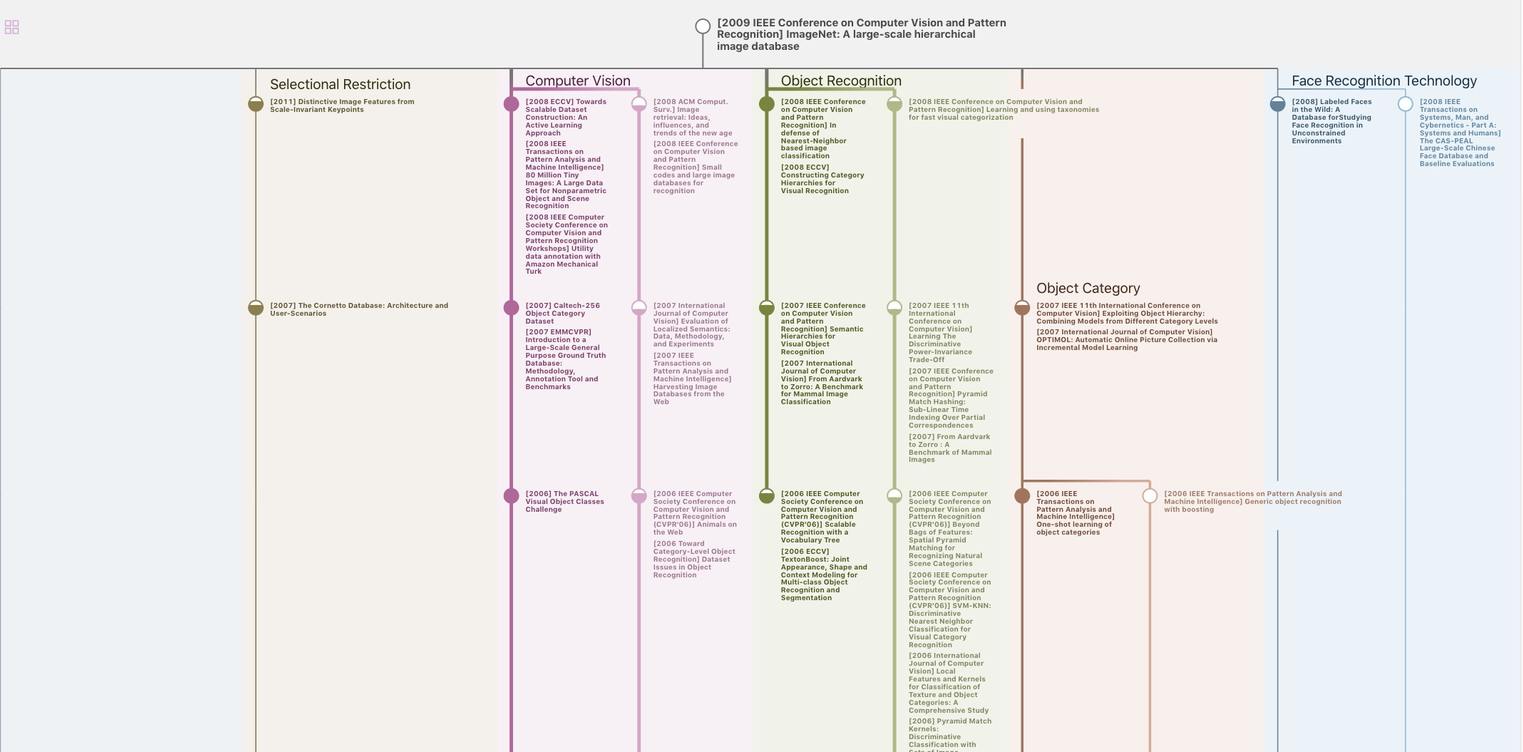
生成溯源树,研究论文发展脉络
Chat Paper
正在生成论文摘要