DIG: dual interaction and guidance network for salient object detection.
Appl. Intell.(2023)
摘要
The focus of salient object detection (SOD) research is to obtain rich and effective features for characterizing objects. Recent progress on SOD mainly aims at exploiting how to effectively extract and integrate multilevel object features in a SOD network. In some cases, existing approaches mainly consider the cross-layer or cross-modal information fusion in the decoder. What's more, they extract global context information from the last encoder layer and directly integrate them with outputs of each preceding layer, which pay less attention to refine the global context features and explore ways to overcome the scale variability of salient objects. These may limit the ability of the model to represent salient object to a certain extent. Based on this observation, we propose a novel Dual Interaction and Guidance module (namely DIG) for better leveraging the information of various encoder-outputs and global context information. Specifically, a Dual Interaction Module (DIM) is proposed to realize two-level-granularity feature interaction through a nested residual-like structure. According to the global context features, we design a Global Context Feature Enhancement (GCIE) and Global Guidance Module (GGM) to mine the deep information hidden in the original global information and effective guide feature fusion respectively. Embedding the DIG into a encode-decoder salient detection framework has greatly improved the characterization ability to salient objects. Through extensive experimental results on six benchmark datasets demonstrate that the proposed algorithm performs favorably against most state-of-the-art algorithms.
更多查看译文
关键词
salient,guidance network,detection,object
AI 理解论文
溯源树
样例
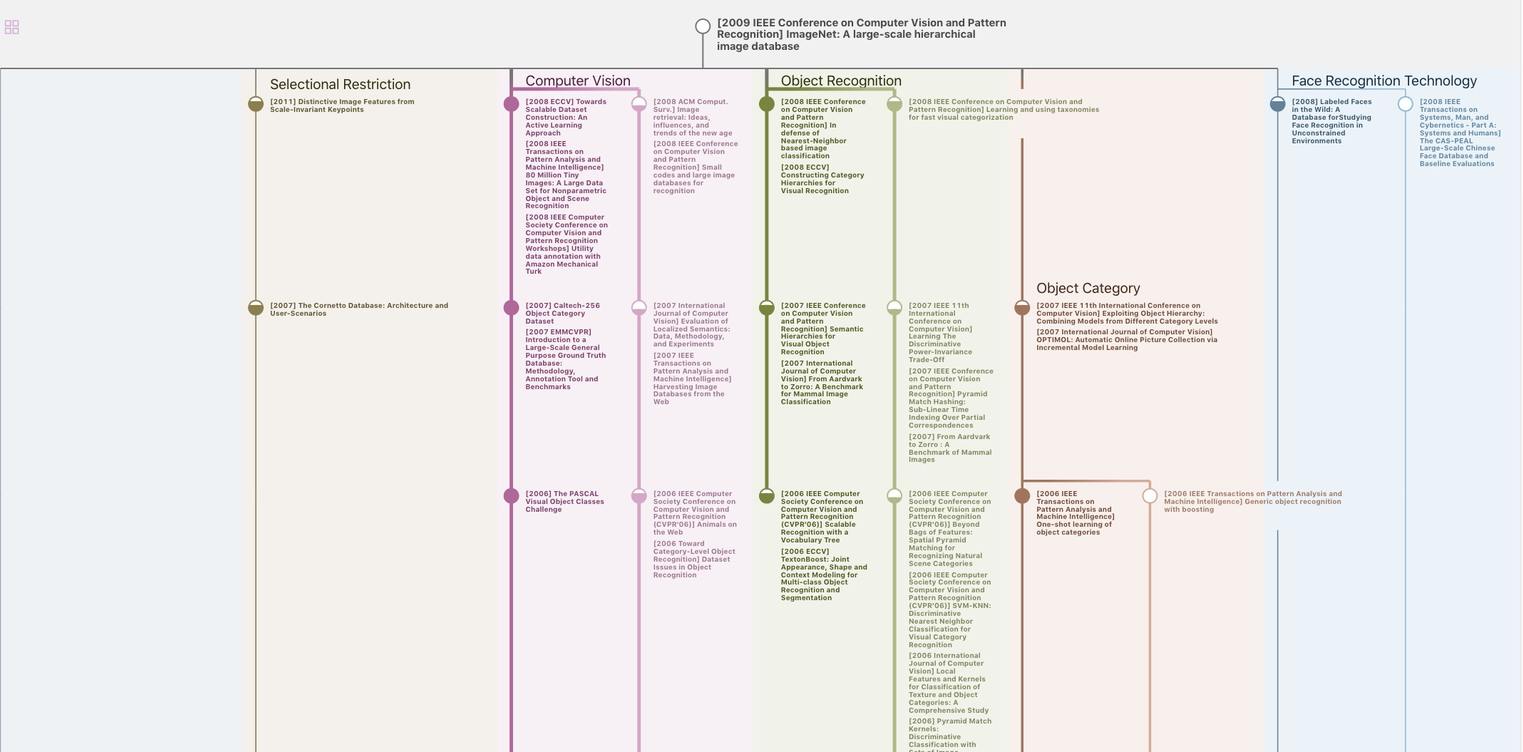
生成溯源树,研究论文发展脉络
Chat Paper
正在生成论文摘要