Towards efficient image-based representation of tabular data
NEURAL COMPUTING & APPLICATIONS(2023)
摘要
Convolutional neural networks (CNNs) have been widely used in image classification tasks and have achieved remarkable results compared with traditional methods. Their main advantage is the ability to extract hidden features automatically using local connectivity and spatial locality. However, CNN cannot be applied to tabular data, mainly due to the unsuitability of the tabular data structure to the CNN input. In this paper, we propose a new generic method for the representation of multidimensional tabular data as color-encoded images that can be used both for data visualization and classification with CNN. Our approach, named FC-Viz (Feature Clustering-Visualization), is based on user-oriented data visualization ideas, such as pixel-oriented techniques, feature clustering, and feature interactions. The proposed approach includes a transformation of each instance of the tabular data into a 2D pixel-based representation, where pixels representing features with strong correlation and interaction are adjacent to each other. We applied FC-Viz to ten multidimensional tabular datasets with dozens to thousands of features and compared its classification and visualization performance with a state-of-the-art tabular data transformation method. The evaluation experiments show that our approach is as accurate as the state-of-the-art, but with much smaller images resulting in much more compact and faster CNN models.
更多查看译文
关键词
Tabular data representation,Convolutional neural networks,Feature interaction,Feature clustering,Data visualization,Data transformation
AI 理解论文
溯源树
样例
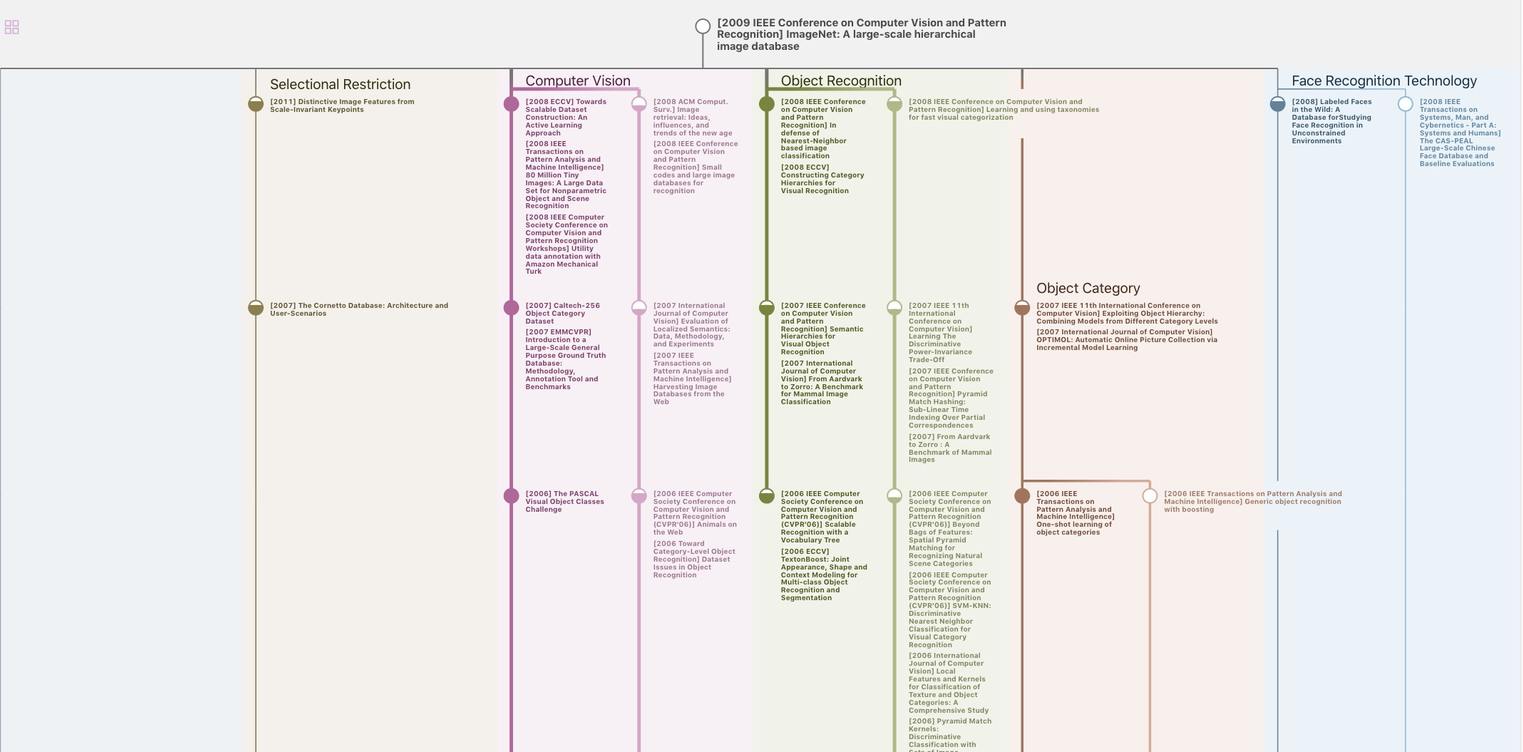
生成溯源树,研究论文发展脉络
Chat Paper
正在生成论文摘要