APO-AN feature selection based Glorot Init Optimal TransCNN landslide detection from multi source satellite imagery
MULTIMEDIA TOOLS AND APPLICATIONS(2023)
摘要
The work presents a comprehensive approach for landslide detection in satellite imagery by combining multiple techniques. The proposed methodology involves denoising the landslide image using the maximum information coefficient-based Wavelet Filter (MIC-WF) to enhance image quality. The denoising process with MIC-WF effectively reduces noise in the landslide image, resulting in improved image clarity and quality. To address the issue of overlapping pixels, an innovative approach based on Independent Component Analysis (ICA) based "Density-Based Spatial Clustering of Applications with Noise (DBSCAN)" is exploited for pixel separation. The separation of overlapping pixels using ICA-DBSCAN overcomes the challenge of pixel ambiguity, allowing for precise identification of individual landslide areas. Furthermore, the feature point discriptor as a feature selection method based on the Archimedes principle optimization-based attention network (APO-AN) is utilized to identify the most informative features for landslide detection. The feature selection based on APO-AN ensures that only the most relevant features are used for landslide detection, reducing computational complexity and enhancing model efficiency. Finally, a landslide detection model is developed using the Glorot Initialization Optimal TransCNN approach for accurate classification of landslide areas, leveraging the benefits of optimized weight initialization. FMRCNN, R-CNN, M-CNN, and CNN techniques achieve specificities of 93.47%, 92.11%, 91.78%, and 90.25%, respectively. The denoising, pixel separation, feature selection, and classification stages collectively contribute to the accurate detection of landslide areas in satellite imagery. The integration of these techniques offers a comprehensive solution for landslide detection, aiding in early warning systems and disaster management.
更多查看译文
AI 理解论文
溯源树
样例
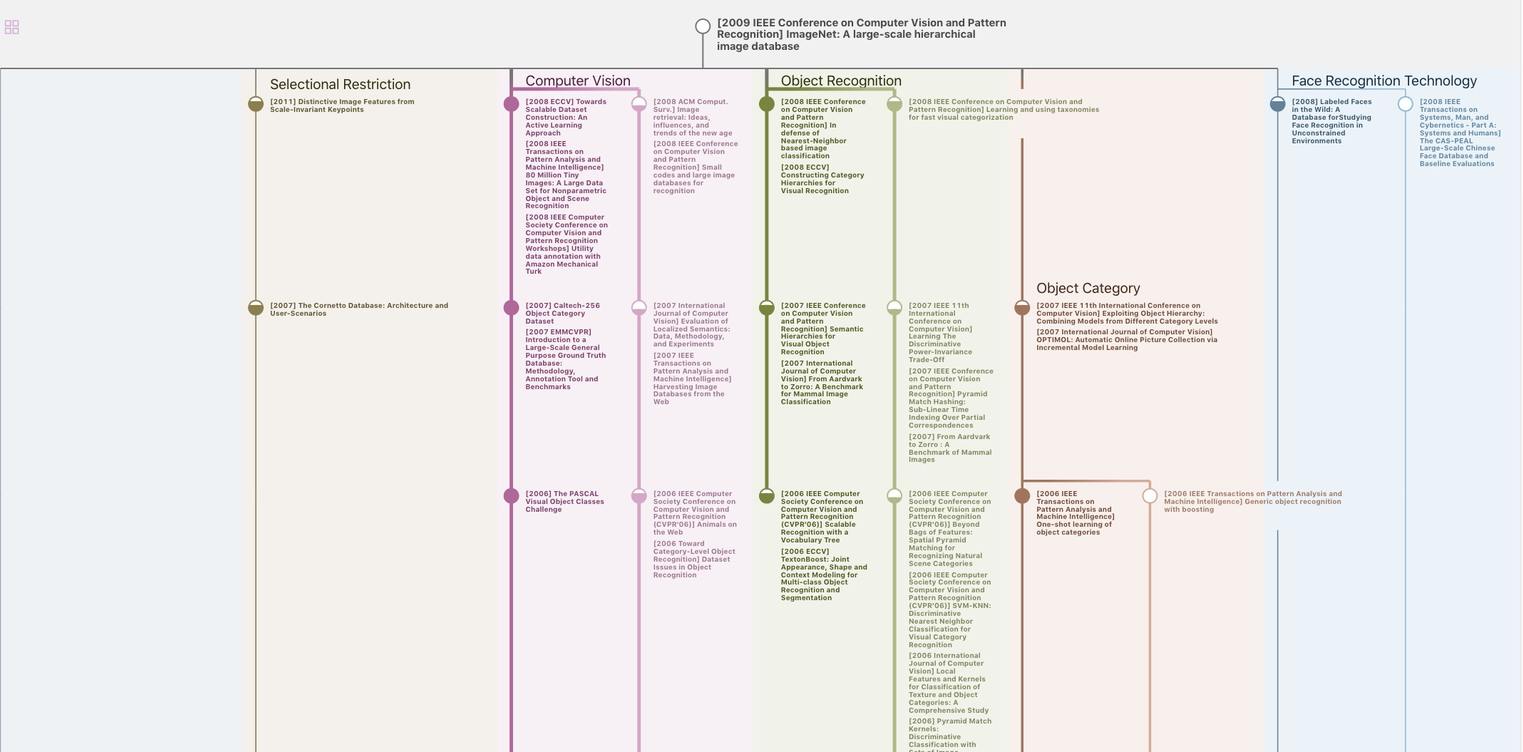
生成溯源树,研究论文发展脉络
Chat Paper
正在生成论文摘要