Energy data classification at the edge: a comparative studyfor energy efficiency applications
CLUSTER COMPUTING-THE JOURNAL OF NETWORKS SOFTWARE TOOLS AND APPLICATIONS(2023)
摘要
As the global economy is increasingly influenced by energy policy and efficiency, the opportunities of energy data classification are broadening. Performance metrics, especially for Deep Learning (DL), have motivated the accelerated development of computing hardware. In particular, High-Performance Edge Computing (HPEC) has an important role in complementing cloud computing in the collection, pre-processing, and post-processing of big data in a more privacy-preserving manner. Yet, such opportunities bring challenges concerning the selection of hardware platforms and classification algorithms. Therefore, in this article, we aim to carry out a comparative study that examines the merits, performance and efficiency metrics, and limitations of notable HPEC platforms centered around a DL classification framework. In this implementation, a 2D Gramian Angular Field (GAF) energy data classifier is presented and run on a publicly available dataset. Following, a DL classifier is trained and exported as a lightweight counterpart for validation on several commonly used HPEC platforms, namely the Jetson Nano, ODROID-XU4, Coral Dev Board, and the BeagleBone AI. Current results show competitive computational performance among the reviewed platforms, as fast as similar to 8 ms per classified image.
更多查看译文
关键词
Energy efficiency, High performance edge computing, Artificial intelligence, Data lake, Deep learning, Internet of energy, Gramian angular fields
AI 理解论文
溯源树
样例
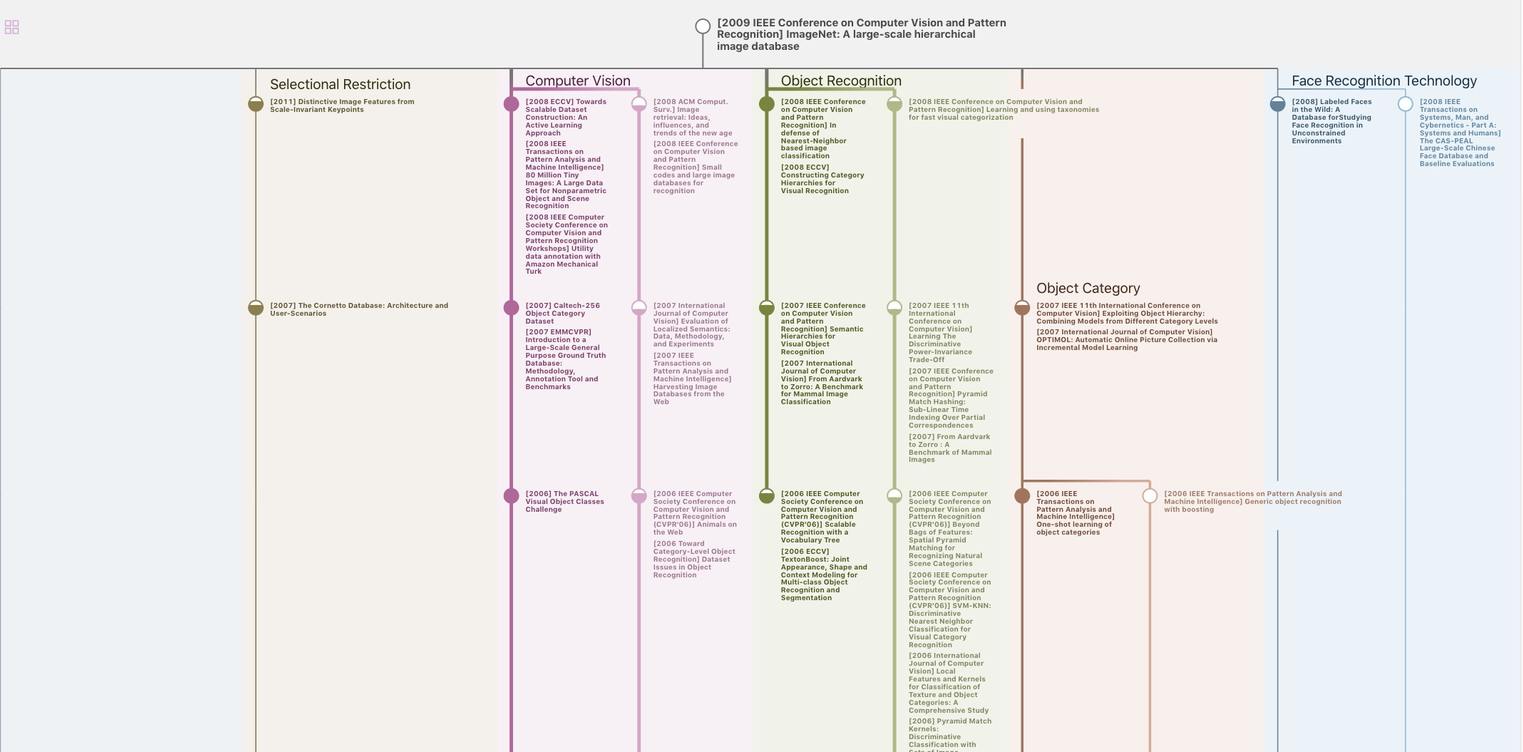
生成溯源树,研究论文发展脉络
Chat Paper
正在生成论文摘要