Adversarial enhanced attributed network embedding
KNOWLEDGE AND INFORMATION SYSTEMS(2024)
摘要
Attributed network embedding aims to extract latent features of complex networks from structural topology and node attributes. Existing embedding models either use two separate learning processes to capture the complementarity of network topology and node attributes, or one process to capture their consistency. However, it is rare to simultaneously consider the complementarity and consistency of the network topology and node attributes in a single model, and network noise is often ignored. To address these issues, an unsupervised adversarial enhanced attributed network embedding model is proposed in this paper, called AEANE. The model firstly designs a novel feature extractor to simultaneously learn the consistency and complementarity features of node attributes and network topology, aiming to capture more accurate and comprehensive features. Secondly, a noisy version of the feature extractor is constructed as a fake feature generator to quickly generate more unseen fake features, aiming to simulate network noise and construct two game players. Finally, a novel adversarial auto-encoder network is designed, which integrates the auto-encoder network and the generative adversarial network into a single network, aiming to quickly learn robust node embedding and tolerate network noise. Experiments on four publicly available datasets and three analysis tasks demonstrate the advantages of our AEANE model.
更多查看译文
关键词
Attribute network,Attribute network embedding,Adversarial learning,Consistent feature,Complementary feature
AI 理解论文
溯源树
样例
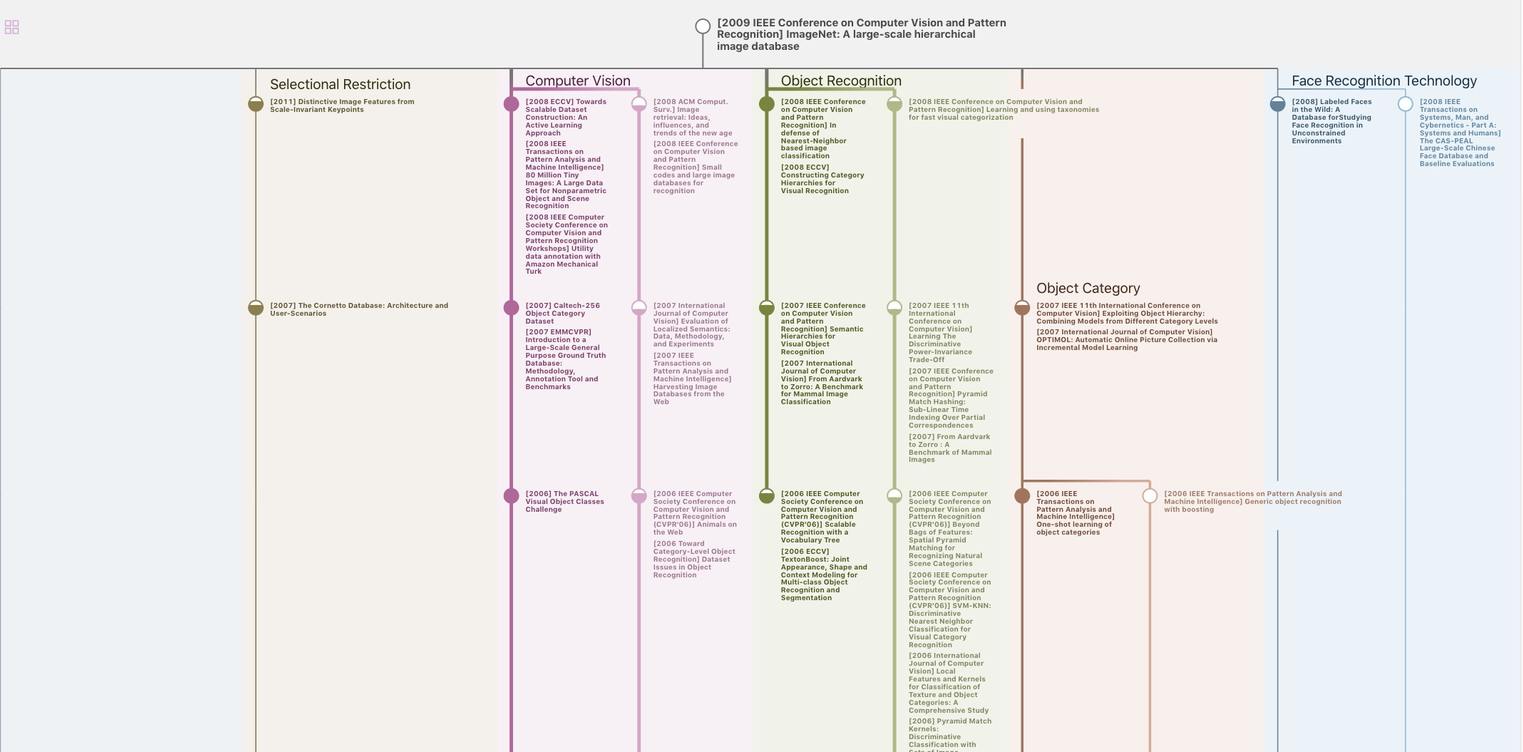
生成溯源树,研究论文发展脉络
Chat Paper
正在生成论文摘要