A Lightweight Method of Knowledge Graph Convolution Network for Collaborative Filtering
INTERNATIONAL JOURNAL ON SEMANTIC WEB AND INFORMATION SYSTEMS(2023)
摘要
In recent years, knowledge-aware recommendation systems have gained popularity as a solution to address the challenges of data sparsity and cold start in collaborative filtering. However, traditional knowledge graph convolutional networks impose significant computational burdens during training, demanding substantial resources and increasing the cost of recommendations. To address this issue, this article proposes a lightweight knowledge graph convolutional network for collaborative filtering (LKGCF). LKGCF eliminates the feature transformation and nonlinear activation components, by focusing on essential elements such as neighborhood aggregation and layer combination. LKGCF captures the user's long-distance personalized interests on the knowledge graph by sampling from neighborhood information and constructing a weighted sum of item embeddings. Experimental results demonstrate that the proposed model is easy to train and implement due to its coherence and simplicity. Furthermore, notable improvements in recommendation performance are observed compared to strong baselines.
更多查看译文
关键词
collaborative filtering,graph convolution network,knowledge graph
AI 理解论文
溯源树
样例
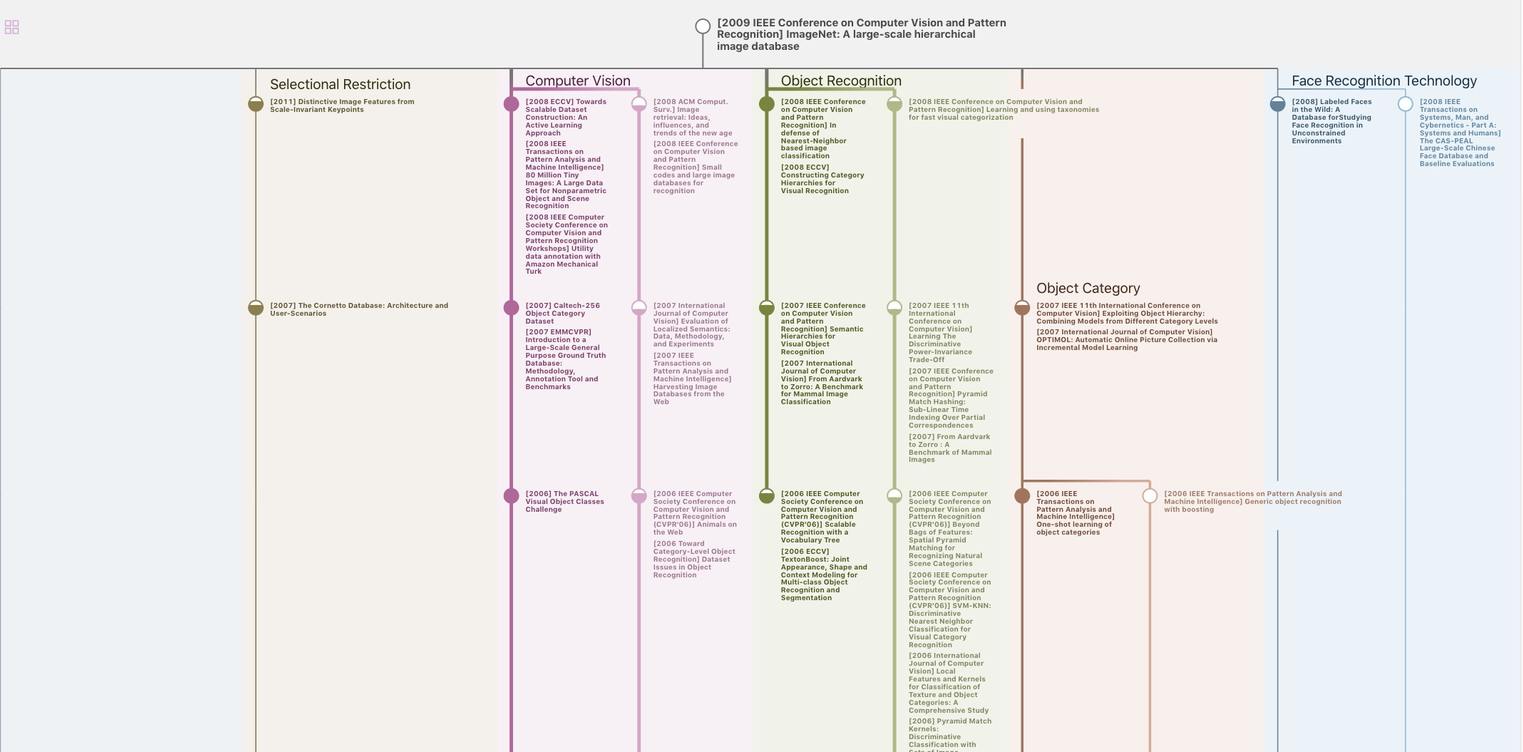
生成溯源树,研究论文发展脉络
Chat Paper
正在生成论文摘要