A temporal convolutional recurrent autoencoder based framework for compressing time series data
APPLIED SOFT COMPUTING(2023)
摘要
The sharply growing volume of time series data due to recent sensing technology advancement poses emerging challenges to the data transfer speed and storage as well as corresponding energy consumption. To tackle the overwhelming volume of time series data in transmission and storage, compressing time series, which encodes time series into smaller size representations while enables authentic restoration of compressed ones with minimizing the reconstruction error, has attracted significant attention. Numerous methods have been developed and recent deep learning ones with minimal assumptions on data characteristics, such as recurrent autoencoders, have shown themselves to be competitive. Yet, capturing long-term dependencies in time series compression is a signifi-cant challenge calling further development. To make a response, this paper proposes a temporal convolutional recurrent autoencoder framework for more effective time series compression. First, two autoencoder modules, the temporal convolutional network encoder with a recurrent neural network decoder (TCN-RNN) and the temporal convolutional network encoder with an attention assisted recurrent neural network decoder (TCN-ARNN), are developed. The TCN-RNN employs only the recurrent neural network decoder to reconstruct the time series in reverse order. In contrast, the TCN-ARNN uses two recurrent neural networks to reconstruct the time series in both forward and reverse order in parallel. In addition, a timestep-wise attention network is developed to incorporate the forward and reverse reconstructions into the ultimate reconstruction with adaptive weights. Finally, a model selection procedure is developed to adaptively select between the TCN-RNN and TCN-ARNN based on their reconstruction performance on the validation dataset. Computational experiments on five datasets show that the proposed temporal convolutional recurrent autoencoder outperforms state-of-the-art benchmarking models in terms of lower reconstruction errors with the same compression ratio, achieving an improvement of up to 45.14% in the average of mean squared errors. Results indicate a promising potential of the proposed temporal convolutional recurrent autoencoder on the time series compression for various applications involving long time series data. (c) 2023 Elsevier B.V. All rights reserved.
更多查看译文
关键词
Attention mechanism,Data compression,Deep learning,Recurrent neural networks,Temporal convolutional networks
AI 理解论文
溯源树
样例
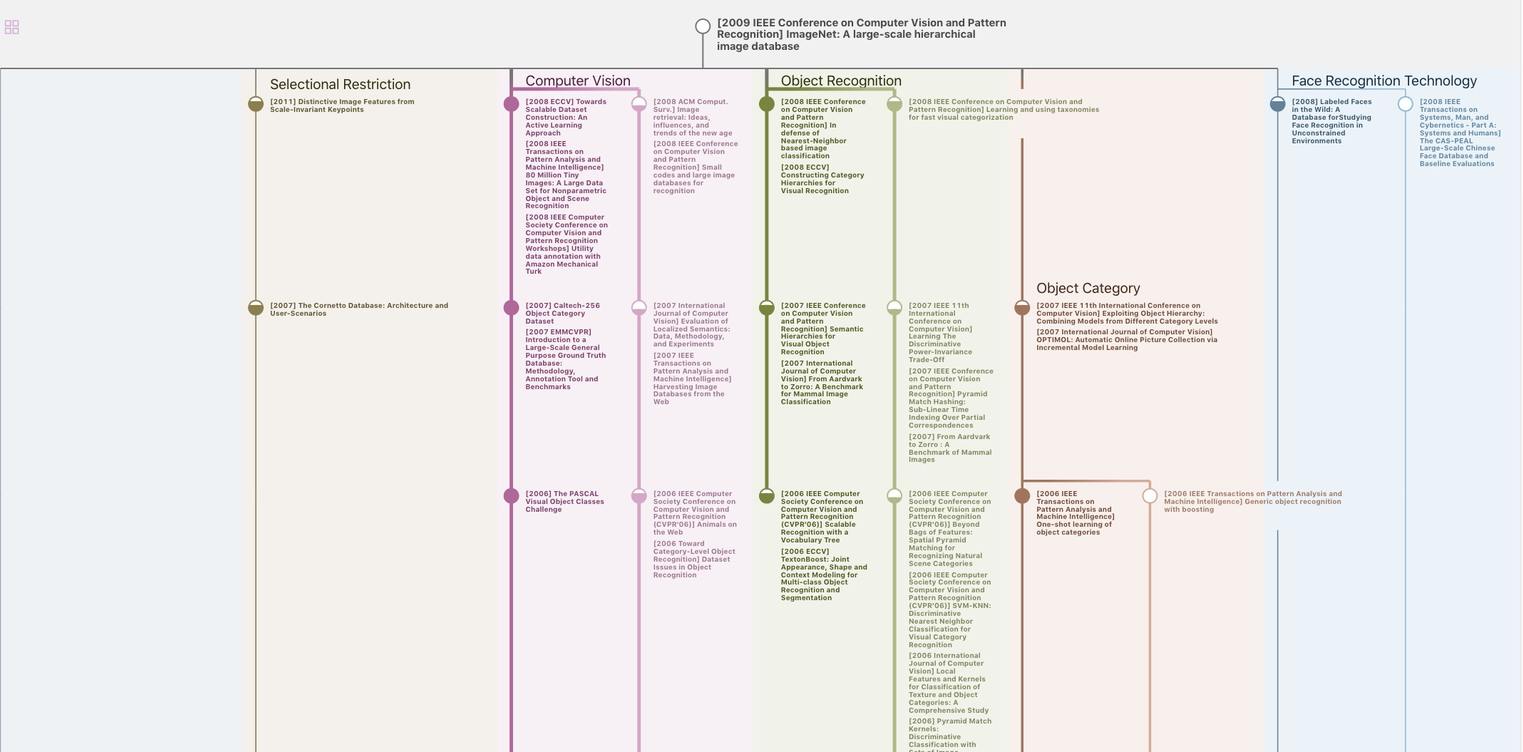
生成溯源树,研究论文发展脉络
Chat Paper
正在生成论文摘要