Prior-based collaborative representation with global adaptive weight for hyperspectral anomaly detection
JOURNAL OF APPLIED REMOTE SENSING(2023)
摘要
Hyperspectral anomaly detection (HAD) is a technique to find observations without prior knowledge, which is of particular interest as a branch of remote sensing object detection. However, the application of HAD is limited by various challenges, such as high-dimensional data, high intraclass variability, redundant information, and limited samples. To overcome these restrictions, we report an unsupervised strategy to implement HAD by dimensionality reduction (DR) and prior-based collaborative representation with adaptive global salient weight. The proposed framework includes three main steps. First, we select the most discriminating bands as the input hyperspectral images for subsequent processing in a DR manner. Then, we apply piecewise-smooth prior and local salient prior to collaborative representation to produce the initial detection map. Finally, to generate the final detection map, a global adaptive salient map is applied to the initial anomaly map to further highlight anomalies. Most importantly, the experimental results show that the proposed method outperforms alternative detectors on several datasets over different scenes. In particular, on the Gulfport dataset, the area under the curve value obtained by the proposed method is 0.9932, which is higher than the second-best method, convolutional neural network detector, by 0.0071.
更多查看译文
关键词
anomaly detection,hyperspectral imagery,remote sensing,collaborative representation
AI 理解论文
溯源树
样例
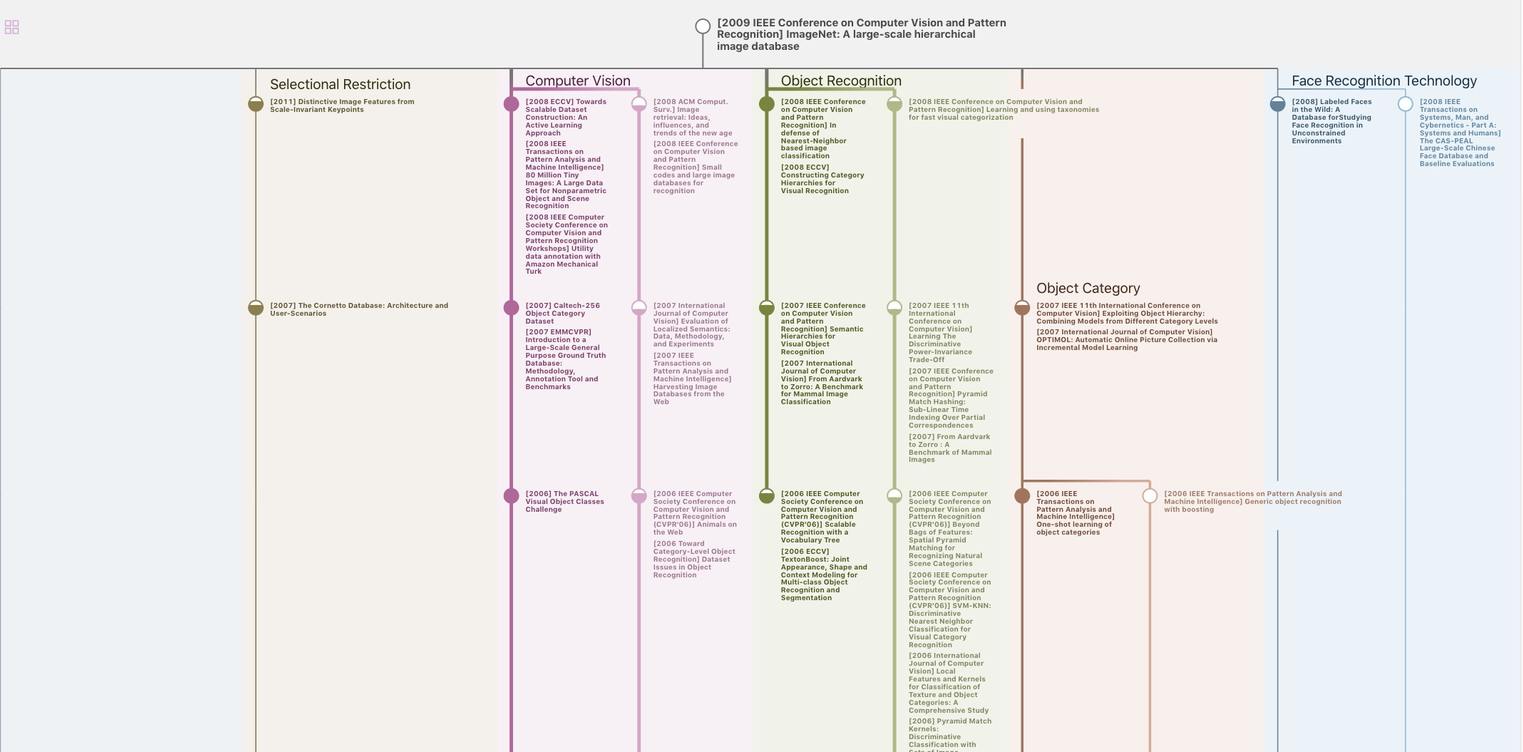
生成溯源树,研究论文发展脉络
Chat Paper
正在生成论文摘要