A Nonvolatile AI-Edge Processor With SLC-MLC Hybrid ReRAM Compute-in-Memory Macro Using Current-Voltage-Hybrid Readout Scheme
IEEE JOURNAL OF SOLID-STATE CIRCUITS(2024)
摘要
On-chip non-volatile compute-in-memory (nvCIM) enables artificial intelligence (AI)-edge processors to perform multiply-and-accumulate (MAC) operations while enabling the non-volatile storage of weight data in power-off mode to enhance energy efficiency. However, the design challenges of nvCIMbased AI-edge processors include: 1) lack of a nvCIM-friendly computing flow; 2) a tradeoff between usage of memory devices versus process variations, computing yield and area overhead; 3) long computing latency and low energy efficiency; and 4) small signal margin and large bitline current. This article presents an nvCIM-friendly AI-edge processor that uses a hybrid-mode resistive random access memory nvCIM (hmRe-nvCIM) macro to overcome the abovementioned challenges by three processor-level schemes: 1) a multimode nvCIM engine controller (mmCIMEC); 2) a bitwise-input-sparsity and place-value-aware dynamic accumulation (BIS-PVA-DA); and 3) a bitwise weight column inversion (BWCI) and two macro-level schemes: 1) a dynamic accumulation-aware current quantization (DACQ) and 2) a current-voltage-hybrid analog-to-digital converter (CVH-ADC). The proposed AI-edge processor fabricated using 22-nm technology achieved 51.4 TOPS/W and 472.7-mu s wake-up to response time, while the hmRe-nvCIM macro achieved 67.2 TOPS/W under 8-bit input, 8-bit weight, and 22-or 24-bit output precision.
更多查看译文
关键词
Energy efficiency,Nonvolatile memory,Energy consumption,System-on-chip,Process control,Neural networks,Memory management,Artificial intelligence (AI),compute-in-memory (CIM),convolution neural network (CNN) edge processors,hybrid readout,multiply-and-accumulate (MAC),ReRAM
AI 理解论文
溯源树
样例
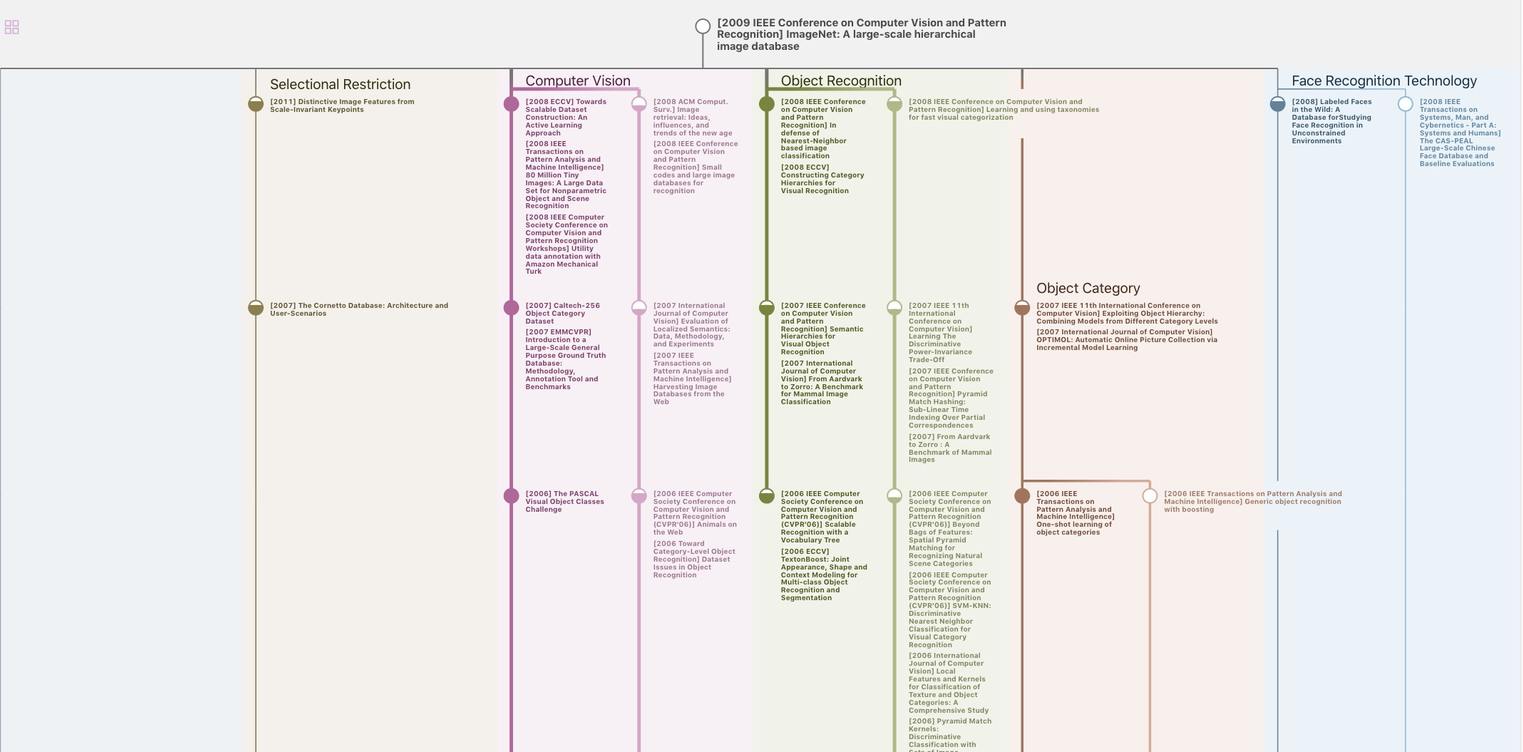
生成溯源树,研究论文发展脉络
Chat Paper
正在生成论文摘要