Switching threshold event-triggered critic algorithm for optimal orbit tracking and formation motion
IET CONTROL THEORY AND APPLICATIONS(2024)
摘要
This paper deals with the orbit tracking and formation motion problems with optimal energies and reduced computational cost. First, the orbit tracking and formation motion are decomposed into movements in the normal and tangent directions of level orbits, respectively, and simultaneously, the optimal value functions in both directions are defined. Then, to reduce the computational cost, a switching threshold event-triggered (STET) mechanism is designed. Based on the STET mechanism, the optimal value functions are constructed to evaluate the optimal energies of orbit tracking and formation motion. Critic neural networks are then designed to approximate the optimal value functions, which yield the optimal policies along the normal and tangent directions of desired orbits, that is, a so-called switching threshold event-triggered critic algorithm (STET-C). Theoretical analysis of system convergence is given in detail. Finally, two comparison simulations are given. The former intends to verify the optimal energy of STET-C compared to the feedback controllers. The latter shows that STET-C significantly reduces the computational cost in contrast with the non-triggered actor-critic algorithm, non-triggered critic algorithm, and the relative threshold event-triggered critic algorithm. This paper proposes a novel switching threshold event-triggered critic algorithm for optimal orbit tracking and formation motion with optimal energy and reduced computational cost. Theoretical analysis and comparative simulations show that the computational cost is significantly reduced by the switching threshold event-triggered mechanism compared with other optimal and event-triggered methods, and the optimal value functions of energy on orbit tracking and formation motion are achieved through critic neural networks with optimal policies.image
更多查看译文
关键词
distributed control,multi-agent systems,optimal control
AI 理解论文
溯源树
样例
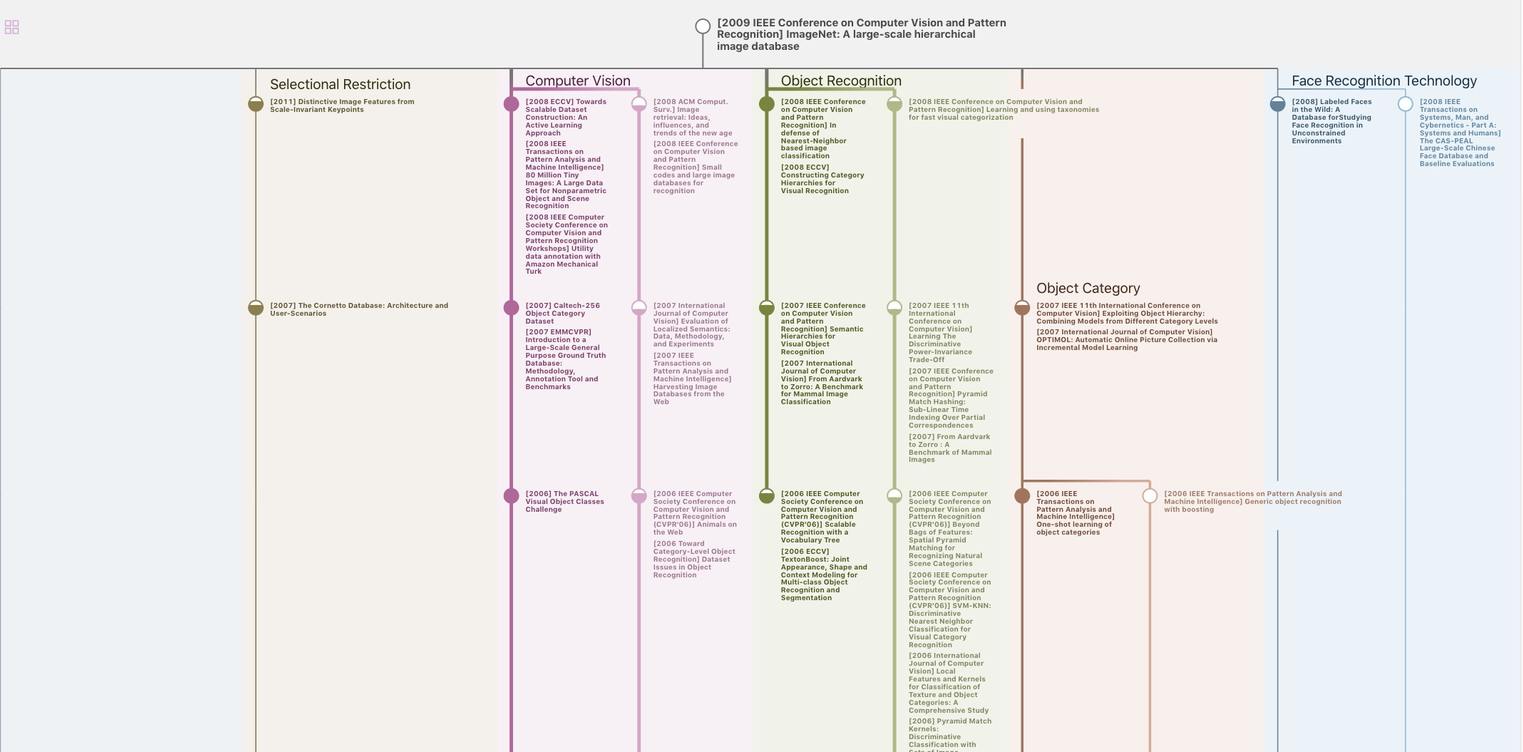
生成溯源树,研究论文发展脉络
Chat Paper
正在生成论文摘要