A Graph Memory Neural Network for Sea Surface Temperature Prediction
REMOTE SENSING(2023)
摘要
Sea surface temperature (SST) is a key factor in the marine environment, and its accurate forecasting is important for climatic research, ecological preservation, and economic progression. Existing methods mostly rely on convolutional networks, which encounter difficulties in encoding irregular data. In this paper, allowing for comprehensive encoding of irregular data containing land and islands, we construct a graph structure to represent SST data and propose a graph memory neural network (GMNN). The GMNN includes a graph encoder built upon the iterative graph neural network (GNN) idea to extract spatial relationships within SST data. It not only considers node but also edge information, thereby adequately characterizing spatial correlations. Then, a long short-term memory (LSTM) network is used to capture temporal dynamics in the SST variation process. We choose the data from the Northwest Pacific Ocean to validate GMNN's effectiveness for SST prediction in different partitions, time scales, and prediction steps. The results show that our model has better performance for both complete and incomplete sea areas compared to other models.
更多查看译文
关键词
graph memory neural network,neural network,temperature,sea
AI 理解论文
溯源树
样例
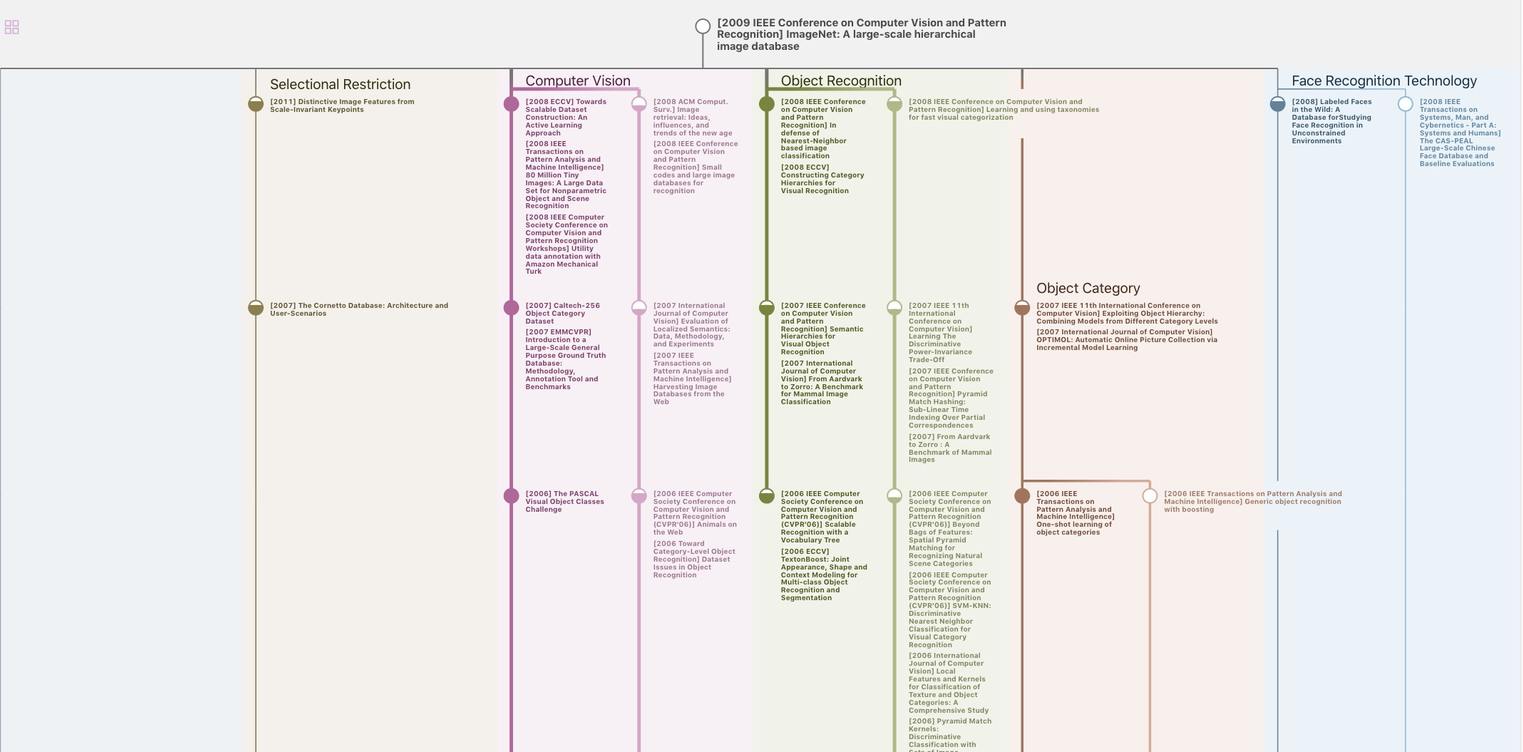
生成溯源树,研究论文发展脉络
Chat Paper
正在生成论文摘要