Developing a data-driven modeling framework for simulating a chemical accident in freshwater
JOURNAL OF CLEANER PRODUCTION(2023)
摘要
Chemical accidents in freshwater pose threats to public health and aquatic ecosystems. Process-based models (PBMs) have been used to identify spatiotemporal chemical distributions in natural water. However, their computationally expensive simulations can hinder timely incident responses, which are crucial for minimizing negative impacts. Therefore, this study proposes a site-specific data-driven model (DDM) to supplement PBM-based chemical accident simulations. A convolutional neural network (CNN) was employed as the DDM because of its outstanding performance in capturing spatial patterns. Our model was developed to facilitate chemical accident simulations in the Namhan River, South Korea. The model datasets were generated using the PBM simulation outputs from toluene accident scenarios. Our DDM showed a Nash-Sutcliffe-efficiency of 0.94 and a root-mean-square-error of 0.023 μg/L for the validation set. Its computational time was approximately 64 times faster than that of PBMs. In addition, this study interpreted the DDM results using SHapley Additive exPlanations (SHAP). The SHAP findings highlighted the influential role of distance from the accident site in this study. Overall, this study demonstrated the applicability of our modeling approach in freshwater chemical accidents by providing rapid spatial distribution results complementing PBM simulations.
更多查看译文
关键词
Chemical accident modeling,CNN,EFDC,Explainable AI,SHAP
AI 理解论文
溯源树
样例
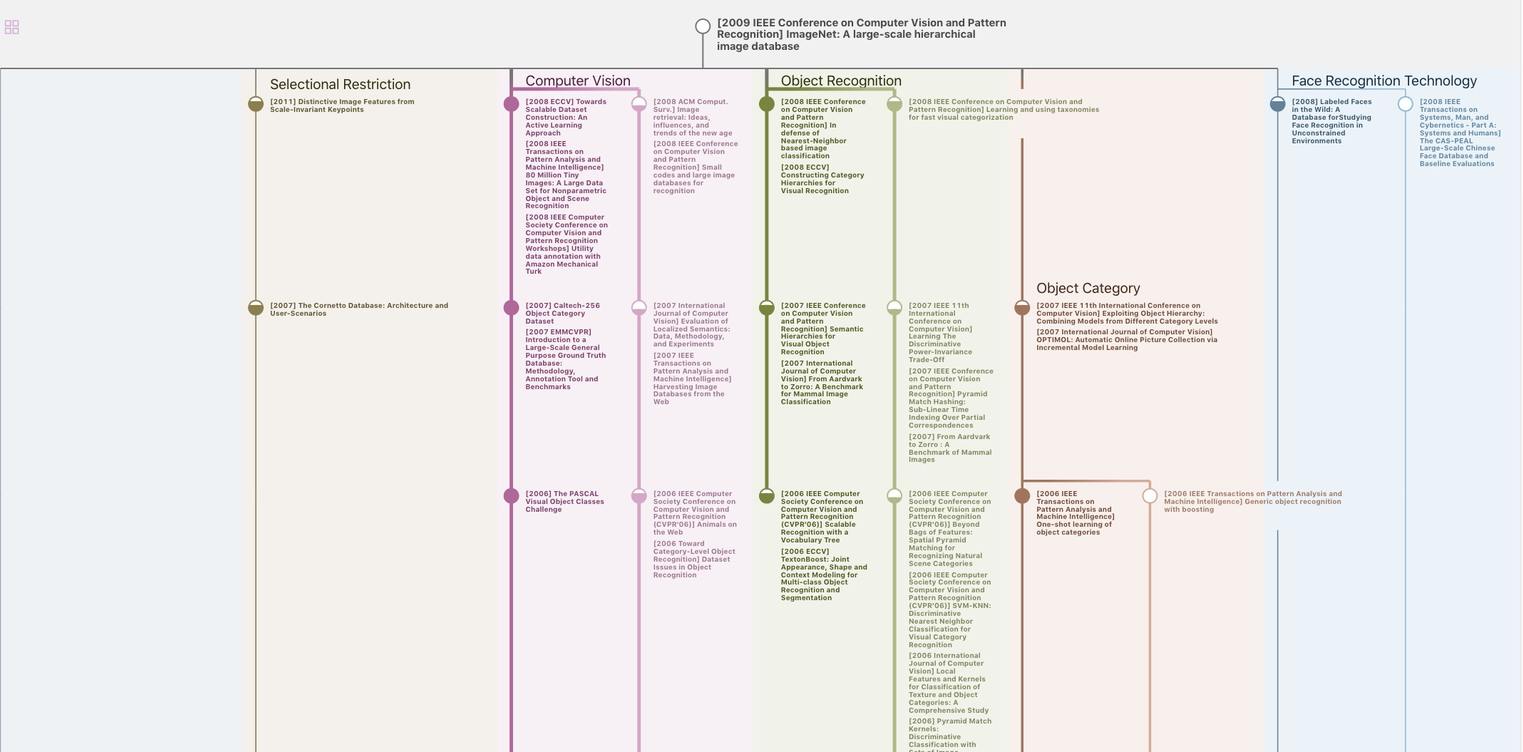
生成溯源树,研究论文发展脉络
Chat Paper
正在生成论文摘要