Surrogate Model Development for Slope Stability Analysis Using Machine Learning
SUSTAINABILITY(2023)
摘要
In many countries, slope failure is a complex natural issue that can result in serious natural hazards, such as landslide dams. It is associated with the challenge of slope stability evaluation, which involves the classification problem of slopes and the regression problem of predicting the factor of safety (FOS) value. This study explored the implementation of machine learning to analyze slope stability using a comprehensive database of 880 homogenous slopes (266 unstable and 614 stable) based on a simulation model developed as a surrogate model. A classification model was developed to categorize slopes into three classes, including S (stable, FOS > 1.2), M (marginally stable, 1.0 = FOS = 1.2), and U (unstable, FOS < 1.0), and a regression model was used to predict the target FOS value. The results confirmed the efficiency of the developed classification model via testing, achieving an accuracy of 0.9222, with 96.2% accuracy for the U class, 55% for the M class, and 95.2% for the S class. When U and M are in the same class (i.e., the U + M class), the test accuracy is 0.9315, with 93.3% accuracy for the S class and 92.9% accuracy for the U + M class. The low accuracy level for class M led to minor inaccuracies, which can be attributed to a data imbalance. Additionally, the regression model was found to have a high correlation coefficient R-square value of 0.9989 and a low test mean squared error value of 5.03 x 10(-4), which indicates a strong relationship between the FOS values and the selected slope parameters. The significant difference in the elapsed time between the traditional method and the developed surrogate model for slope stability analysis highlights the potential benefits of machine learning.
更多查看译文
关键词
slope stability, factor of safety, machine learning, surrogate model
AI 理解论文
溯源树
样例
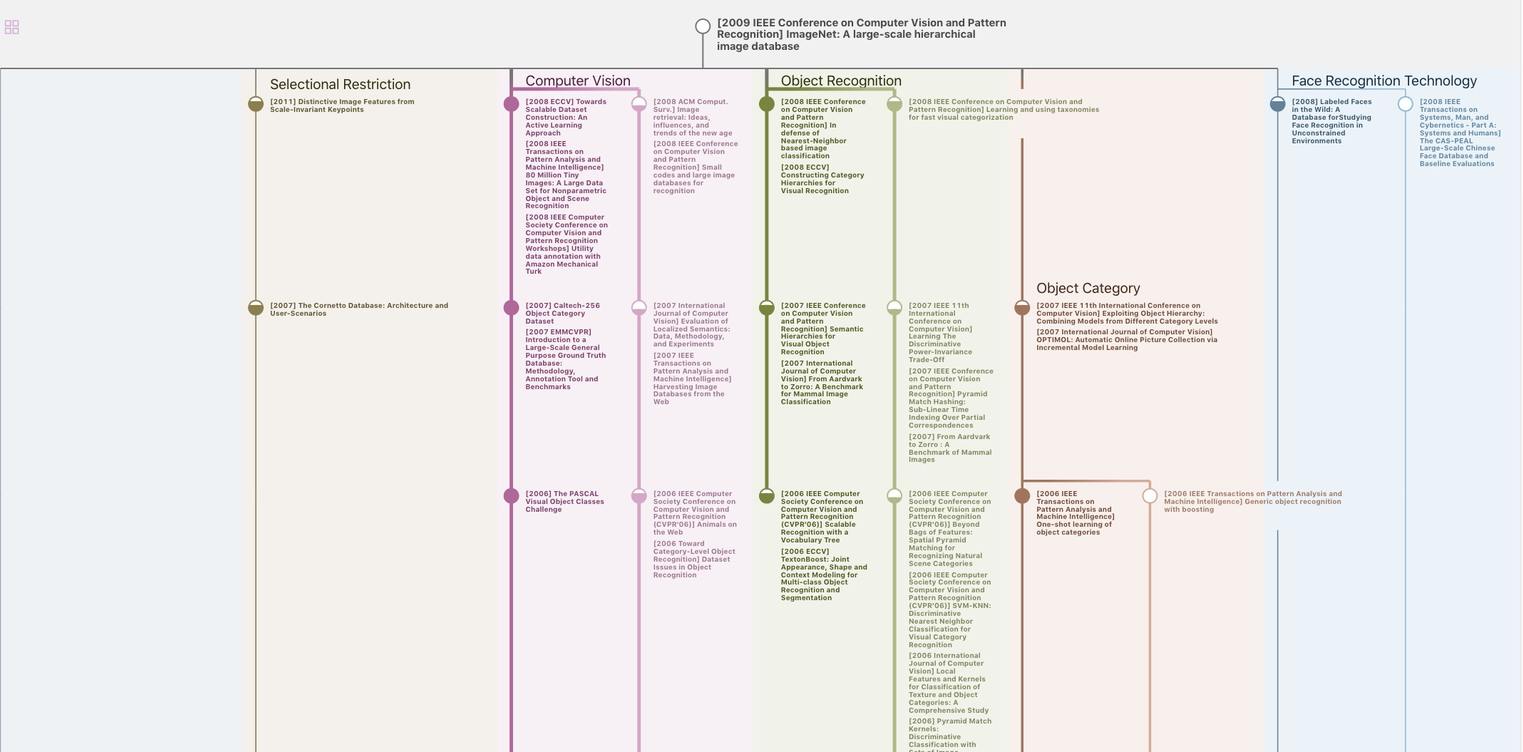
生成溯源树,研究论文发展脉络
Chat Paper
正在生成论文摘要