A Full-Reference Image Quality Assessment Method via Deep Meta-Learning and Conformer
IEEE TRANSACTIONS ON BROADCASTING(2024)
摘要
In this paper, a full-reference image quality assessment (FR-IQA) model based on deep meta-learning and Conformer is proposed. We combine the Conformer architecture with a Siamese network to extract the feature vectors of the reference and distorted images and calculate the similarity of these feature vectors as the predicted score of the image. We use meta-learning to help the model identify different types of image distortion. First, because the information taken as input by the human visual system (HVS) ranges in scale from local to global, we use a Conformer network as a feature extractor to obtain the global and local features of the pristine and distorted images and use a Siamese network to reduce the number of parameters in our model. Second, we use meta-learning to carry out bilevel gradient descent from the query set to the support set in the training stage and fine-tune the model parameters on a few images with unknown distortion types in the testing stage to improve the generalization ability of the model. Experiments show that our method is competitive with existing FR-IQA methods on three standard IQA datasets.
更多查看译文
关键词
Feature extraction,Metalearning,Deep learning,Image quality,Distortion,Task analysis,Visualization,Full-reference image quality assessment,meta-learning,knowledge-driven,conformer
AI 理解论文
溯源树
样例
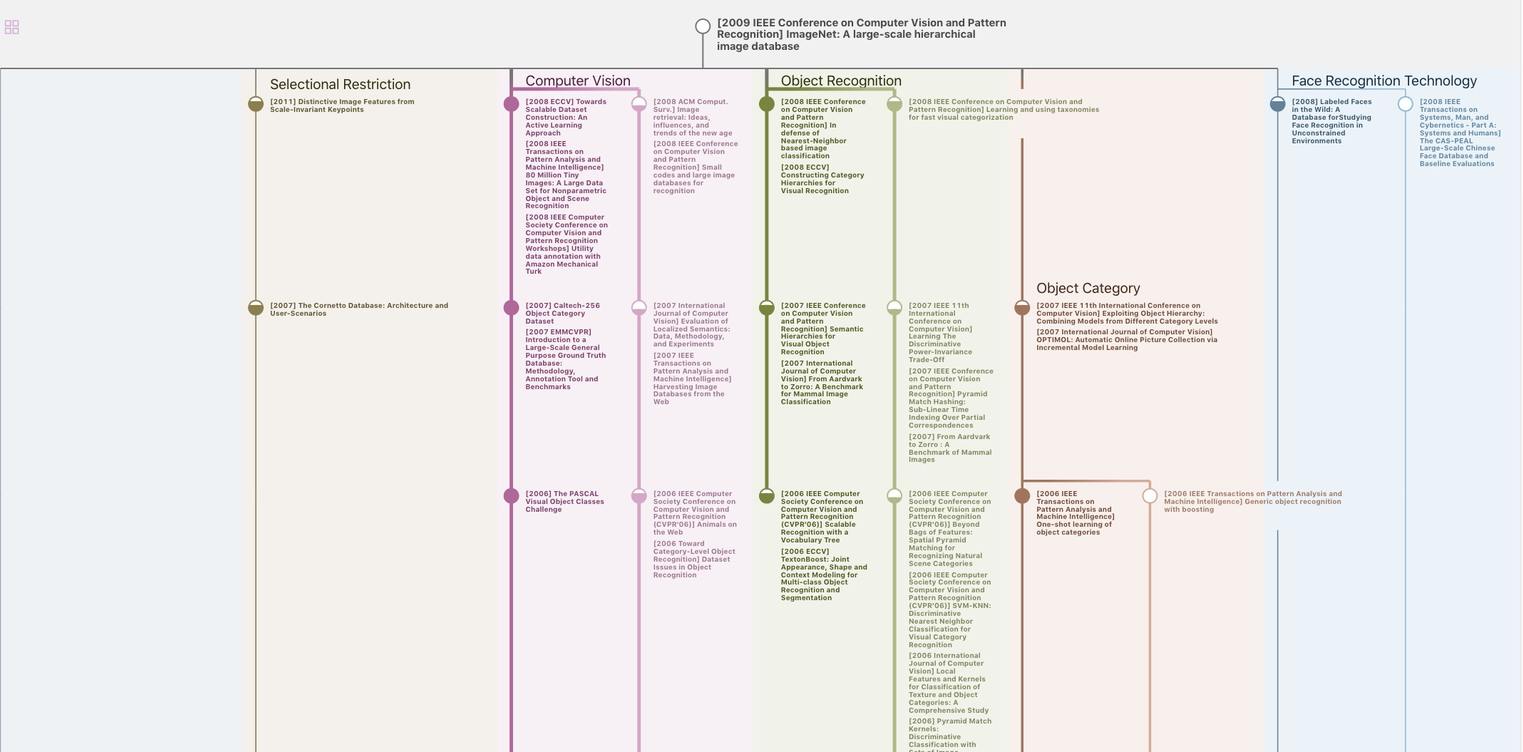
生成溯源树,研究论文发展脉络
Chat Paper
正在生成论文摘要