Flow-Inflated Selective Sampling for Efficient Agent-Based Dynamic Ride-Pooling Simulations
TRANSPORTATION RESEARCH RECORD(2024)
摘要
Agent-based simulations are powerful tools for simulating emergent mobility modes, but they often require significant memory and computing power. To address this issue, researchers have previously used sampling techniques, where only a fraction of agents are explicitly simulated while others are simulated through teleportation. However, recent studies have highlighted the challenges of scaling ride-pooling simulations because of their reliance on demand density, which does not scale linearly. In this study, we introduce a new methodology for simulating dynamic ride-pooling services called flow-inflated selective sampling (FISS). Unlike previous approaches, FISS considers all agents but-for selected modes-only explicitly simulates a fraction of their trips while simulating the remaining trips through teleportation. Here, we explicitly simulate all public transport and ride-pooling trips and sample private car trips. The capacity consumption of explicitly simulated cars is scaled up to obtain realistic traffic flows, rather than adjusting the network capacity as in previous approaches. We implement FISS in the MATSim simulation environment for a large scenario in Munich, Germany, and show that it preserves traffic flows while keeping key performance indicators of ride-pooling services stable and unbiased. Mode choice decisions based on FISS also remain stable, and runtimes of the assignment can be almost halved. Overall, FISS is a simple yet effective approach that can significantly reduce the computational burden of agent-based simulations while maintaining the accuracy of the results. It can be particularly useful for simulating ride-pooling services, which can be challenging to scale because of their dependence on demand density.
更多查看译文
关键词
operations,multi-agent simulation,planning and analysis,travel demand modeling,dynamic traffic assignment,network assignment,simulation modeling
AI 理解论文
溯源树
样例
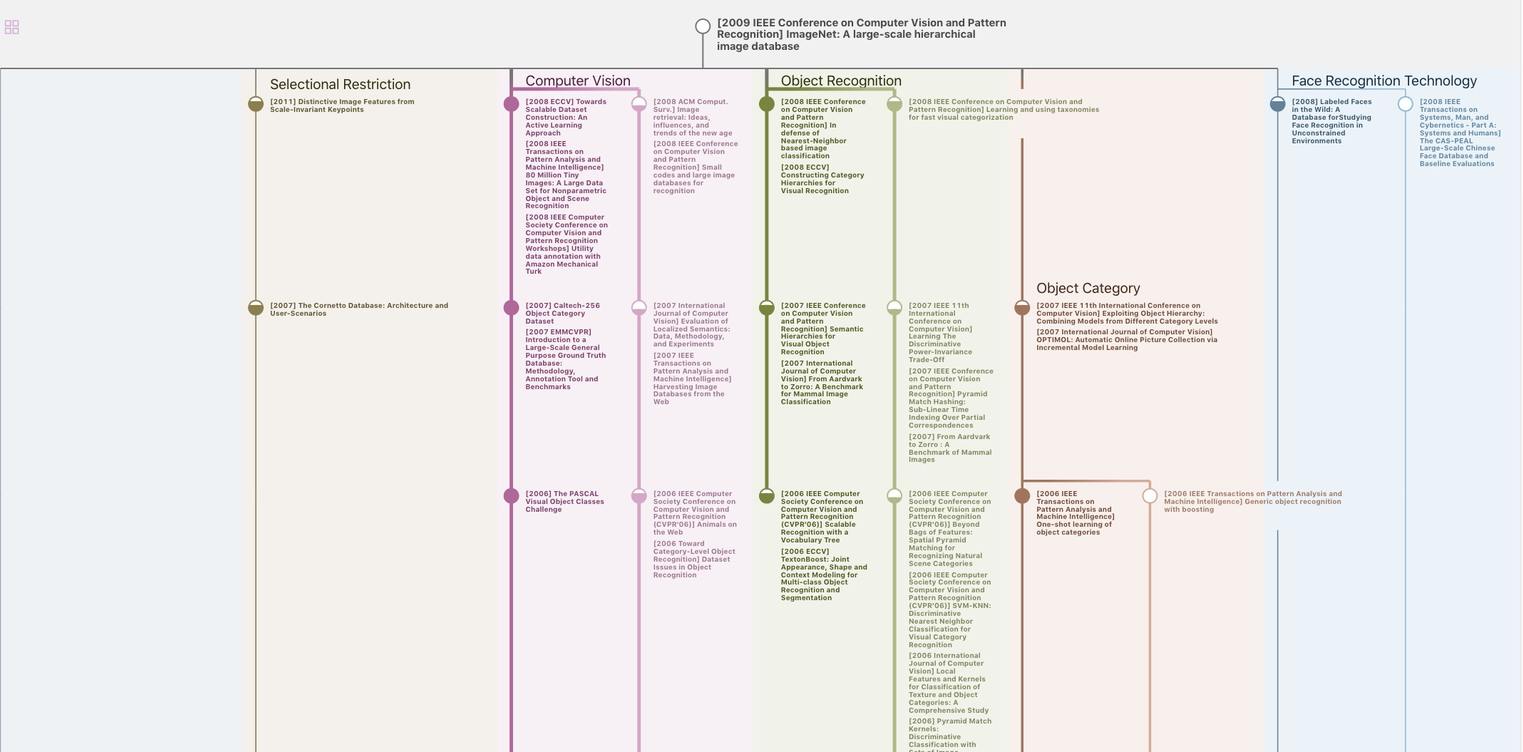
生成溯源树,研究论文发展脉络
Chat Paper
正在生成论文摘要