Small Target Augmentation for Urban Remote Sensing Image Real-Time Segmentation
IEEE TRANSACTIONS ON INTELLIGENT TRANSPORTATION SYSTEMS(2023)
摘要
Urban remote sensing (URS) image segmentation is very important for many applications from automotive navigation to infrastructure monitoring, and urban management. There are numerous small targets in URS image due to a large shooting field of view. However, the existing learning-based real-time image segmentation methods are not strong enough to handle small target and edge segmentation problems well, resulting in small targets that are easy to be missed, and blurred target edges. To segment the small targets and edges more accurately in real time, we propose a fast URS image segmentation method based on a multi-layer pixel attention mechanism (MPAM). We improve the performance and efficiency of the URS image segmentation from two perspectives: model and data. Specifically, to enhance semantic detailed information such as small targets and edges, we design mask-guided edge and small target feature enhancement modules in the real-time segmentation network. In addition, we propose a small target data enhancement method which uses an interpolation algorithm to amplify small targets in URS images, in order to improve the efficiency of existing URS data. The experimental results on Vaihingen, Potsdam, and DLRSD datasets show that the segmentation accuracy of our method reaches 86.74% mIoU, which is better than the state-of-the-art algorithms STDC, CFNet, and UNetFormer.
更多查看译文
关键词
URS image segmentation,small target,data augmentation,edge
AI 理解论文
溯源树
样例
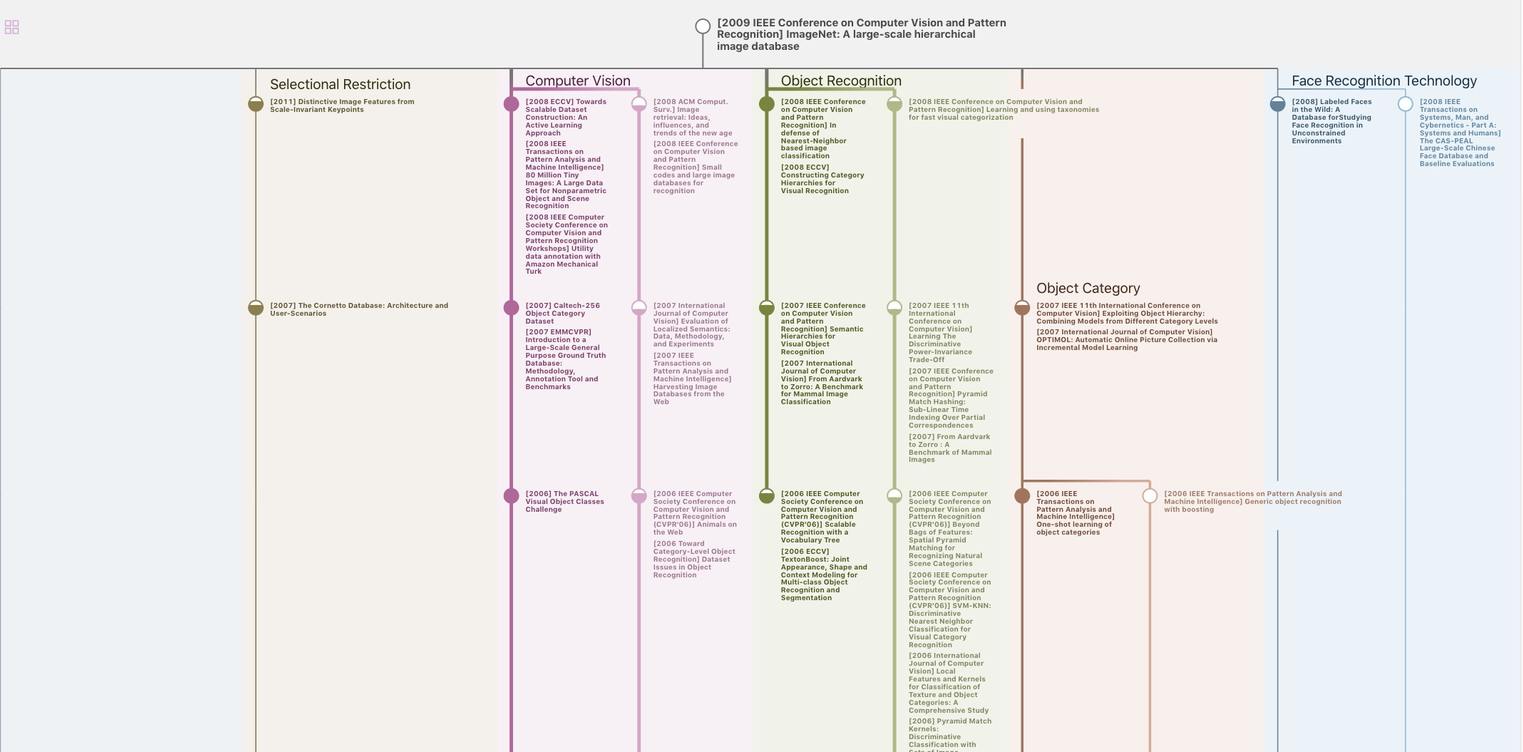
生成溯源树,研究论文发展脉络
Chat Paper
正在生成论文摘要