Quantitative Identification of Driver Distraction: A Weakly Supervised Contrastive Learning Approach
IEEE TRANSACTIONS ON INTELLIGENT TRANSPORTATION SYSTEMS(2024)
关键词
Behavioral sciences,Vehicles,Feature extraction,Transformers,Training,Decoding,Support vector machines,Driver distraction quantification,weakly supervised contrastive learning,representation clustering
AI 理解论文
溯源树
样例
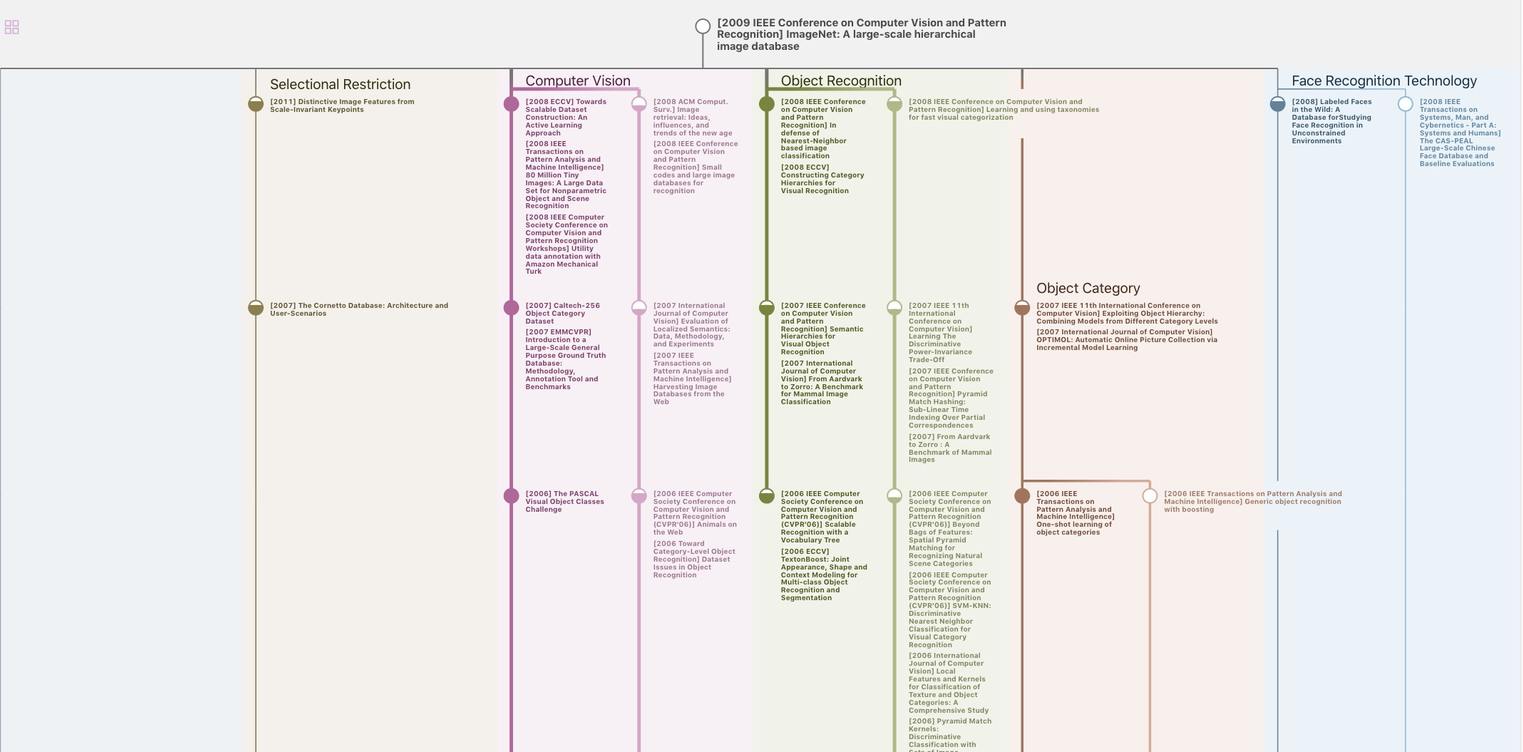
生成溯源树,研究论文发展脉络
Chat Paper
正在生成论文摘要